A Neural-Symbolic Model for Fan Interpretable Fault Diagnosis On Steel Production Lines
IEEE Internet of Things Journal(2024)
摘要
During the age of the Industrial Internet of Things (IIoT), extensive sensors are deployed on steel production lines to construct intelligent monitoring systems. A fan is a crucial piece of machinery in steel production lines, making its fault diagnosis imperative to prevent air pollution and casualties. DNN (Deep Neural Network) with powerful real-time IIoT data analysis has achieved outstanding performance in recognizing faults. Due to the black-box nature of DNNs, these models cannot provide reasonable explanations for their diagnostic decisions. It is still challenging for experts to make reliable and trustworthy conclusions. To address the issue, this paper introduces a new neural-symbolic model, termed Confidence and Classification DBN (CC-DBN), where confidence and classification rules are extracted from a Deep Belief Network (DBN) to provide an explainable representation of DBN feature learning and reasoning. In order to extract confidence rules, this paper develops a new clustering logic Restricted Boltzmann Machine (C-LRBM). Confidence rules can generate latent features of the raw vibration data of the fan and simultaneously explain the hierarchical reasoning of stacked RBM. Besides, to make trustworthy fan diagnosis decisions, classification rules are extracted to provide an explainable symbolic representation between input and output feature spaces. The experiment is performed on an industrial fan dataset from a leading steel production line in Shanghai. The results demonstrate that the proposed CC-DBN can effectively discover knowledge for fan diagnostic decisions and simultaneously achieve superior fault discrimination over typical classifiers and DBNs.
更多查看译文
关键词
Fan fault diagnosis,Deep belief network,Knowledge discovery,Neural-symbolic integration
AI 理解论文
溯源树
样例
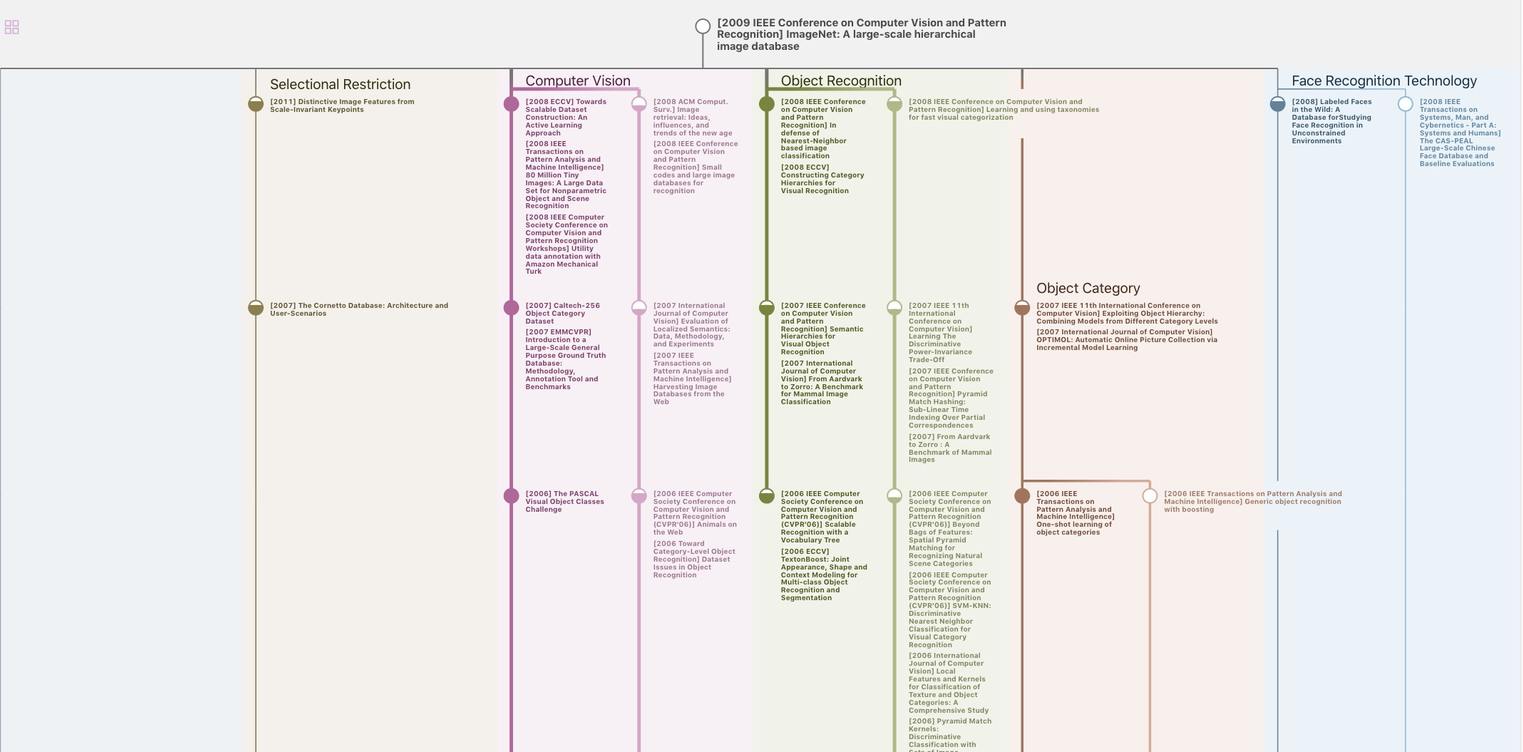
生成溯源树,研究论文发展脉络
Chat Paper
正在生成论文摘要