Deep Learning Methods for IoT Device Authentication Using Symbols Density Trace Plot.
IEEE Internet Things J.(2024)
摘要
Transmitter authentication is critical for secured Internet of Things (IoT) applications. Recently, there has been growing interest in utilizing the physical layer authentication technique, radio frequency (RF) fingerprinting, to introduce extra security measurements without adding additional components. This work presents a novel fingerprint exploitation modality, Density Trace Plot (DTP), to leverage RF fingerprints originating from symbol transition trajectories for transmitter authentication. With a particular focus on IQ imbalance as the source impairment for RF fingerprints, we investigate the feasibility of three types of DTPs based on constellation, eye, and phase traces. The potential fingerprints presented in the DTP modalities are then used in training three deep learning classifiers: 2D-convolutional neural network (CNN), 2D-CNN+bi-directional long short-term memory (biLSTM), and 3D-CNN for transmitter authentication. The feasibility of the proposed approach in both wired and wireless conditions is validated using an experimental setup built using ADALM-PLUTO software-defined radios (SDRs). Experimental results demonstrate the best authentication accuracy of 96.7% is achieved across signals of various modulation complexities.
更多查看译文
关键词
physical layer security,device authentication,RF fingerprinting,IQ imbalance,deep learning
AI 理解论文
溯源树
样例
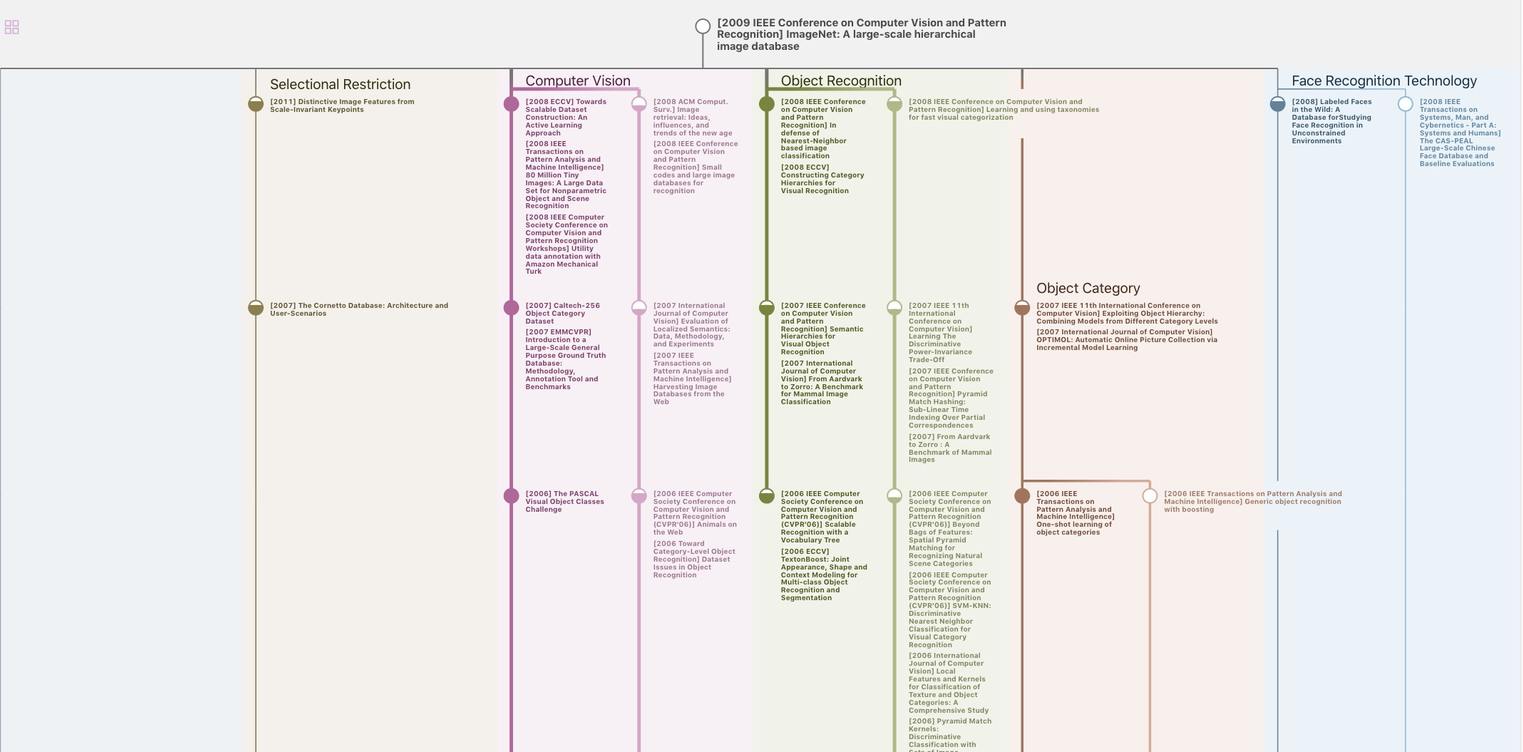
生成溯源树,研究论文发展脉络
Chat Paper
正在生成论文摘要