Are You Diligent, Inefficient, or Malicious? A Self-Safeguarding Incentive Mechanism for Large Scale-Federated Industrial Maintenance Based On Double Layer Reinforcement Learning
IEEE Internet of Things Journal(2024)
摘要
Fault prediction is an important application in the Industrial Internet of Things (IIoT) to ensure the safety of industrial systems and factories. Currently, deep learning-based fault prediction models are more popular, and multi-factory co-operation is required to improve the accuracy and generality of fault prediction models. Federated learning can coordinate multiple clients to train models together while protecting client privacy, and thus is widely used for training fault prediction models. How to incentivise more factories to participate in model training is crucial, however, most of the existing incentive mechanisms focus on the problem of fair measurement of client contributions and ignore the problem of incentive allocation in scenarios with limited incentive budgets. In this paper, we design a self-safeguarding incentive mechanism for large scale-federated industrial maintenance based on double layer reinforcement learning, known as Dual Layer Incentive (DLI). The method enables the central server to achieve higher model training accuracy within a limited incentive budget through rational allocation of incentives, which ultimately reduces the overall cost of model training. In addition, we categorise participating clients into “diligent clients”“, inefficient clients” and “malicious clients” based on their contributions and design tailor-made incentives for each client type, which saves training costs and enhances the safety of the model training process. Finally, the proposed approach is evaluated through experiments using various datasets. The results show that the method significantly improves the accuracy and safety of industrial fault prediction model compared to other existing methods.
更多查看译文
关键词
Fault Prediction,Industrial Internet of things,Federated Learning,Incentive Mechanism,Deep Reinforcement Learning
AI 理解论文
溯源树
样例
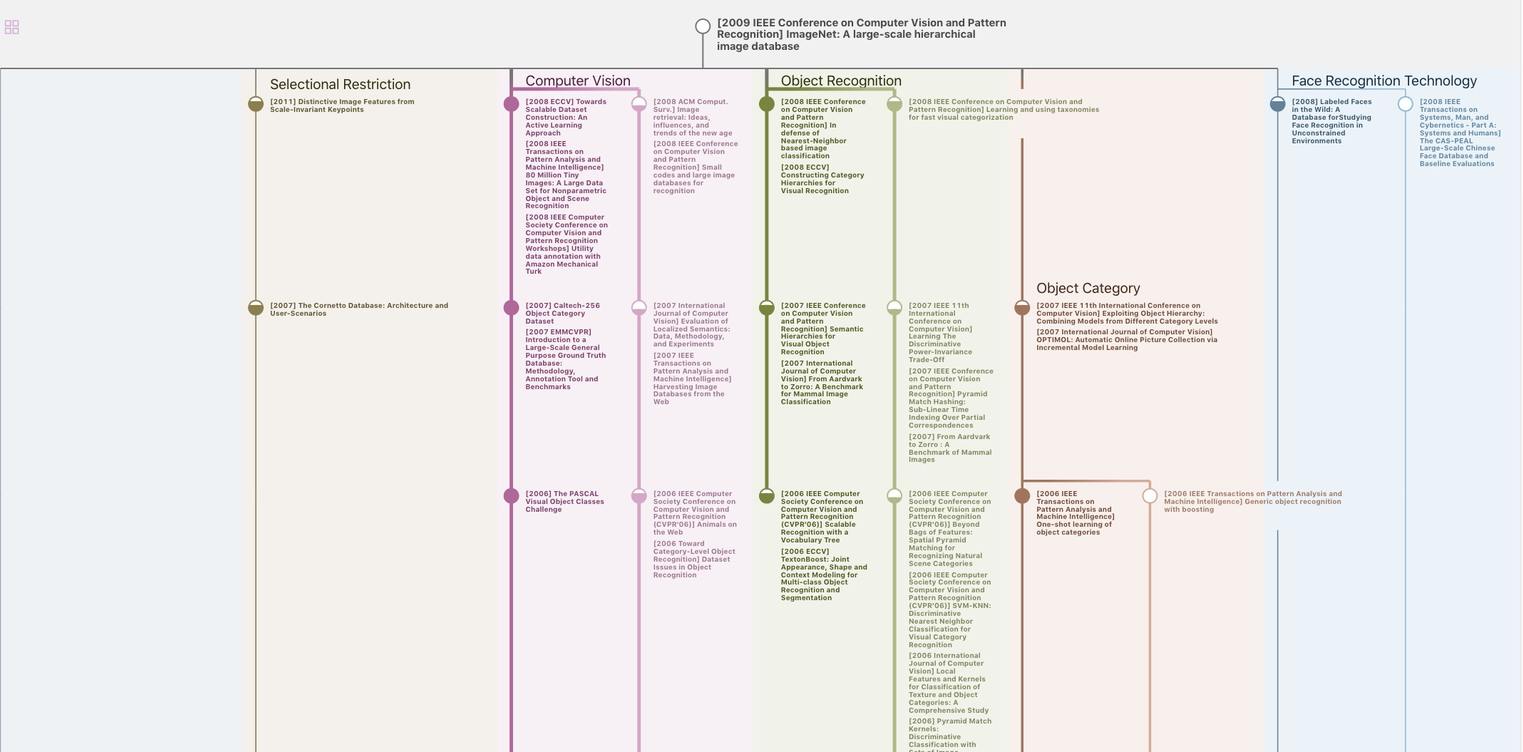
生成溯源树,研究论文发展脉络
Chat Paper
正在生成论文摘要