Deep Blind Image Quality Assessment Using Dynamic Neural Model with Dual-order Statistics
IEEE Transactions on Circuits and Systems for Video Technology(2024)
摘要
Deep convolutional neural networks (CNNs) have increasingly become a prominent method for blind image quality assessment (BIQA). The process of quality assessment typically involves feature extraction, average-based pooling, and quality regression. Based on this process, as well as the consensus that the visual quality of an image mainly relies on its content and distortions, this work improves CNNs for BIQA in two ways. First, considering the content-awareness of visual quality perception, we incorporate content-awareness via a dynamic filtering module to extract content-adaptive features and a dynamic regression module to learn content-adaptive perception rules based on local content and global semantics. Second, considering distortion-sensitivity in visual quality perception, we introduce second-order global variance pooling and combine it with global average pooling (GAP). First-order pooling methods like GAP are limited in distinguishing complex distortions that cause local degradation while preserving global features. Thus, pooling with dual-order statistics enables a more distortion-sensitive and discriminative global representation. These two improvements result in a content-adaptive BIQA model with a dual-order global pooling mechanism, improving generalization on diverse images with varying contents and distortion types. Extensive experiments on synthetic and authentic distortion datasets demonstrate state-of-the-art performance of the proposed approach.
更多查看译文
关键词
Blind Image Quality Assessment,Convolutional Networks,Dynamic Filtering,Feature Pooling,Dynamic Regression
AI 理解论文
溯源树
样例
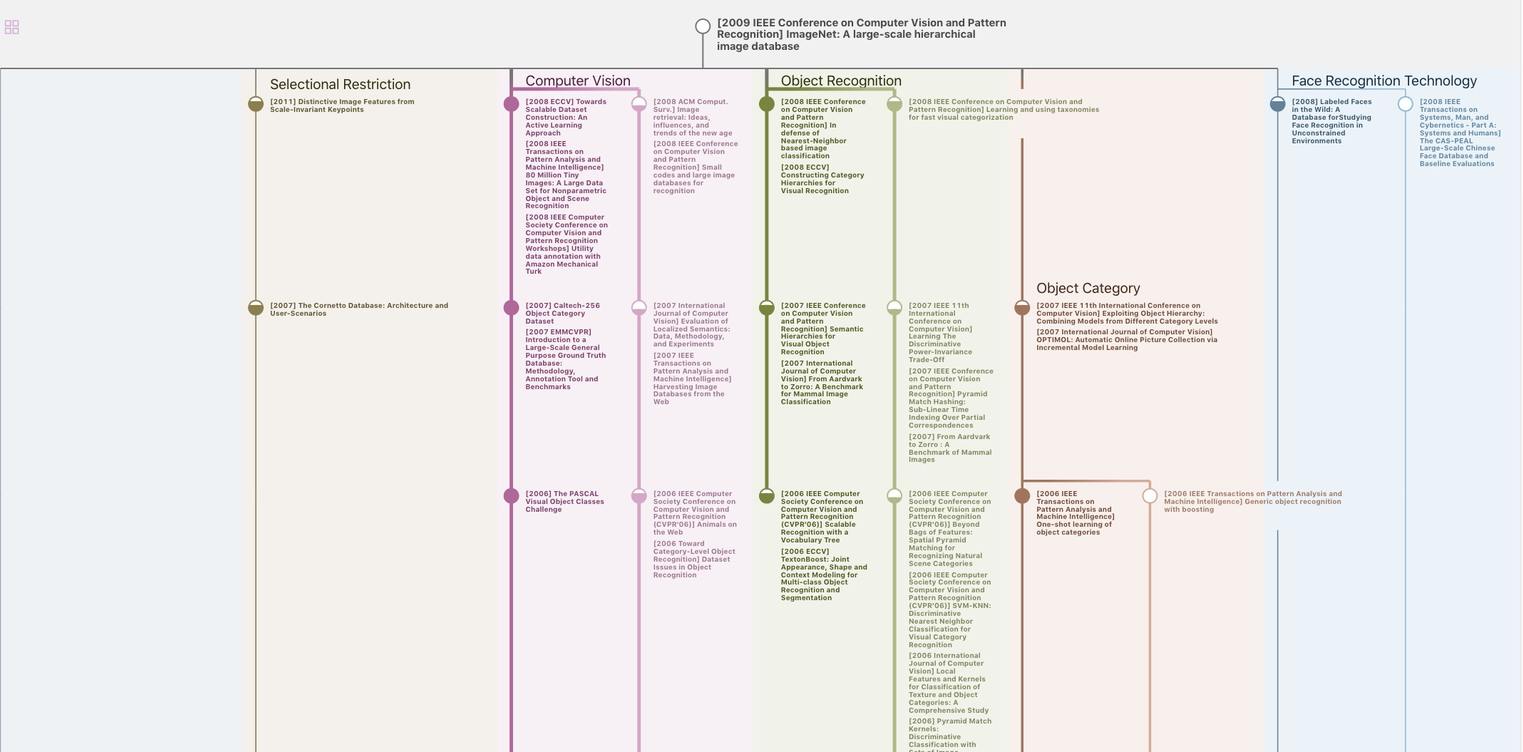
生成溯源树,研究论文发展脉络
Chat Paper
正在生成论文摘要