Cloth-Imbalanced Gait Recognition via Hallucination
IEEE Transactions on Circuits and Systems for Video Technology(2024)
摘要
The study in the gait field has rarely paid attention to the class-imbalanced learning, while the realistic data always exhibits an imbalanced distribution. The main reason lies in the difficulty of collecting the cross-clothes sequences, since the collection is usually aided by person re-identification and it is more likely to obtain the sequences for a subject wearing the same clothes. In this work, we formulate a new problem to tackle the task-specific cloth-imbalanced issue, dubbed as Cloth-Imbalanced Gait Recognition, and the training data consists of two parts denoted as head set and tail set. The sequences for a subject in head set cover the cross-clothes variation which is scarce in tail set to mimic the collection difficulty. Along with the problem formulation, we design a new method to deal with the inherent challenges, called Cross-Clothes Hallucination or CCH for short. Our method is inspired by the observation that certain directions in deep feature space correspond to meaningful semantic transformations, and it tries to generate the cross-clothes sequences for tail set referring to the cloth-changing transformation in head set. To evaluate CCH, we build two cloth-imbalanced benchmarks based on the widely-used CASIA-B and Outdoor-Gait. Extensive experiments demonstrate that CCH brings significant improvements over the baselines.
更多查看译文
AI 理解论文
溯源树
样例
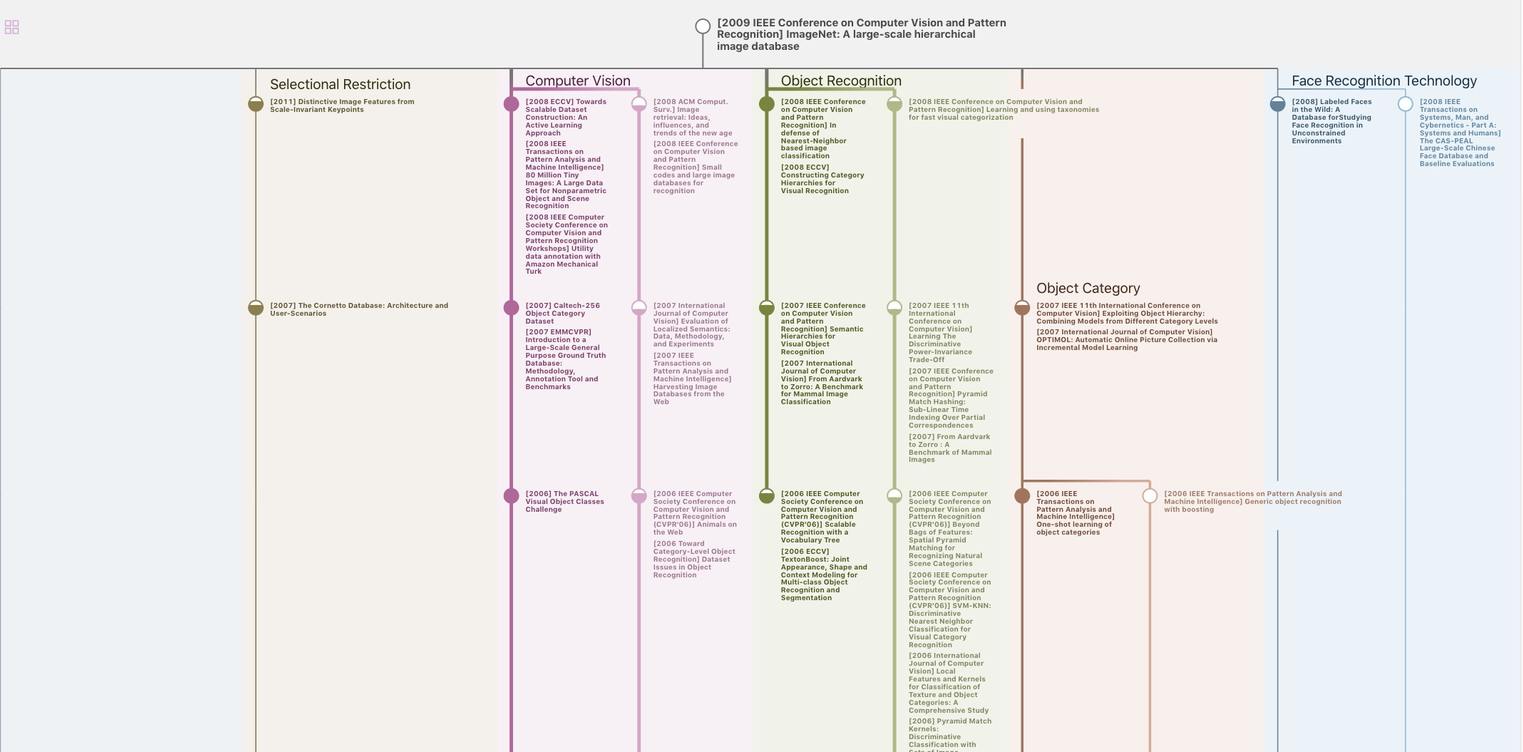
生成溯源树,研究论文发展脉络
Chat Paper
正在生成论文摘要