Enhanced Pseudo-Label Generation with Self-supervised Training for Weakly-supervised Semantic Segmentation
IEEE Transactions on Circuits and Systems for Video Technology(2024)
摘要
Due to the high cost of pixel-level labels required for fully-supervised semantic segmentation, weakly-supervised segmentation has emerged as a more viable option recently. Existing weakly-supervised methods tried to generate pseudo-labels without pixel-level labels for semantic segmentation, but a common problem is that the generated pseudo-labels contain insufficient semantic information, resulting in poor accuracy. To address this challenge, a novel method is proposed, which generates class activation/attention maps (CAMs) containing sufficient semantic information as pseudo-labels for the semantic segmentation training without pixel-level labels. In this method, the attention-transfer module is designed to preserve salient regions on CAMs while avoiding the suppression of inconspicuous regions of the targets, which results in the generation of pseudo-labels with sufficient semantic information. A pixel relevance focused-unfocused module has also been developed for better integrating contextual information, with both attention mechanisms employed to extract focused relevant pixels and multi-scale atrous convolution employed to expand receptive field for establishing distant pixel connections. The proposed method has been experimentally demonstrated to achieve competitive performance in weakly-supervised segmentation, and even outperforms many saliency-joined methods.
更多查看译文
关键词
Semantic segmentation,weakly-supervised learning,attention transfer mechanism,class attention/activation maps
AI 理解论文
溯源树
样例
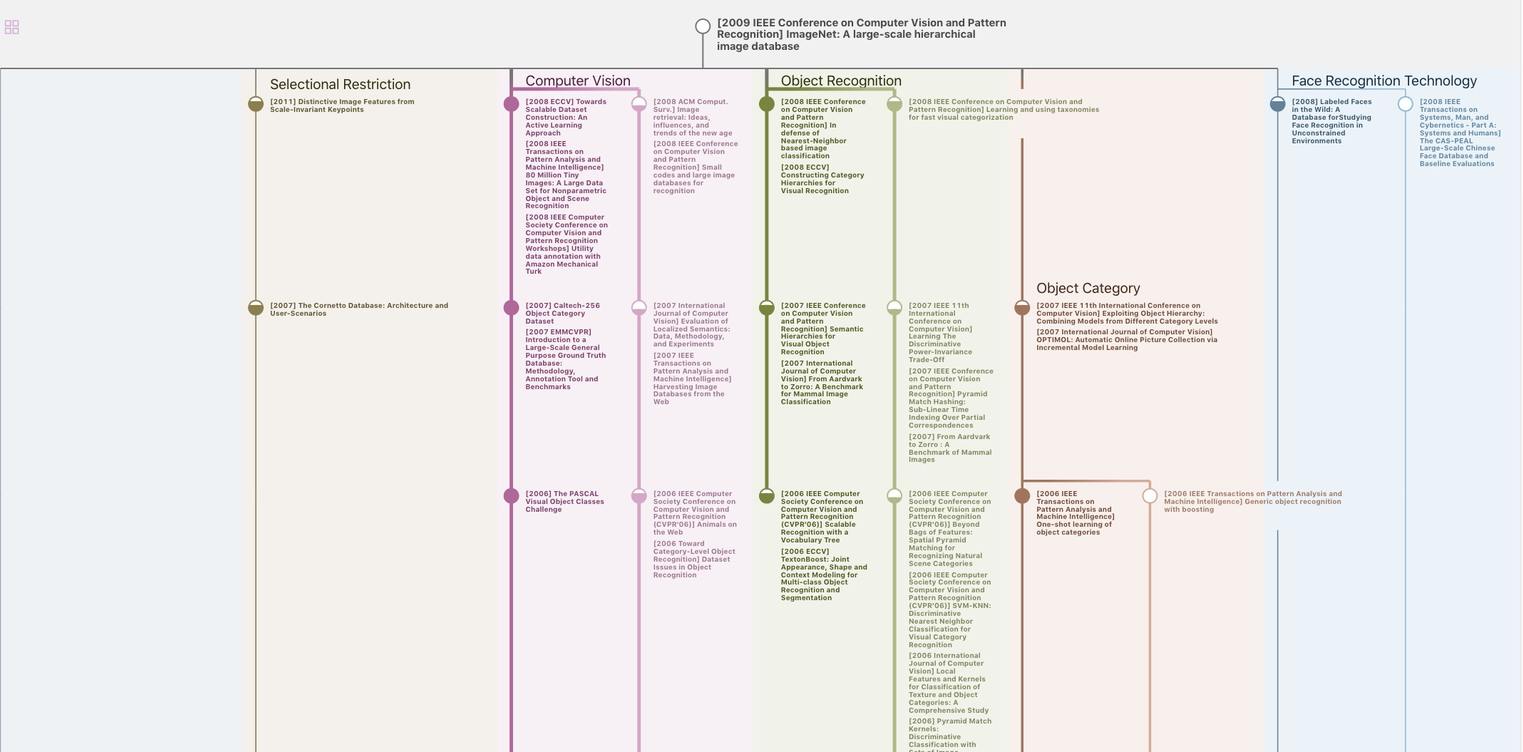
生成溯源树,研究论文发展脉络
Chat Paper
正在生成论文摘要