Rate-Distortion Optimized Cross Modal Compression with Multiple Domains
IEEE Transactions on Circuits and Systems for Video Technology(2024)
摘要
Cross-modal compression (CMC) aims to compress highly redundant visual data into compact, common, and human-comprehensible domains, such as text, to preserve semantic fidelity. However, CMC is limited by a constant level of semantic fidelity and constrained semantic fidelity due to a single compression domain (plain text). To address these issues, we propose a new approach called Multiple-domains rate-distortion optimized CMC (M-CMC). Specifically, our method divides the image into two complementary representations: (1) a structure representation with an edge map, and (2) a texture representation with dense captions, which include numerous region-caption pairs instead of plain text. In this way, we expand the single domain to multiple domains, namely, edge maps, regions, and text. To achieve diverse levels of semantic fidelity, we suggest a rate-distortion reward function, where the distortion measures the semantic fidelity of reconstructed images and the rate measures the information content of the text. We also propose Multiple-stage Self-Critical Sequence Training (MSCST) to optimize the reward function. Extensive experimental results demonstrate that the proposed method achieves diverse levels of semantic translation more effectively than other CMC-based methods, achieves higher semantic compression performance compared to traditional block-based and learning-based image compression frameworks with 97,000-500 times compression ratio, and provides a simple yet effective way for image editing.
更多查看译文
关键词
Cross Modal Compression,Rate-Distortion Optimization,Reinforcement Learning
AI 理解论文
溯源树
样例
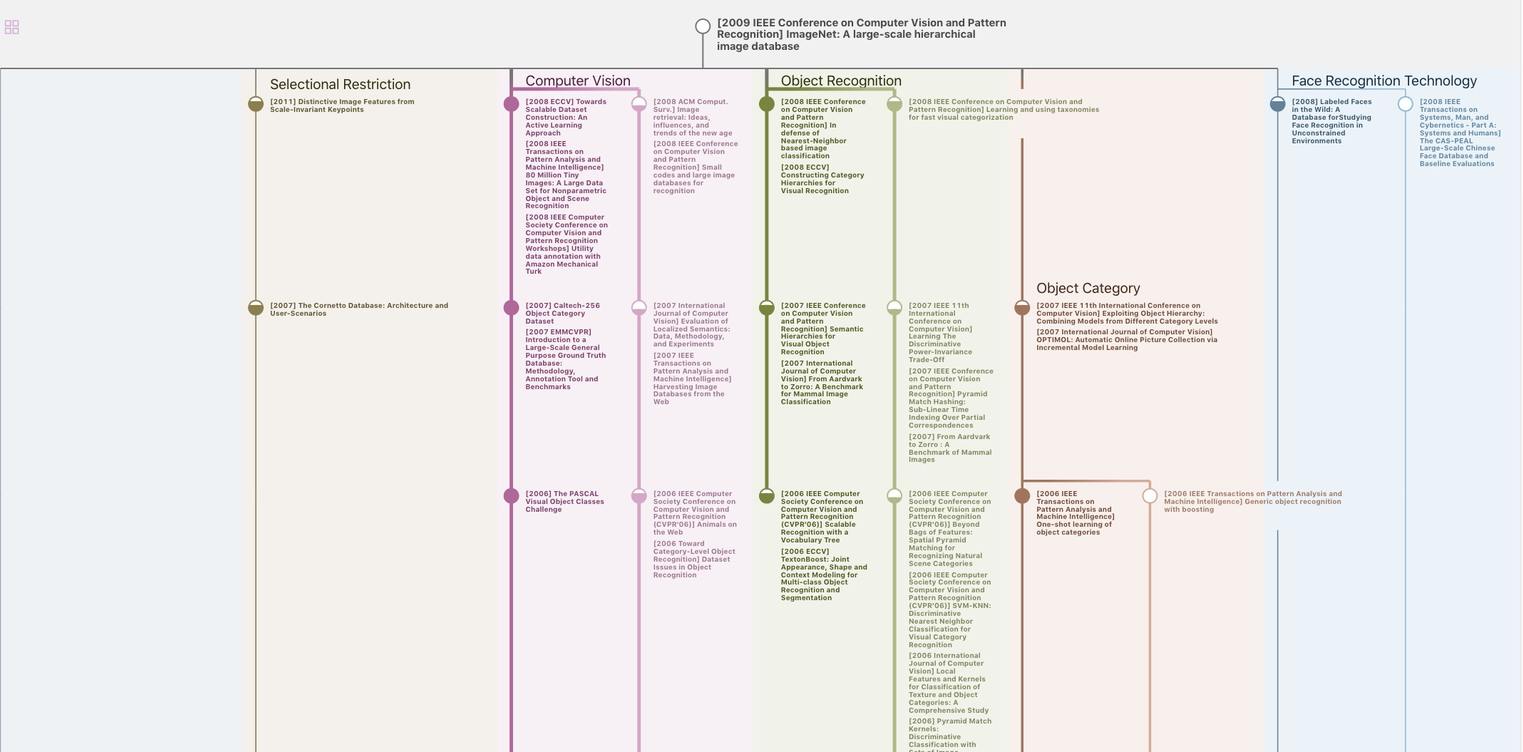
生成溯源树,研究论文发展脉络
Chat Paper
正在生成论文摘要