Efficient detectors for uplink massive MIMO systems
Journal of Communications and Networks(2024)
摘要
Massive multiple-input multiple-output (MIMO) is one of the essential technologies in beyond fifth generation (B5G) communication systems due to its impact in attaining high power efficiency and spectrum efficiency. The design of low-complexity detectors for massive MIMO continues to attract significant research and industry attention due to the critical need to find the right balance between performance and computational complexity, especially with a large number of antennas at both the transmitting and receiving sides. It has been noticed in several recent studies that appropriate initialization of iterative data detection techniques plays a crucial role in both the performance and the computational complexity. In this article, we propose three efficient initialization methods that achieve a favorable balance between performance and complexity. Instead of using the conventional diagonal matrix, we employ the scaled identity matrix, the stair matrix, and the band matrix with the first iteration of the Newton method to initialize the accelerated overrelaxation (AOR), the successive overrelaxation (SOR), the Gauss-Seidel (GS), the Jacobi (JA), and the Richardson (RI) based detectors. The scaling factor depends on the minimum and maximum eigenvalues of the equalization matrix. The proposed detectors are tested with different massive MIMO configurations, different modulation schemes (QPSK, 16QAM and 64QAM), and perfect and imperfect channel state information (CSI). Using simulations, we show that the proposed detectors achieve a significant performance gain compared to the minimum mean-squared error (MMSE) based detector, the conventional linear massive MIMO detectors, and other existing detectors, at a remarkable complexity reduction.
更多查看译文
关键词
Acceleration overrelaxation,B5G,Gauss-Seidel,Jacobi,massive MIMO,Newton iteration,Richardson,successive overrelaxation
AI 理解论文
溯源树
样例
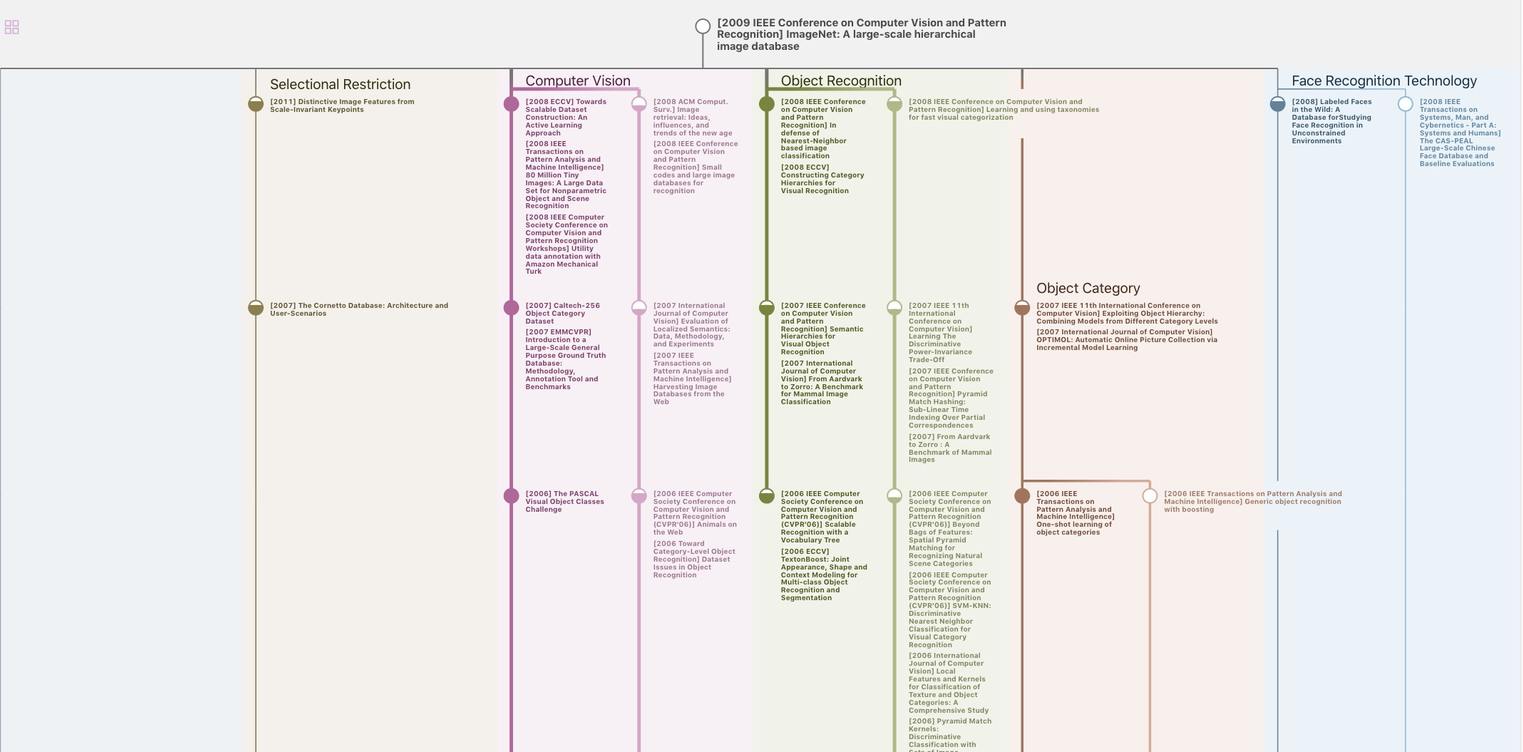
生成溯源树,研究论文发展脉络
Chat Paper
正在生成论文摘要