Fine-Grained Butterfly Classification Based on Multi-Feature Enhancement
IEEE ACCESS(2024)
摘要
Due to the challenges caused by the complexity and repetitiveness of discriminative features in fine-grained butterfly images, this paper proposes a fine-grained butterfly classification model based on multi-feature enhancement and multi-scale fusion to improve the accuracy of fine-grained butterfly classification. We use ResNet50 as the base network to extract features at different scales of the image. Next, the discriminative features are enhanced using spatial attention. The enhanced discriminative features are fed into the next stage of the base network, and the above operation is repeated to obtain the enhanced discriminative features at different scales. We then use the channel covariance attention module to eliminate the extra noise introduced during multi-feature enhancement. Finally, the final feature map is obtained by fusing the complementary information of different scale feature maps using the multi-scale fusion module. Ultimately, the method used in this paper achieved 96.896% accuracy on a dataset of 10 classes of fine-grained butterfly classification, and the comparison experiments proved that the method used in this paper outperforms the mainstream fine-grained visual classification methods.
更多查看译文
关键词
Butterfly classification,fine grained visual classification,multiscale fusion,multi feature enhancement
AI 理解论文
溯源树
样例
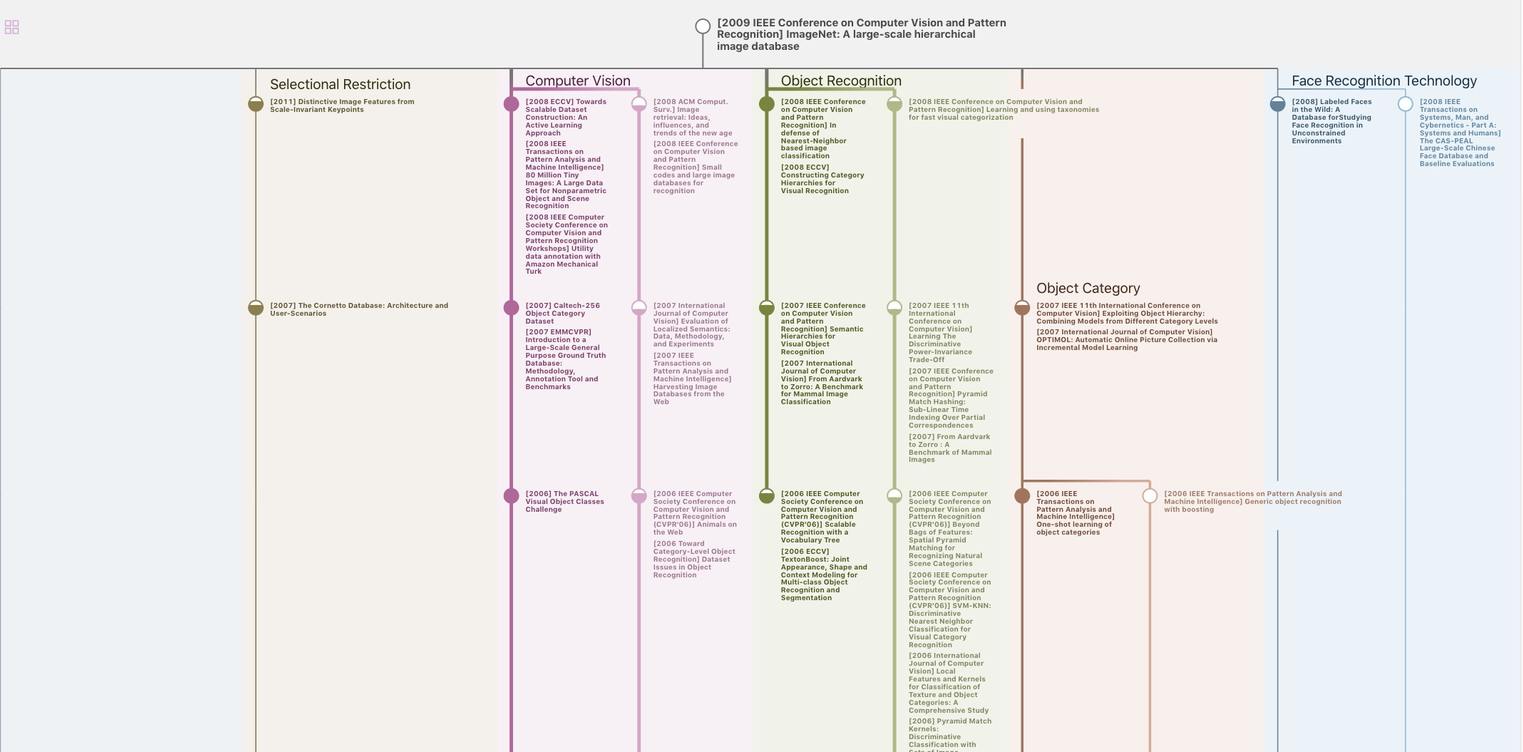
生成溯源树,研究论文发展脉络
Chat Paper
正在生成论文摘要