Augmentation of Fingerprints for Indoor BLE Localization Using Conditional GANs
IEEE ACCESS(2024)
摘要
Location estimation in indoor environments using radiofrequency (RF) has garnered considerable attention in recent years owing to the widespread adoption of mobile devices. RF-based fingerprinting-a direct approach that allows location estimation based on observed signals-relies on manual surveys during the offline phase to create a radio map with coordinates and RF measurements at multiple locations. The accuracy of RF fingerprint-based localization is proportional to the number of reference points. However, conventional site survey procedures incur substantial expenses. To alleviate the workload of site surveys and address the challenge of incomplete fingerprint databases, we propose a data-augmentation method to complement existing fingerprint data. Our approach leverages a conditional generative adversarial network with long short-term memory (CGAN-LSTM) prediction model to effectively learn the intricate patterns inherent in the initial training data and generate high-quality synthetic data that align with the underlying data distribution. In an experimental evaluation conducted on a real testbed, our data augmentation framework increased the average localization accuracy by 15.74% compared with fingerprinting without data augmentation. Compared with linear interpolation, inverse distance weighting, and Gaussian process regression, the proposed approach demonstrates an average accuracy improvement ranging from 1.84% to 14.04%, achieving average accuracies of 1.065 and 1.956 m in both scenarios. In experiments conducted in two typical indoor environments using sparse data, the proposed approach substantially reduced localization error and proved comparable to state-of-the-art data-augmentation methods.
更多查看译文
关键词
Fingerprint recognition,Location awareness,Radio frequency,Wireless fidelity,Costs,Databases,Surveys,Bluetooth Low Energy,Data augmentation,Generative adversarial networks,Indoor environment,Bluetooth low energy (BLE),fingerprint,data augmentation,generative adversarial network (GAN),location estimation
AI 理解论文
溯源树
样例
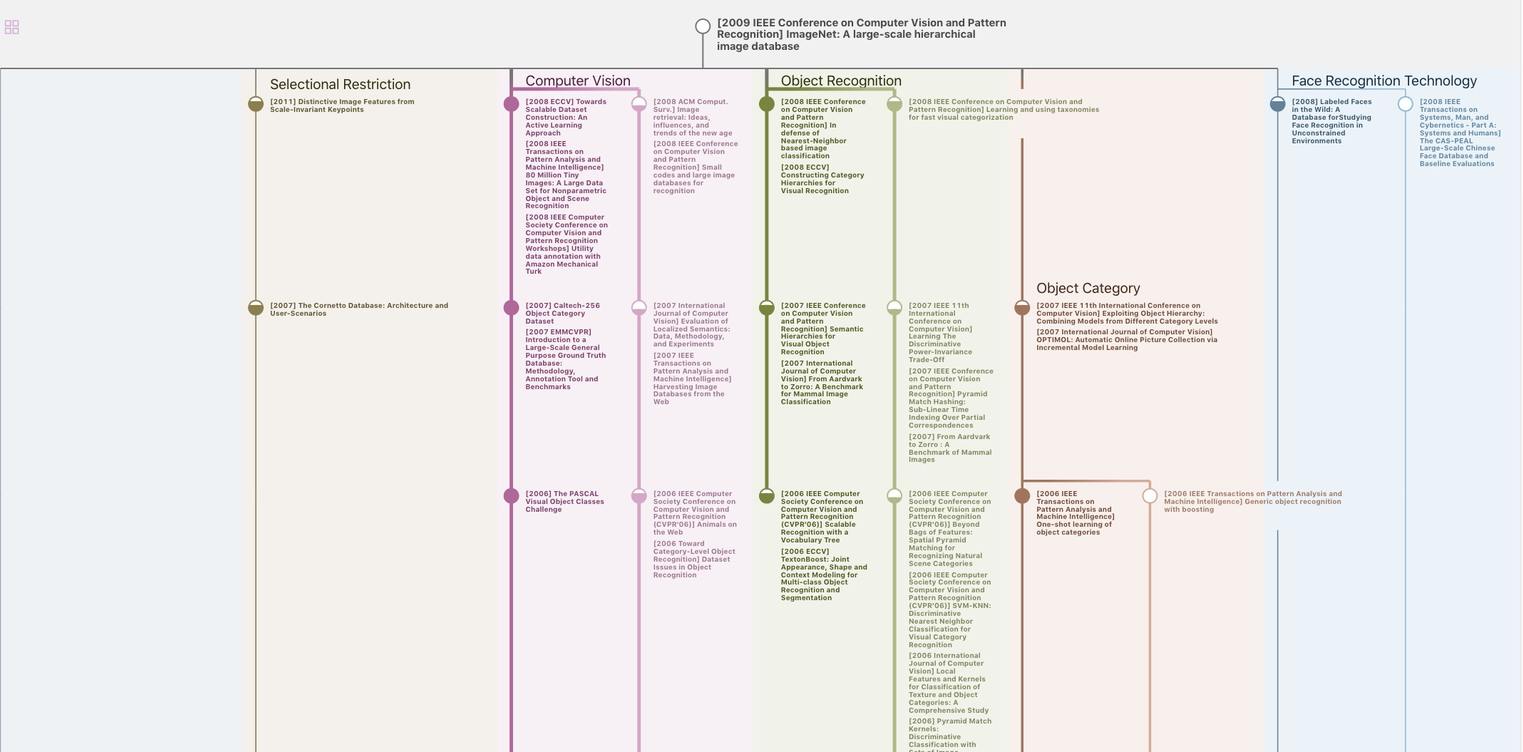
生成溯源树,研究论文发展脉络
Chat Paper
正在生成论文摘要