UJAT-Net: A U-Net Combined Joint-Attention and Transformer for Breast Tubule Segmentation in H&E Stained Images
IEEE ACCESS(2024)
摘要
The formation of breast tubules is an important evaluation index in the pathological grading of breast cancer. However, the tubules of breast present a wide variety of morphologies and a significant demonstrated significant advantages in the automatic analysis of histopathology images. We propose a Joint Attention and Transformer U-Net network to accurately segment breast tubules, named UJAT-Net. UJAT-Net uses the Joint Attention Block (named JA BLOCK) as the encoder of the network to enhance the extraction effect of the network for different layer features. And the Channel Cross fusion with Transformer (named CCT) module is used as the skip connection structure of the network. Furthermore, we employ a Transpose Cross Attention (named TCA) module as the decoder of the network to fuse the features of the skip connection layer and the decoder. Experimental results on our own invasive breast cancer tubule (Tubule of Breast Cancer, TBC) dataset and the benchmark dataset (Glas) of the GlaS challenge contest at MICCAI'2015 demonstrate that our method achieves competitive performance.
更多查看译文
关键词
Hematoxylin-eosin (H&E) stained images,tubule segmentation,U-Net,attention mechanism
AI 理解论文
溯源树
样例
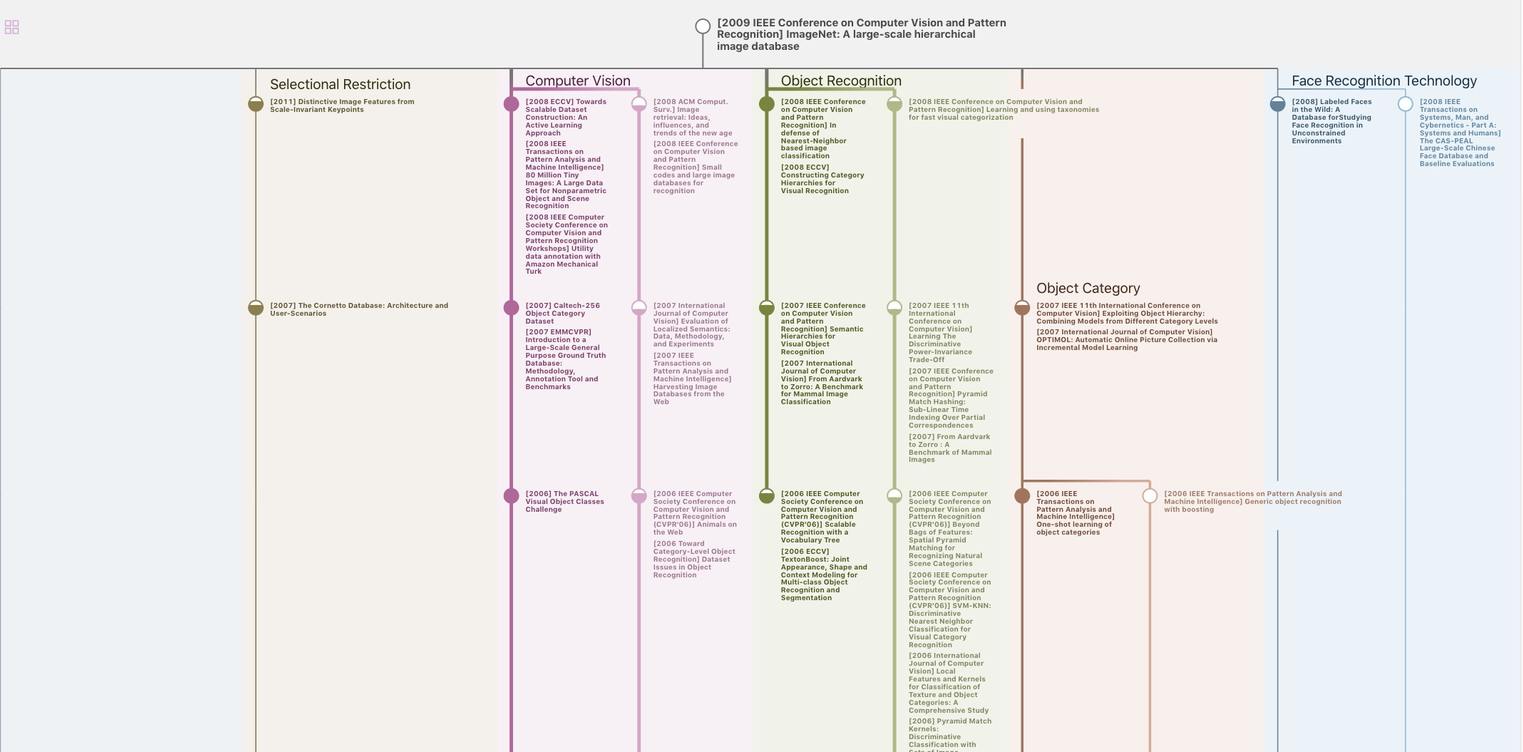
生成溯源树,研究论文发展脉络
Chat Paper
正在生成论文摘要