Deep Transfer Learning for Detecting Electric Vehicles Highly-Correlated Energy Consumption Parameters
IEEE Transactions on Artificial Intelligence(2024)
摘要
Implementation of advanced intelligent deep learning techniques for Electric Vehicles (EVs) energy consumption analysis is obstructed by two main subjects. First, the problem of finding a very similar collection of data sets to the actual EVs energy usage in terms of feature space and data distribution. Second, training a retrained model from scratch requires a massive amount of computational power, however, this does not guarantee to catch rare events included in data sets. To mitigate the aforementioned concerns, this paper aims to present a model based on Deep Transfer Learning (DTL) between domain-variant data sets, to reduce the need for the existence of a vast amount of EVs data, including driving characteristics and patterns. Also, this model applies a distributed cooperative learning approach to identify highly-correlated energy consumption parameters by building the model on previously acquired knowledge from preceding learning phases in order to enhance the Artificial Intelligence (AI) accuracy level of the proposed energy management system.
更多查看译文
关键词
EVs,AI in Transportation,Deep Learning,Transfer Learning,Neural Networks
AI 理解论文
溯源树
样例
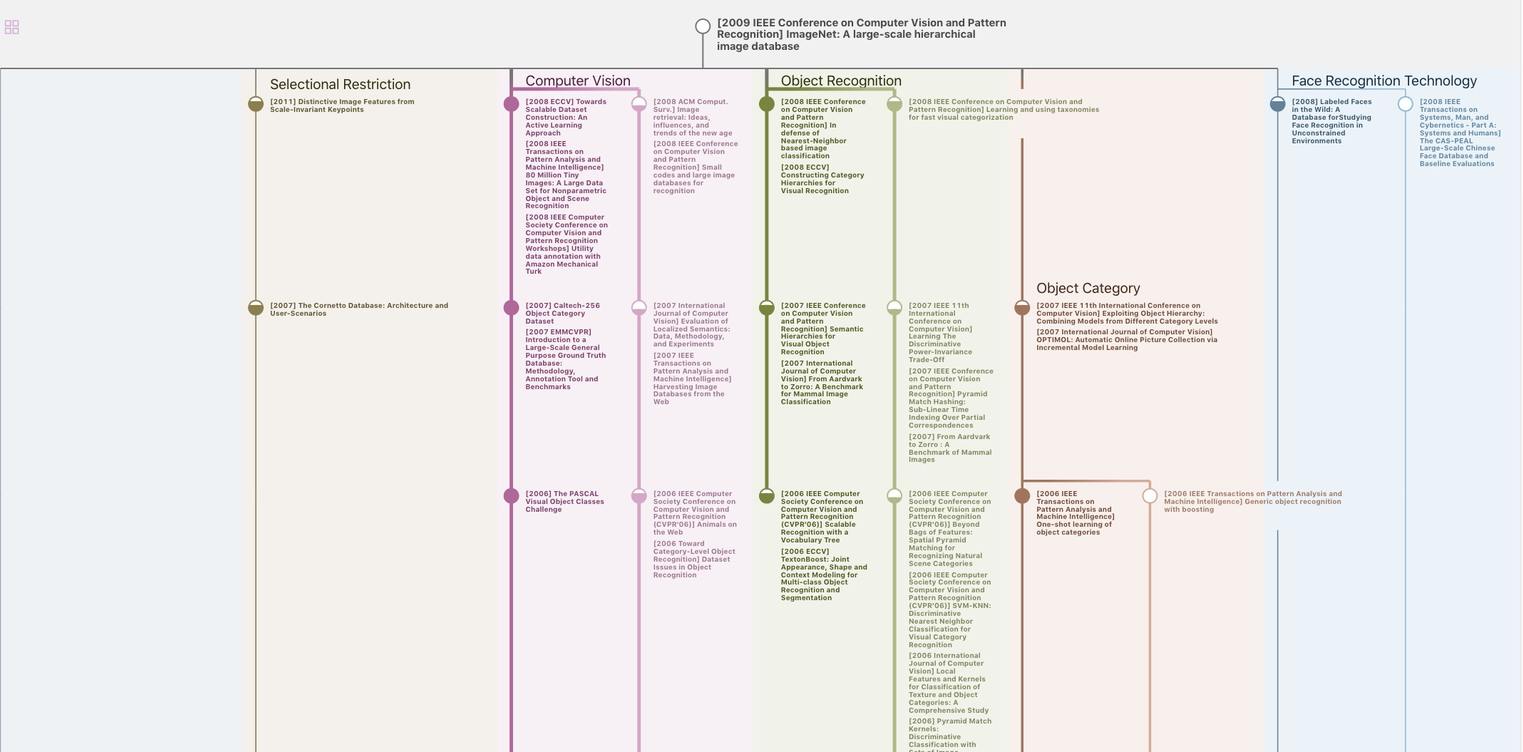
生成溯源树,研究论文发展脉络
Chat Paper
正在生成论文摘要