Sequence Labeling as Non-Autoregressive Dual-Query Set Generation.
IEEE ACM Trans. Audio Speech Lang. Process.(2024)
摘要
Sequence labeling is a crucial task in the NLP community that aims at identifying and assigning spans within the input sentence. It has wide applications in various fields such as information extraction, dialogue system, and sentiment analysis. However, previously proposed span-based or sequence-to-sequence models conduct locating and assigning in order, resulting in problems of error propagation and unnecessary training loss, respectively. This paper addresses the problem by reformulating the sequence labeling as a non-autoregressive set generation to realize locating and assigning in parallel. Herein, we propose a Dual-Query Set Generation (DQsetGen) model for unified sequence labeling tasks. Specifically, the dual-query set, including a prompted type query and a positional query with anchor span, is fed into the non-autoregressive decoder to probe the spans which correspond to the positional query and have similar patterns with the type query. By avoiding the autoregressive nature of previous approaches, our method significantly improves efficiency and reduces error propagation. Experimental results illustrate that our approach can obtain superior performance on 5 sub-tasks across 11 benchmark datasets. The non-autoregressive nature of our method allows for parallel computation, achieving faster inference speed than compared baselines. In conclusion, our proposed non-autoregressive dual-query set generation method offers a more efficient and accurate approach to sequence labeling tasks in NLP. Its advantages in terms of performance and efficiency make it a promising solution for various applications in data mining and other related fields.
更多查看译文
关键词
Sequence Labeling,Non-Autogressive,Set Generation,Transformer
AI 理解论文
溯源树
样例
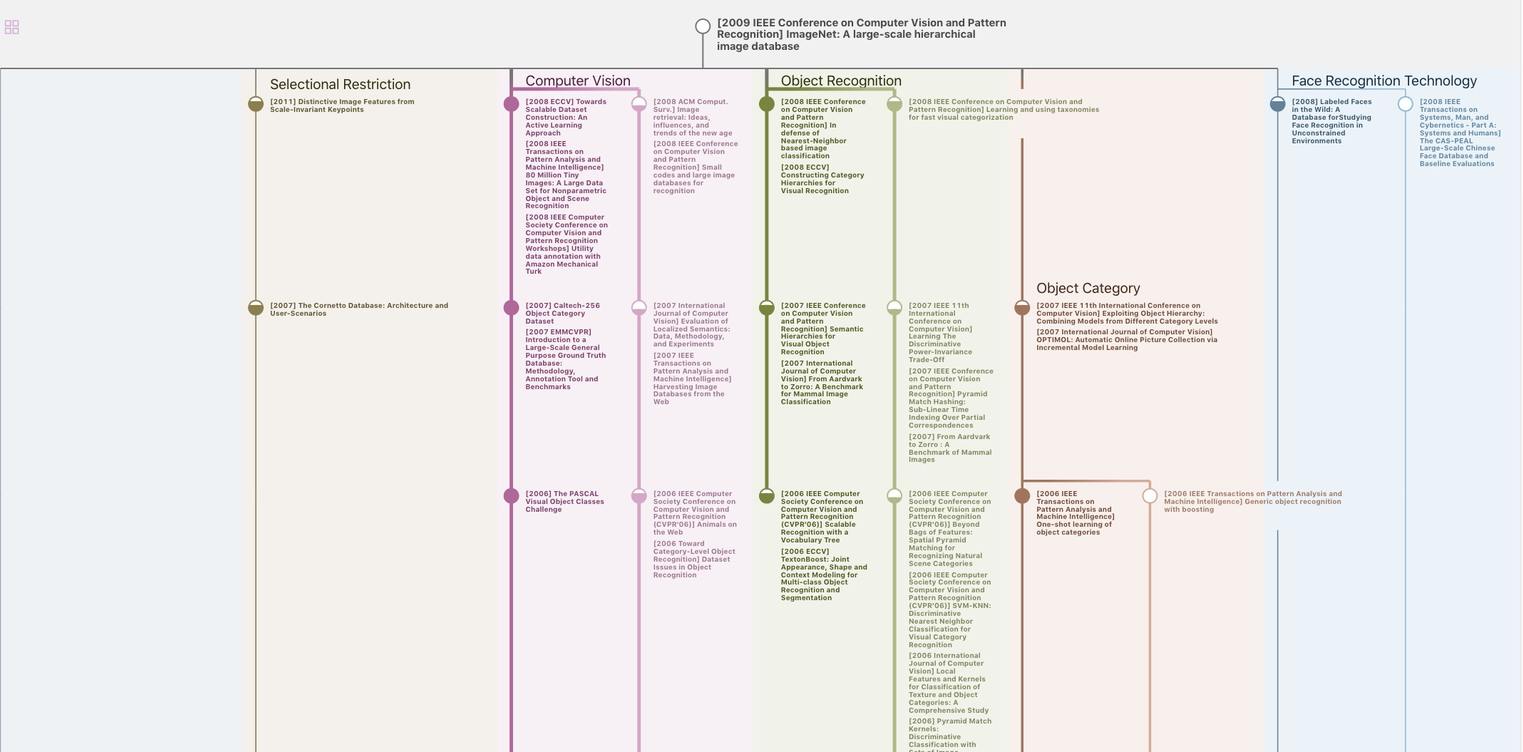
生成溯源树,研究论文发展脉络
Chat Paper
正在生成论文摘要