Looking into Gait for Perceiving Emotions via Bilateral Posture and Movement Graph Convolutional Networks
IEEE Transactions on Affective Computing(2024)
摘要
Emotions can be perceived from a person's gait, i.e., their walking style. Existing methods on gait emotion recognition mainly leverage the posture information as input, but ignore the body movement, which contains complementary information for recognizing emotions evoked in the gait. In this paper, we propose a Bilateral Posture and Movement Graph Convolutional Network (BPM-GCN) that consists of two parallel streams, namely posture stream and movement stream, to recognize emotions from two views. The posture stream aims to explicitly analyse the emotional state of the person. Specifically, we design a novel regression constraint based on the hand-engineered features to distill the prior affective knowledge into the network and boost the representation learning. The movement stream is designed to describe the intensity of the emotion, which is an implicitly cue for recognizing emotions. To achieve this goal, we employ a higher-order velocity-acceleration pair to construct graphs, in which the informative movement features are utilized. Besides, we design a PM-Interacted feature fusion mechanism to adaptively integrate the features from the two streams. Therefore, the two streams collaboratively contribute to the performance from two complementary views. Extensive experiments on the largest benchmark dataset Emotion-Gait show that BPM-GCN performs favorably against the state-of-the-art approaches (with at least
$4.59\%$
performance improvement). The source code is released on
https://github.com/exped1230/BPM-GCN
.
更多查看译文
关键词
Emotion identification,gait,bilateral posture and movement graph convolutional network,affective constraint
AI 理解论文
溯源树
样例
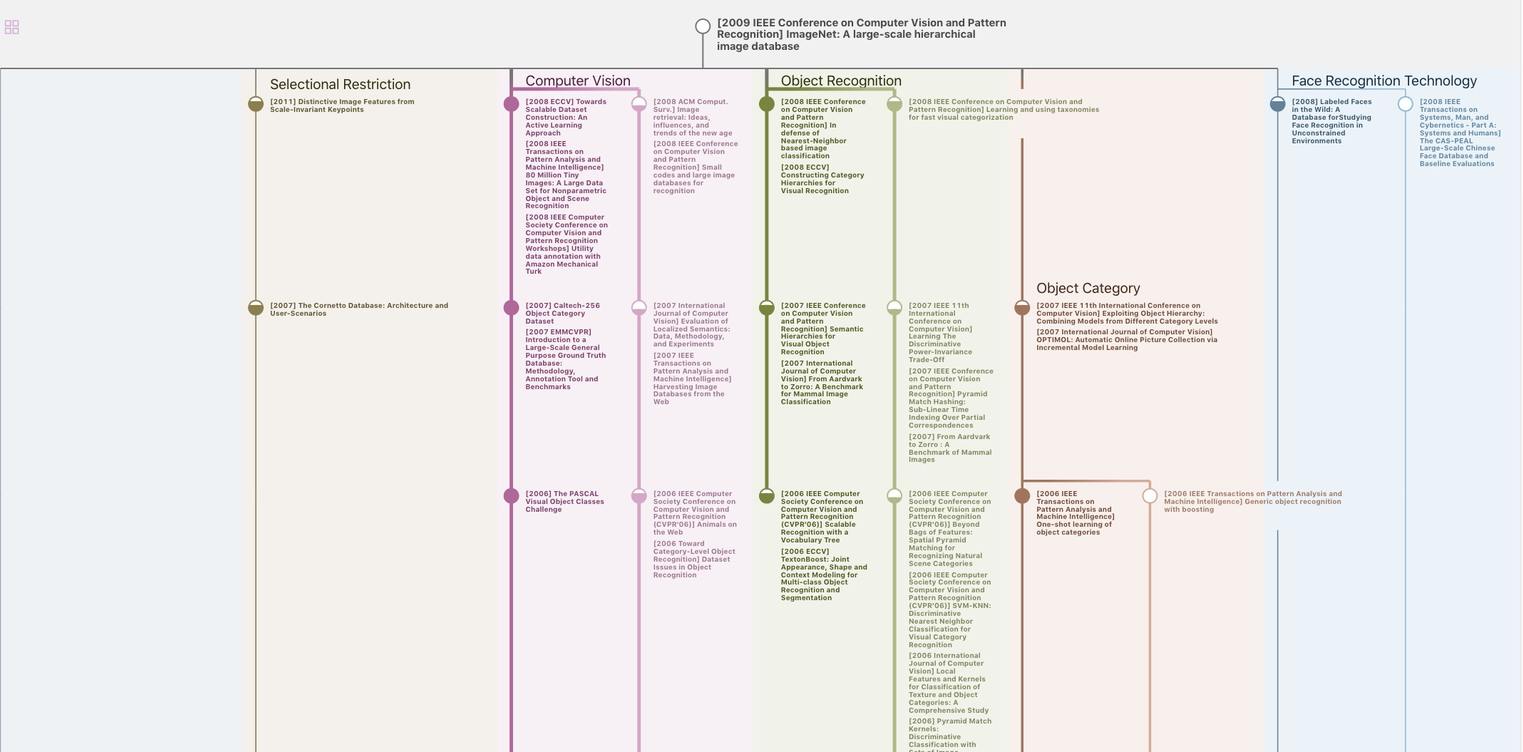
生成溯源树,研究论文发展脉络
Chat Paper
正在生成论文摘要