Model based Online Adaptive Inverse Non-Cooperative Linear-Quadratic Differential Games via Finite-Time Concurrent Learning
IEEE Transactions on Artificial Intelligence(2024)
摘要
Non-cooperative differential games provides a basis for the study of coordination, conflict, and control for a single dynamical system with multiple players. Within the linear quadratic differential games (LQDG), the optimal feedback gain matrix and the weighting matrices of individual cost function depict each player’s control policy and the tradeoff of various objectives, respectively. In this paper, we investigate the inverse problem of LQDG with partial state observation via a model based online method, i.e., recover the cost function of each player. First, a state observer is used to estimate the system state. Then, the feedback gain matrices of players are learned using a finite-time concurrent learning (FTCL) based adaptive law relaxing the persistent excitation (PE) condition which is required in traditional adaptive estimation methods. With the learned feedback gain matrices, a semidefinite programming (SDP) problem with the quadratic objective function can be set up for determining the weighting matrices of the cost function. The applicability and effectiveness of the proposed method are demonstrated with a numerical example and a shared steering control simulation.
更多查看译文
关键词
Linear quadratic differential games (LQDG),coupled algebraic Riccati equations (CAREs),finite-time concurrent learning (FTCL),semidefinite programming (SDP)
AI 理解论文
溯源树
样例
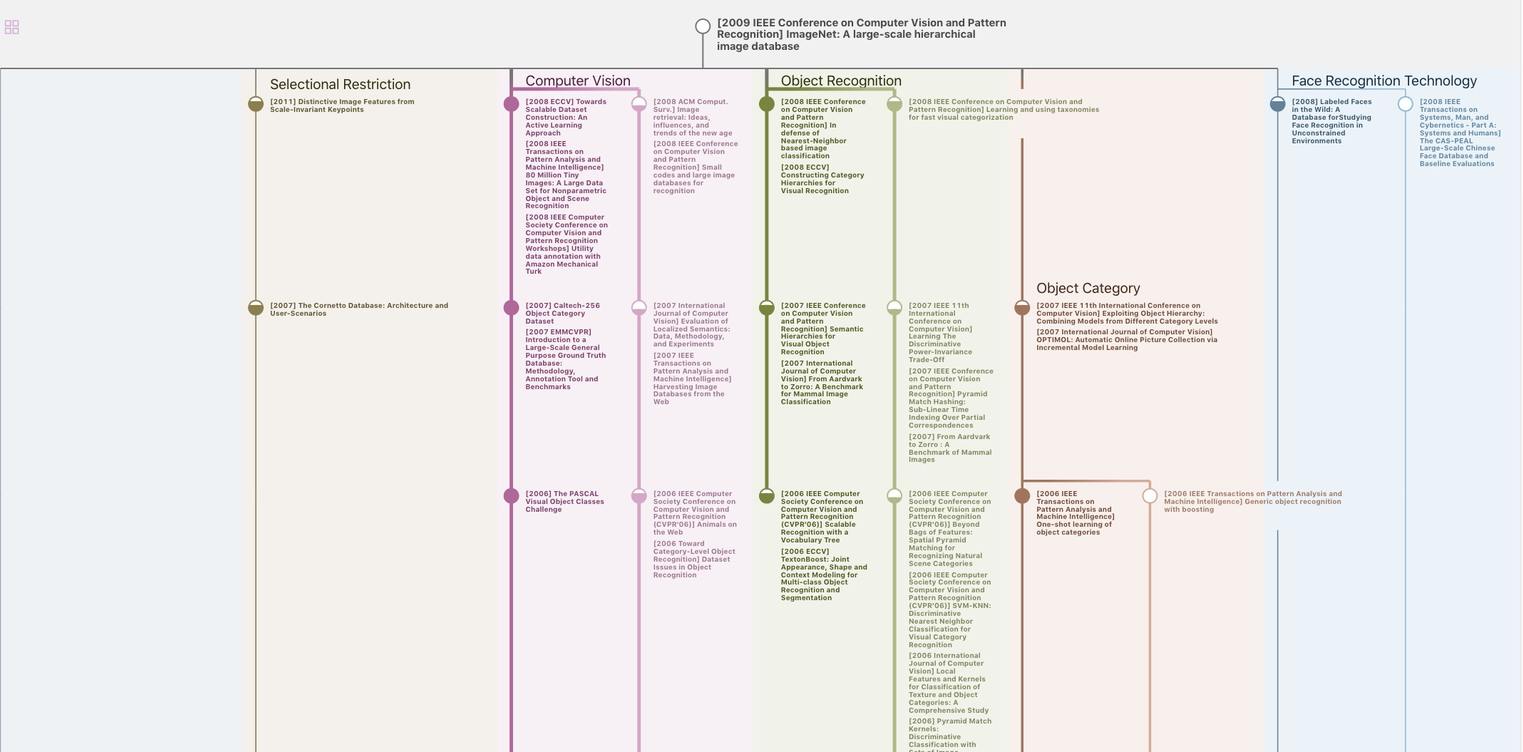
生成溯源树,研究论文发展脉络
Chat Paper
正在生成论文摘要