RBP-MTL: Agricultural Parcel Vectorization via Region-Boundary-Parcel Decoupled Multitask Learning
IEEE TRANSACTIONS ON GEOSCIENCE AND REMOTE SENSING(2024)
摘要
Agricultural parcel vectorization is important for precision agriculture when analyzing crops at the parcel scale. However, it is a challenging task to vectorize parcels from satellite imagery, where undersegmentation and the discontinuous boundaries of parcels are common problems, due to the varied shapes and sizes of parcels caused by the terrain and the farming mode, and the blurred boundaries caused by the limited resolution and the shadows. In this article, a region-boundary-parcel decoupled multitask learning (RBP-MTL) framework is proposed for agricultural parcel vectorization, where the local spatial constraints between the regions, boundaries, and the objects of each parcel are jointly modeled via multitask learning, to promote the object separability of agricultural parcels and the boundary connectivity. In addition, simple and efficient boundary-object interaction vectorization is introduced to further ensure that each parcel is independent with a complete and separable boundary. In the experiments, the proposed framework was verified using three datasets with different spatial resolutions, i.e., the public parcel extraction dataset from the iFLYTEK Challenge 2021 (similar to 1 m), in addition to a single-temporal Gaofen-1 dataset from Hubei province in China (2 m) and a multitemporal Sentinel-2 dataset from The Netherlands (10 m), which were annotated and built by the authors. The proposed RBP-MTL framework achieved a state-of-the-art accuracy, with effective extraction of complete parcel boundaries in the different scenes.
更多查看译文
关键词
Agricultural parcels,deep learning,multitask learning,vectorization
AI 理解论文
溯源树
样例
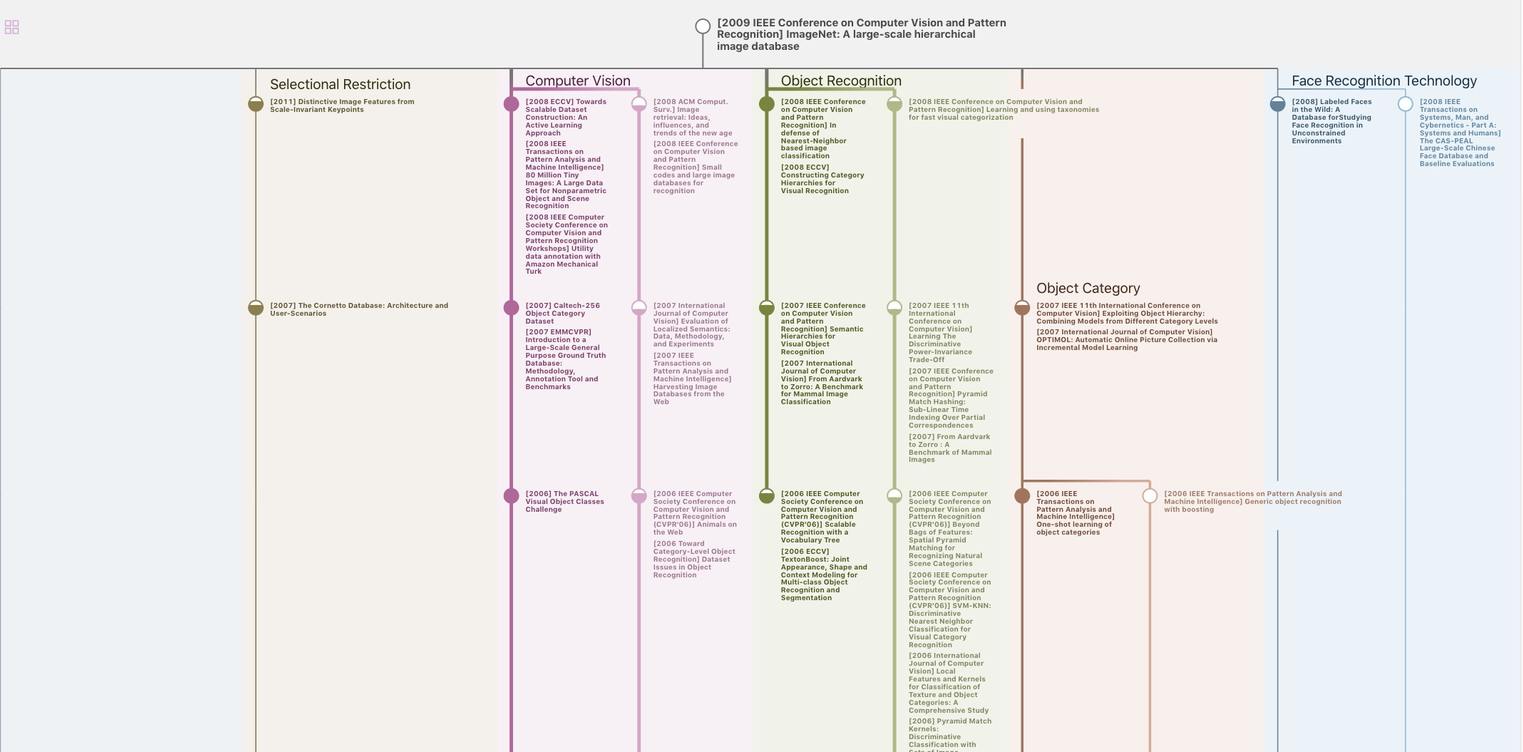
生成溯源树,研究论文发展脉络
Chat Paper
正在生成论文摘要