Using Text Embeddings for Deductive Qualitative Research at Scale in Physics Education
arxiv(2024)
摘要
We propose a technique for performing deductive qualitative data analysis at
scale on text-based data. Using a natural language processing technique known
as text embeddings, we create vector-based representations of texts in a
high-dimensional meaning space within which it is possible to quantify
differences as vector distances. To apply the technique, we build off prior
work that used topic modeling via Latent Dirichlet Allocation to thematically
analyze 18 years of the Physics Education Research Conference proceedings
literature. We first extend this analysis through 2023. Next, we create
embeddings of all texts and, using representative articles from the 10 topics
found by the LDA analysis, define centroids in the meaning space. We calculate
the distances between every article and centroid and use the inverted, scaled
distances between these centroids and articles to create an alternate topic
model. We benchmark this model against the LDA model results and show that this
embeddings model recovers most of the trends from that analysis. Finally, to
illustrate the versatility of the method we define 8 new topic centroids
derived from a review of the physics education research literature by Docktor
and Mestre (2014) and re-analyze the literature using these researcher-defined
topics. Based on these analyses, we critically discuss the features, uses, and
limitations of this method and argue that it holds promise for flexible
deductive qualitative analysis of a wide variety of text-based data that avoids
many of the drawbacks inherent to prior NLP methods.
更多查看译文
AI 理解论文
溯源树
样例
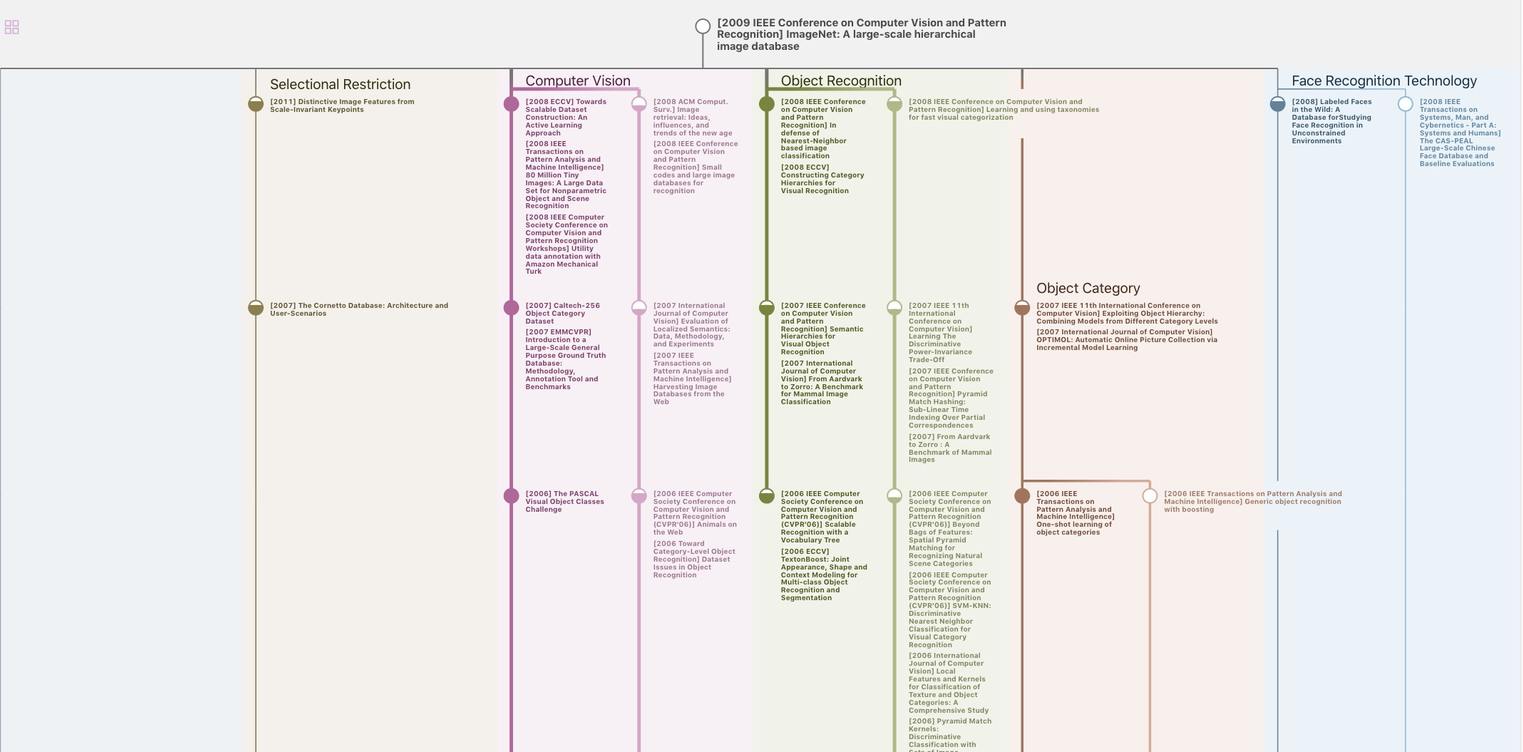
生成溯源树,研究论文发展脉络
Chat Paper
正在生成论文摘要