Benchmarking Large Language Models on Answering and Explaining Challenging Medical Questions
CoRR(2024)
摘要
LLMs have demonstrated impressive performance in answering medical questions,
such as passing medical licensing examinations. However, most existing
benchmarks rely on board exam questions or general medical questions, falling
short in capturing the complexity of realistic clinical cases. Moreover, the
lack of reference explanations for answers hampers the evaluation of model
explanations, which are crucial to supporting doctors in making complex medical
decisions. To address these challenges, we construct two new datasets: JAMA
Clinical Challenge and Medbullets. JAMA Clinical Challenge consists of
questions based on challenging clinical cases, while Medbullets comprises USMLE
Step 2 3 style clinical questions. Both datasets are structured as
multiple-choice question-answering tasks, where each question is accompanied by
an expert-written explanation. We evaluate four LLMs on the two datasets using
various prompts. Experiments demonstrate that our datasets are harder than
previous benchmarks. The inconsistency between automatic and human evaluations
of model-generated explanations highlights the need to develop new metrics to
support future research on explainable medical QA.
更多查看译文
AI 理解论文
溯源树
样例
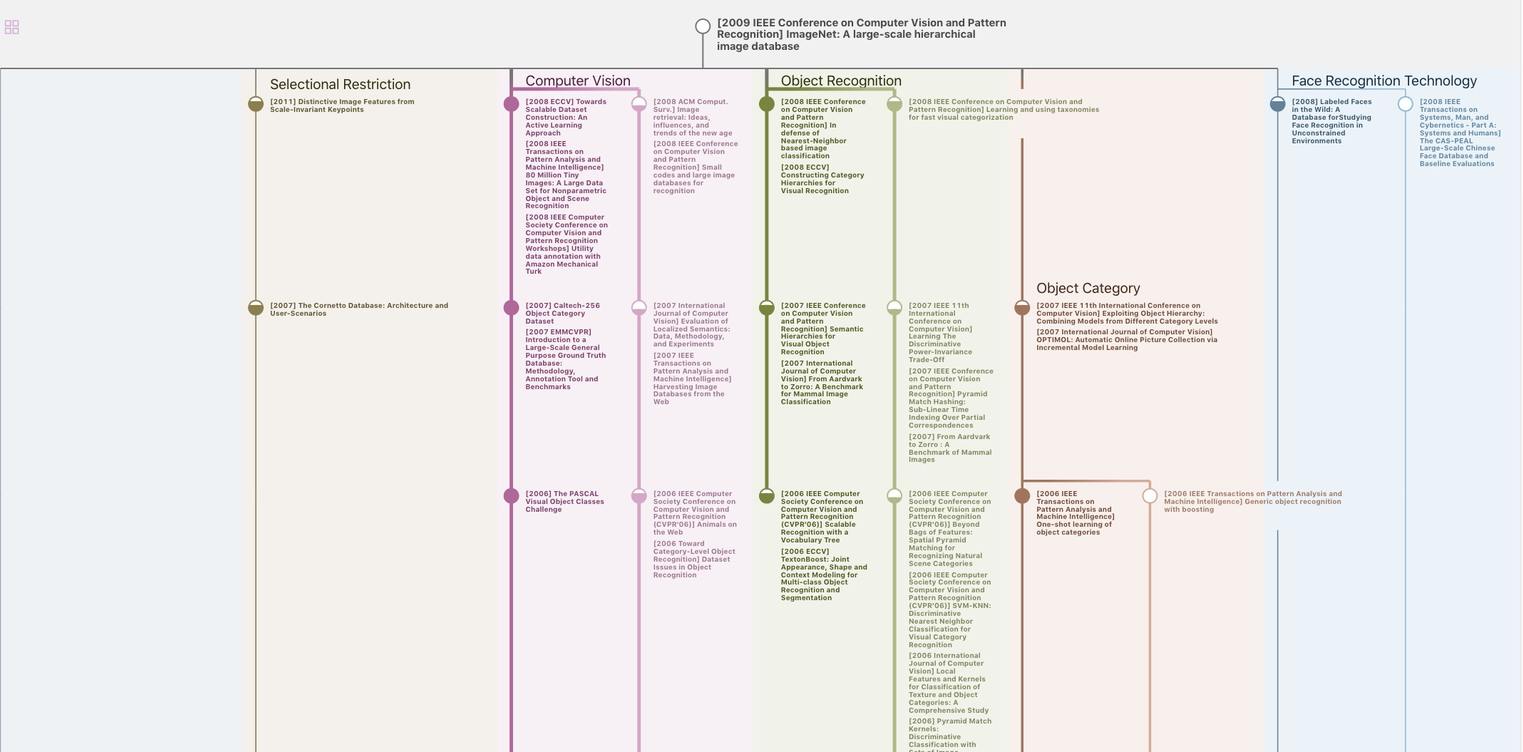
生成溯源树,研究论文发展脉络
Chat Paper
正在生成论文摘要