Robust Federated Learning for Heterogeneous Model and Data
INTERNATIONAL JOURNAL OF NEURAL SYSTEMS(2024)
摘要
Data privacy and security is an essential challenge in medical clinical settings, where individual hospital has its own sensitive patients data. Due to recent advances in decentralized machine learning in Federated Learning (FL), each hospital has its own private data and learning models to collaborate with other trusted participating hospitals. Heterogeneous data and models among different hospitals raise major challenges in robust FL, such as gradient leakage, where participants can exploit model weights to infer data. Here, we proposed a robust FL method to efficiently tackle data and model heterogeneity, where we train our model using knowledge distillation and a novel weighted client confidence score on hematological cytomorphology data in clinical settings. In the knowledge distillation, each participant learns from other participants by a weighted confidence score so that knowledge from clean models is distributed other than the noisy clients possessing noisy data. Moreover, we use symmetric loss to reduce the negative impact of data heterogeneity and label diversity by reducing overfitting the model to noisy labels. In comparison to the current approaches, our proposed method performs the best, and this is the first demonstration of addressing both data and model heterogeneity in end-to-end FL that lays the foundation for robust FL in laboratories and clinical applications.
更多查看译文
关键词
Robust federated learning,data and model heterogeneity,knowledge distillation,noisy label learning,collaborative machine learning
AI 理解论文
溯源树
样例
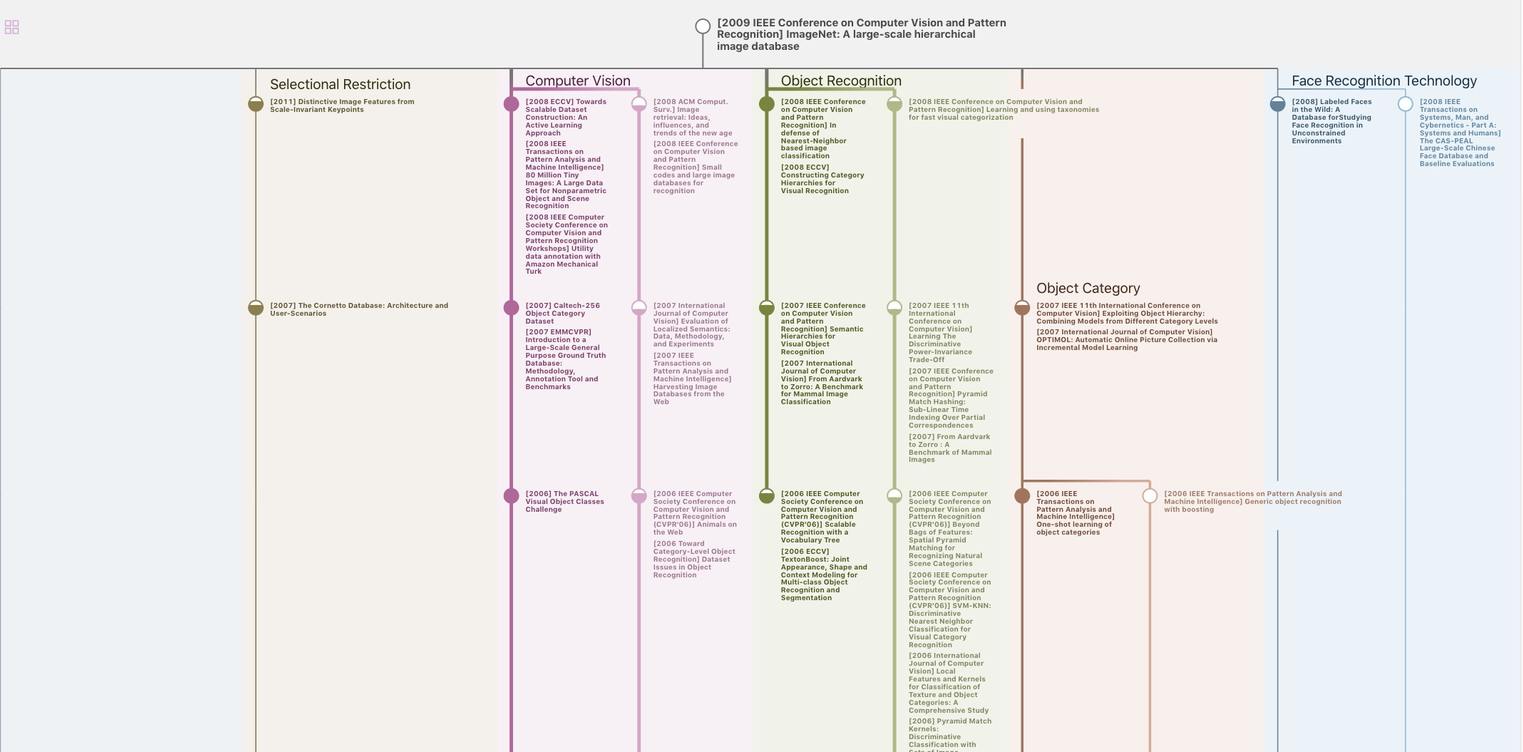
生成溯源树,研究论文发展脉络
Chat Paper
正在生成论文摘要