Principal Properties Attention Matching for Partial Domain Adaptation in Fault Diagnosis
IEEE TRANSACTIONS ON INSTRUMENTATION AND MEASUREMENT(2024)
摘要
Fault diagnosis plays a crucial role in the condition monitoring and health management of rotating machinery. In recent years, there has been a remarkable upsurge of interest in partial domain adaptive fault diagnosis models. The majority of advanced methodologies strive to alleviate the issue of negative transfer by reducing the influence of outlier classes. However, it is crucial to acknowledge that outlier classes within the source domain still encompass valuable sensor information, which can significantly enhance the effectiveness of knowledge transfer. To maximize the utility of outlier class samples instead of discarding them outright, we propose a method named principal properties attention matching (PPAM). First, we employ the principal properties extractor (PPE) to capture common features of fault instances from both shared and outlier classes, thereby characterizing the inherent relationships among the samples. Subsequently, we leverage the principal properties attention responder (PAR) to compute the properties-responsive weights, introducing an embedding-level weighting mechanism to achieve a more fine-grained matching and facilitate better domain adaptation in fault diagnosis. Comprehensive experiments performed under the Case Western Reserve University (CWRU), Paderborn, and PHM09 datasets demonstrate the effectiveness and superiority of the proposed method for industrial fault diagnosis. Specifically, on the more challenging Paderborn dataset, PPAM demonstrates a 2.77% increase in accuracy compared to the state-of-the-art (SOTA) approach.
更多查看译文
关键词
Fault diagnosis,Feature extraction,Training,Machinery,Knowledge transfer,Adaptation models,Testing,Attention matching,embedding-level weighting,fault diagnosis,partial domain adaptation (PDA),principal properties
AI 理解论文
溯源树
样例
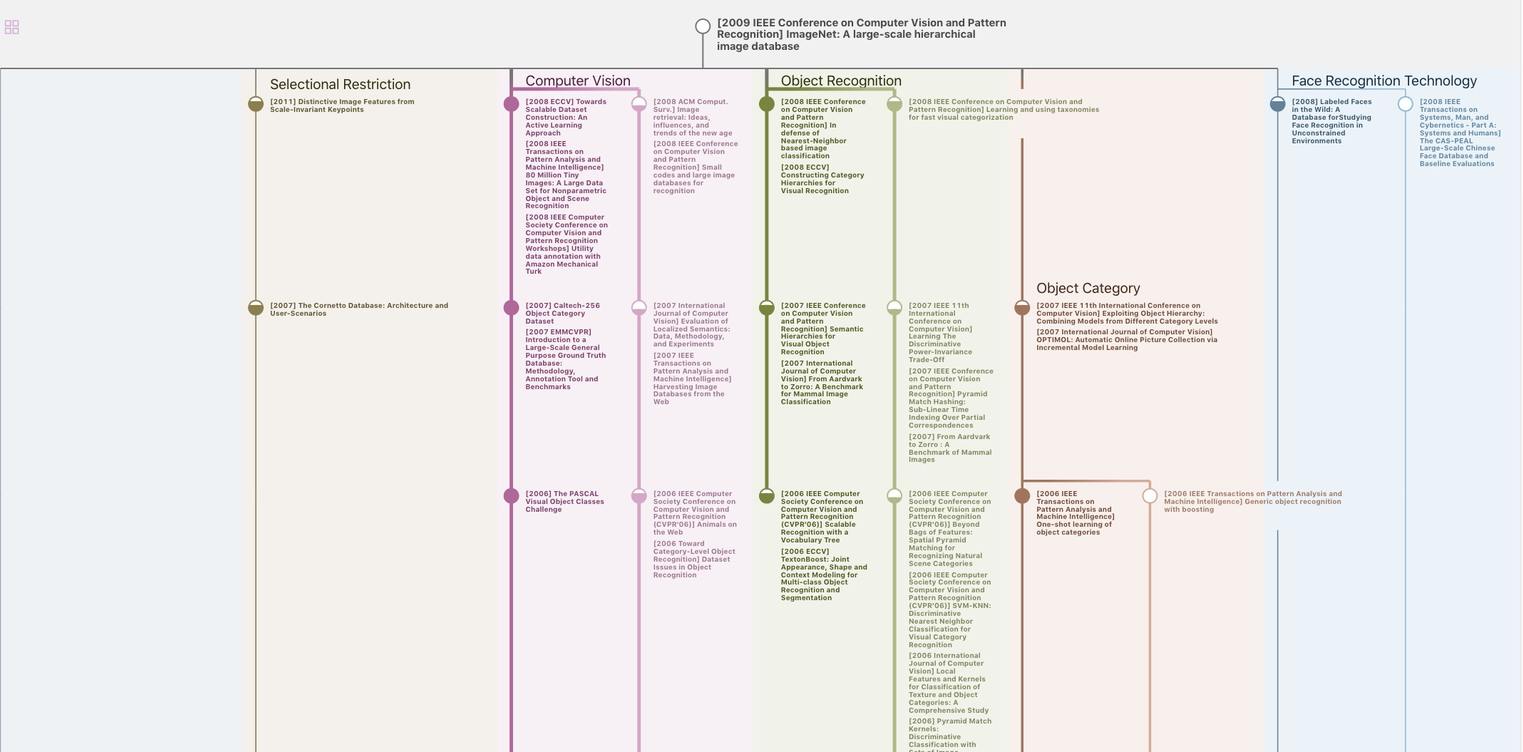
生成溯源树,研究论文发展脉络
Chat Paper
正在生成论文摘要