MSNCog: A Lightweight Faults Diagnosis System Based on Multiscale Transfer Model for Adaptive Infrared Perspective
IEEE TRANSACTIONS ON INSTRUMENTATION AND MEASUREMENT(2024)
摘要
The combination of deep learning and infrared imaging pioneered a new direction for health status monitoring in rotor-bearing systems. However, the single-view images, inadequate feature extraction, and limited labeled data restrict the precision of the diagnosis network. This article introduces a system called MultiScaleNetCog (MSNCog), a diagnosis system consisting of a multiview infrared thermography (IRT) image dataset under variable-speed conditions and a lightweight and multiscale convolutional network (MCNN). Initially, multiview infrared datasets are used to characterize compound faulty bearings at varying speeds. Subsequently, a shallow perception module (SPM) combined with a multistream dimension reduction module (MDRM) is proposed for both shallow and deep feature extraction. Furthermore, the optimal lightweight strategy is proposed for the convolution layer and classification layer for parameter sparsity and feature refinement. Finally, the parameter transfer is introduced to recognize the new defect bearings. Comparison against advanced networks and datasets (including time-frequency images and single-view data) demonstrates that MSNCog only needs 18 samples per defect to achieve a high performance of 96.92% (281 s). Meanwhile, the implementation of MSNCog on Raspberry Pi 4 achieves the accuracy of 95.21% with 3.12 frames/s, which verifies the deployment possibility of the proposed lightweight framework.
更多查看译文
关键词
Lightweight deployment,multiscale,multiview infrared thermography (IRT) images,MultiScaleNetCog (MSNCog),parameter transfer
AI 理解论文
溯源树
样例
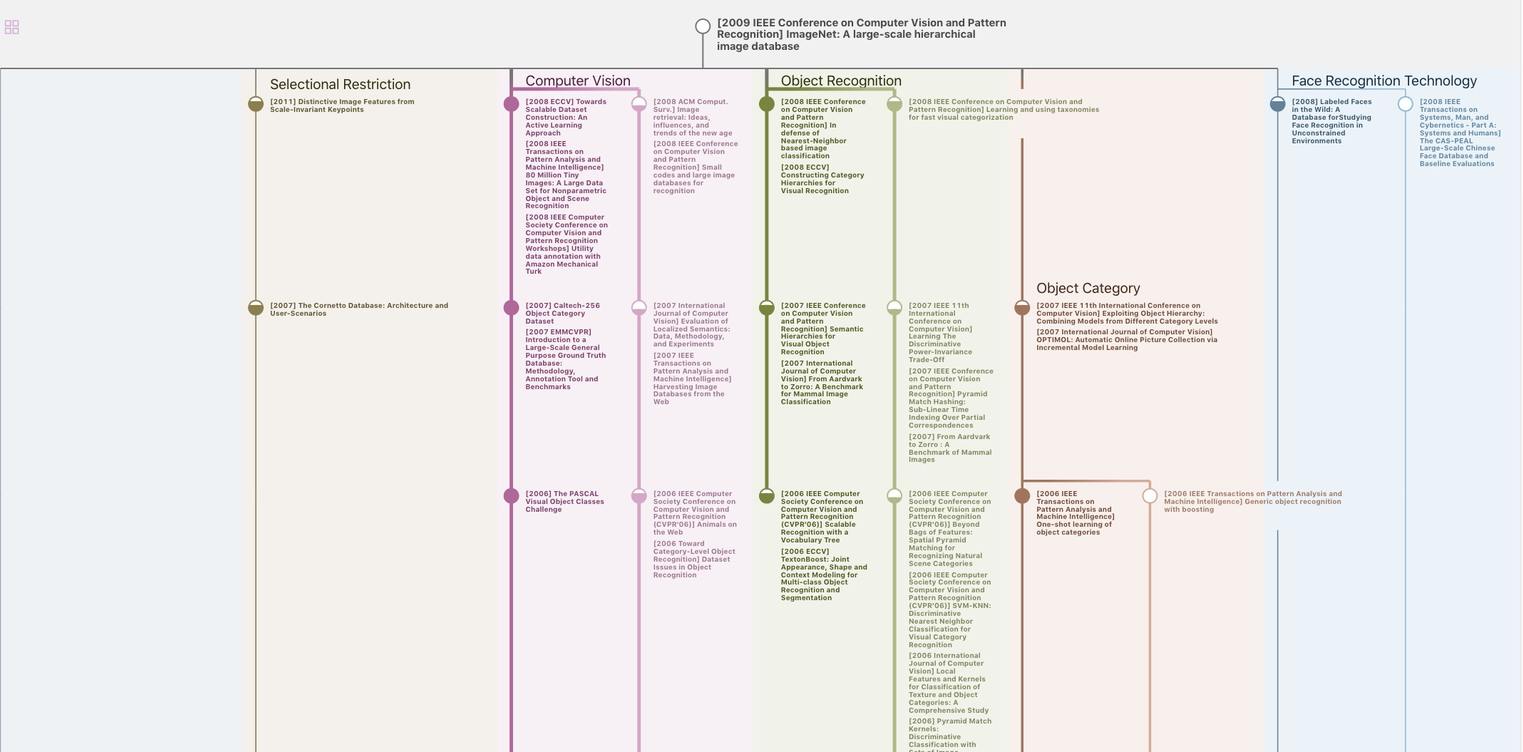
生成溯源树,研究论文发展脉络
Chat Paper
正在生成论文摘要