Testing Stationarity and Statistical Independence of Multistatic/Polarimetric Sea-Clutter With Application to NetRAD Data
IEEE Transactions on Geoscience and Remote Sensing(2024)
摘要
The design of bespoke adaptive detection schemes relying on the joint use of multistatic/polarimetric measurements requires a preliminary statistical inference on the clutter interference environment. This is of paramount importance to develop an analytic model for the received signal samples, which is mandatory for the synthesis of radar detectors. In this respect, the aim of this article is the development of suitable learning tools to study some important statistical features of the sea-clutter environment perceived at the nodes of a multistatic/polarimetric radar system. Precisely, the stationarity of the data in the slow-time domain is first assessed by resorting to generalized inner product (GIP) based statistics. Then, the possible presence of structural symmetries in the clutter covariance matrices is investigated. Finally, relationships between some statistical parameters characterizing the sea-clutter returns on the bistatic polarimetric channels are explored via specific sequential hypothesis testing. This research activity is complemented by the use of radar returns measured via the netted RADar (NetRAD), which collects simultaneously monostatic and bistatic polarimetric measurements. The results indicate that the analyzed data can be modeled as drawn from a stationary Gaussian process within the coherence time. In addition, the bistatic returns on the different polarimetric channels can be assumed statistically independent with speckle components possibly exhibiting proportional/equal covariance matrices depending on the transmit/receive polarization and bistatic geometry.
更多查看译文
关键词
Covariance matrix structure,data homogeneity,generalized inner product (GIP),model order selection (MOS),multistatic/polarimetric radar,proportionality/equality of covariance matrices,sea-clutter,spherically invariant random process (SIRP)
AI 理解论文
溯源树
样例
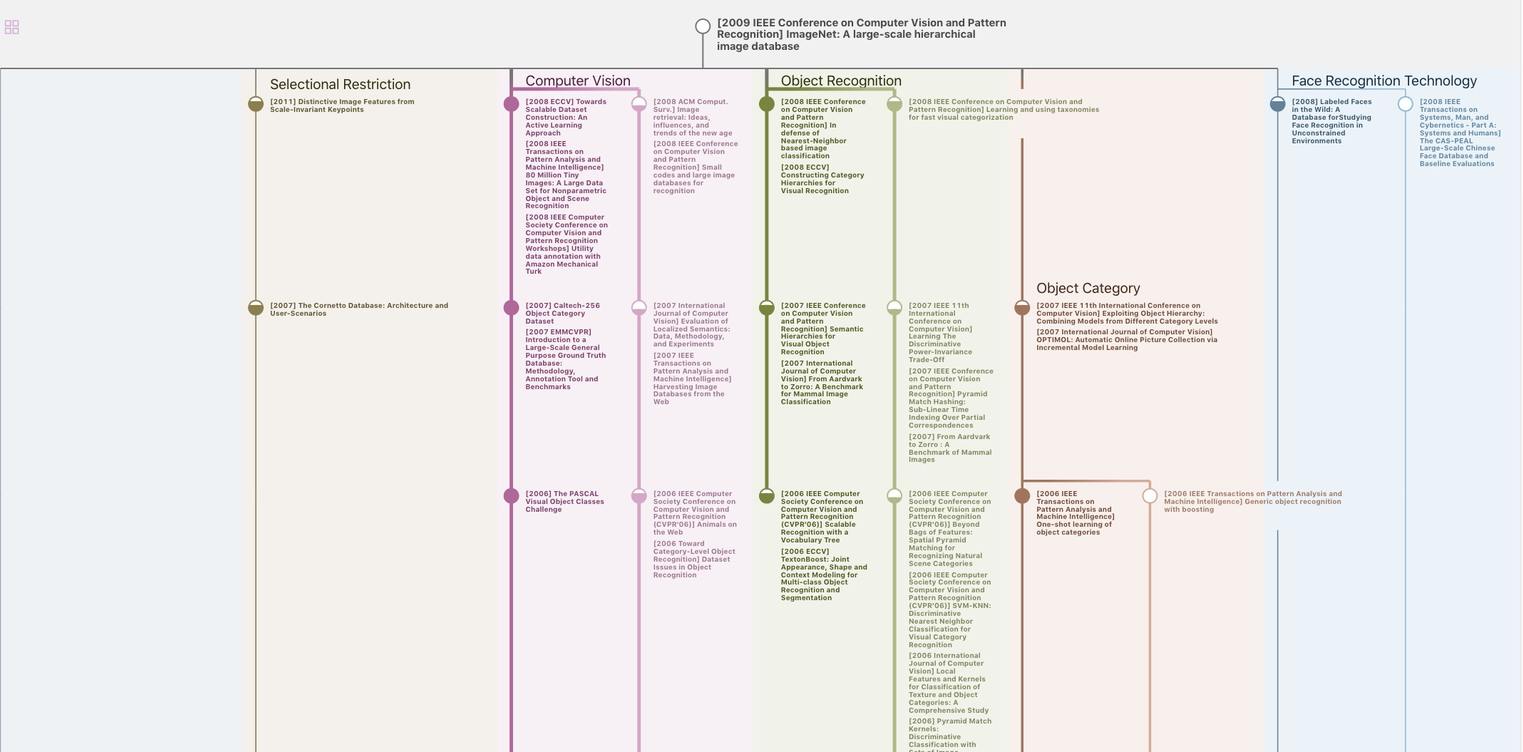
生成溯源树,研究论文发展脉络
Chat Paper
正在生成论文摘要