Enhancing low-light images via skip cross-attention fusion and multi-scale lightweight transformer
Journal of Real-Time Image Processing(2024)
摘要
Images captured in environments with poor lighting conditions often suffer from insufficient brightness, significant noise, and color distortion, which is highly detrimental to subsequent high-level vision tasks. Low-light image enhancement requires effective feature extraction and fusion, and the advantages of transformer and convolution in image processing are complementary. Therefore, it is an intentional exploration to combine them in image enhancement. In this paper, we propose a novel UNet-like method for enhancing low-light images. Transformer blocks are stacked to form the encoder, and convolutional blocks are utilized in the decoder. First, considering that the Transformer can effectively capture global information and convolution can obtain local information, this paper improves the lightweight Transformer by integrating multi-scale depth-wise convolution into the feedforward network to extract comprehensive features. Then, we design a Skip Cross-Attention Module to replace the traditional skip connection, which combines feature maps from different stages of the encoder and decoder. To achieve better feature fusion, this module employs two masks: the Top- k Mask and the adaptive V Channel Mask based on maximum entropy. The Top- k Mask will filter out unfavorable features by preserving the top k scores of attention map, and the V Channel Mask utilizes the corrected V channel of the image as an illumination guide for enhancement. Finally, extensive experiments on seven datasets demonstrate that our method achieves good performance in both subjective and objective evaluations. Specifically, the runtime on LOL-v2-real dataset demonstrates that our method is close to achieving real-time performance.
更多查看译文
关键词
Image enhancement,Feature fusion,Skip-cross attention,Transformer,V channel
AI 理解论文
溯源树
样例
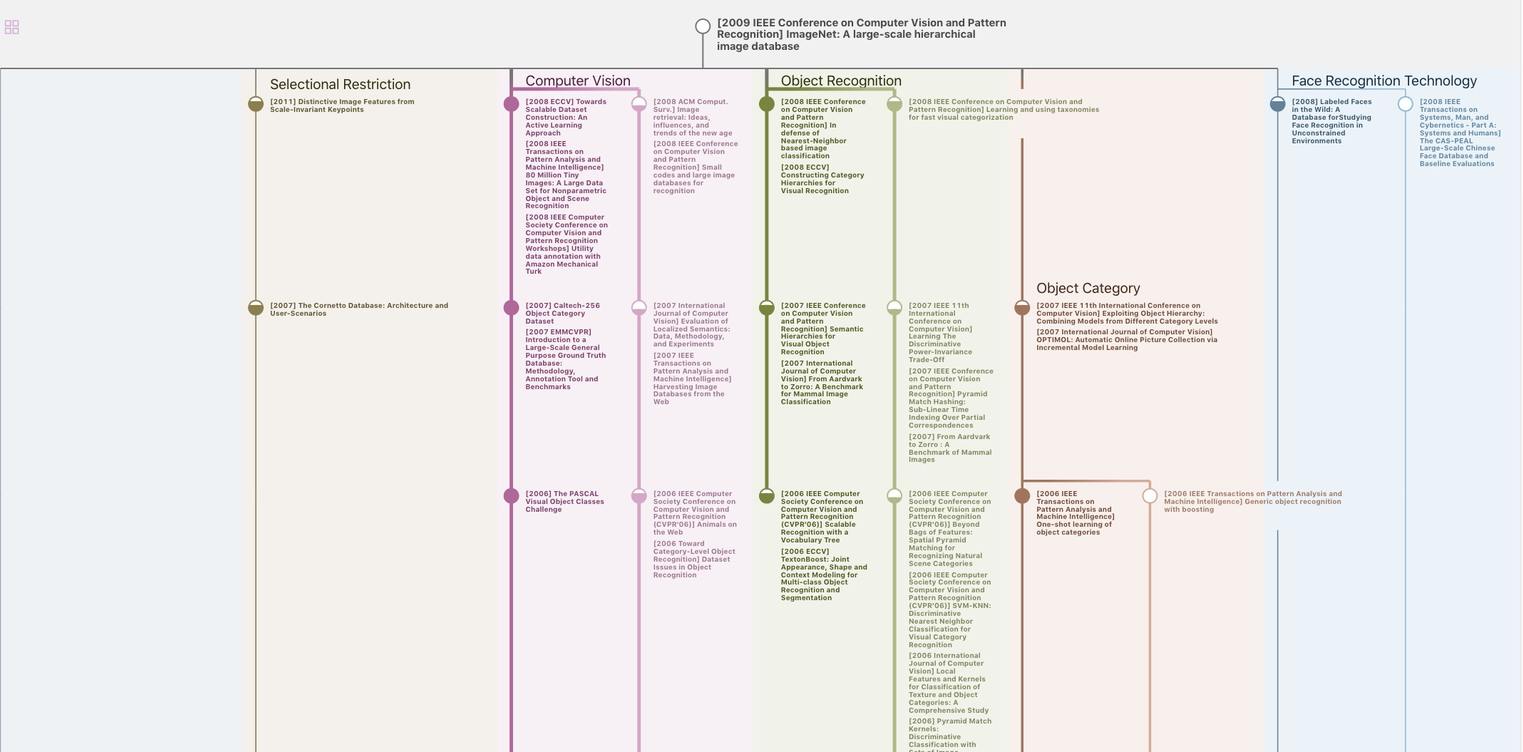
生成溯源树,研究论文发展脉络
Chat Paper
正在生成论文摘要