A Deep Reinforcement Learning Approach for Load Balancing in Open Radio Access Networks.
Global Communications Conference(2023)
摘要
The Open RAN paradigm offers data-driven, intelligent optimization of the radio access network (RAN). The disaggregated nature of the Open RAN combined with virtualization on general-purpose CPUs with limited computation capacity creates different load types at multiple levels, making it more challenging to balance the load within the network. This paper proposes a learning framework that learns the assignment of users (UEs) to network nodes to balance the communication and computation load in the network. The framework incorporates communication resources consumed by the users in the radio unit (RU), and computation resources needed for baseband processing in the virtualized distributed unit (DU). The goal is thus to balance the communication load between RUs and the computation load between DUs to avoid overloading network elements or to handle higher peak data rate demands when new users arrive in the network. We apply a novel utility-based approach to jointly optimize the UE-RU and RU-DU assignments taking into account the users' QoS (quality of service) requirements. Simulations demonstrate that the proposed method generates the assignments that significantly improve the network load conditions compared to baseline schemes, thereby enabling more available communication and computation resources for incoming peak data rate users in the network.
更多查看译文
关键词
O-RAN,Deep Reinforcement Learning,Load Balancing,User Association,Machine Learning
AI 理解论文
溯源树
样例
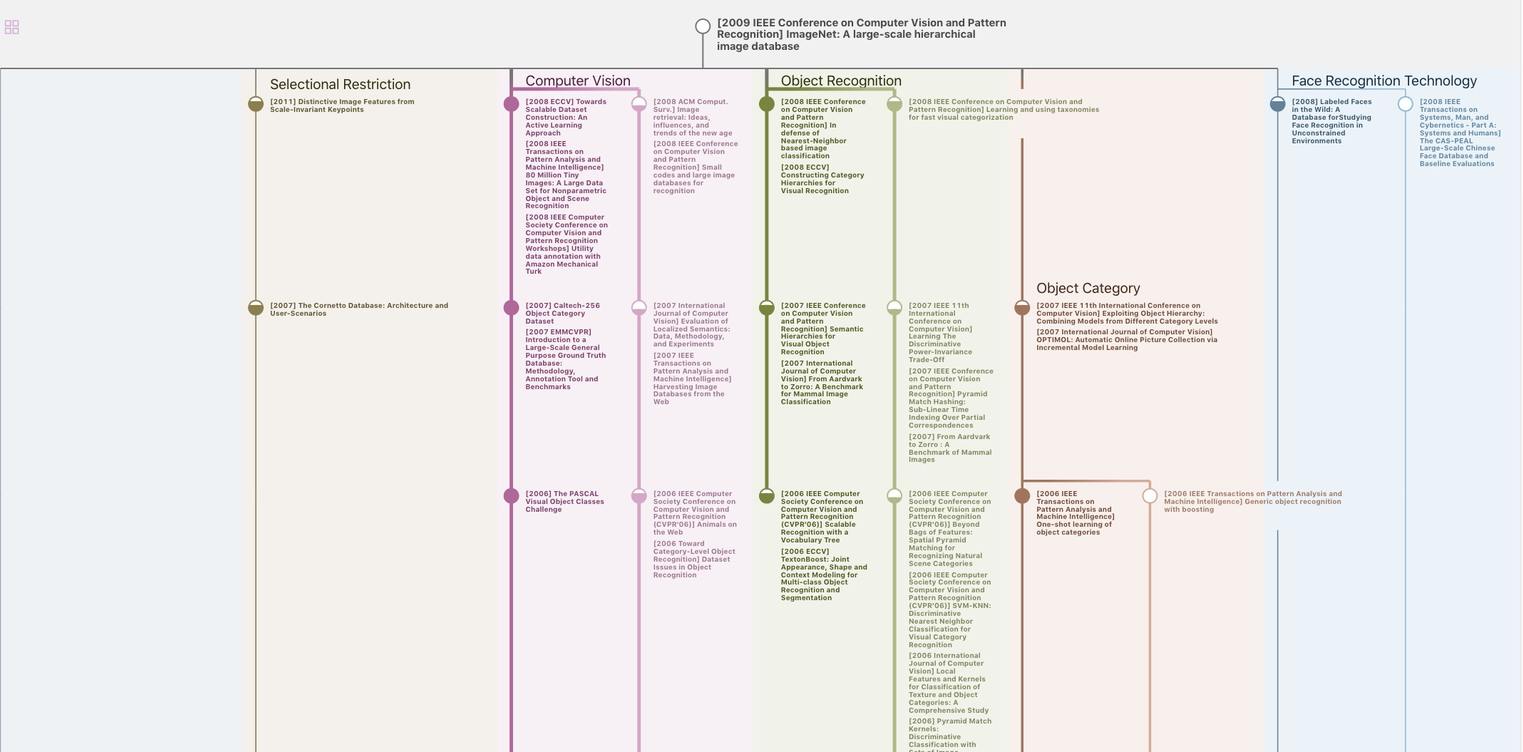
生成溯源树,研究论文发展脉络
Chat Paper
正在生成论文摘要