A Spatial Generative Adversarial Network-based Signal Detection for MIMO-ODDM Systems.
Global Communications Conference(2023)
摘要
The multiple-input multiple-output over orthogonal delay-Doppler division multiplexing (MIMO-ODDM) has recently attracted great interest as a promising solution for high-mobility systems. To achieve its full potential, signal detection becomes a critical issue, while the performance of the existing methods is yet to be satisfactory. To address this issue, we propose a novel signal detection approach called SG-ODDM, which utilizes a spatial-based generative adversarial network (spatial-based GAN) for accurate and interference-resistant performance. We creatively design a spatial-based GAN for comprehensive feature extraction and interference mitigation. In the spatial-based GAN, we develop an attention-based generator with multi-domain feature (AGMF) to effectively reconstruct signals for detection by extracting and utilising signal characteristics across multiple domains, e.g., delay, Doppler, and spatial domains. Moreover, we develop a self-attention-based discriminator with multi-domain feature (SDMF) to guide AGMF to mitigate the impact of interference in MIMO systems, thereby improving the quality of the generated/reconstructed data from AGMF. Additionally, we design a novel hybrid loss function to fully exploit signal features in the multiple domains for detection. Through extensive simulations, we demonstrate that SG-ODDM outperforms state-of-the-art related works regarding detection accuracy and interference resilience.
更多查看译文
关键词
Signal Detection,Loss Function,Detection Accuracy,Characteristic Signals,Generative Adversarial Networks,Spatial Domain,Multiple-input Multiple-output,Multiple-input Multiple-output Systems,Neural Network,Convolutional Neural Network,Superior Performance,Convolutional Layers,Spatial Information,Recurrent Neural Network,Detection Model,Fully-connected Layer,Convolutional Block,Doppler Shift,Orthogonal Frequency Division Multiplexing,Attention Weights,Doppler Indices,Reconstruction Loss,Peak-to-average Power Ratio,Self-attention Mechanism,Principal Directions
AI 理解论文
溯源树
样例
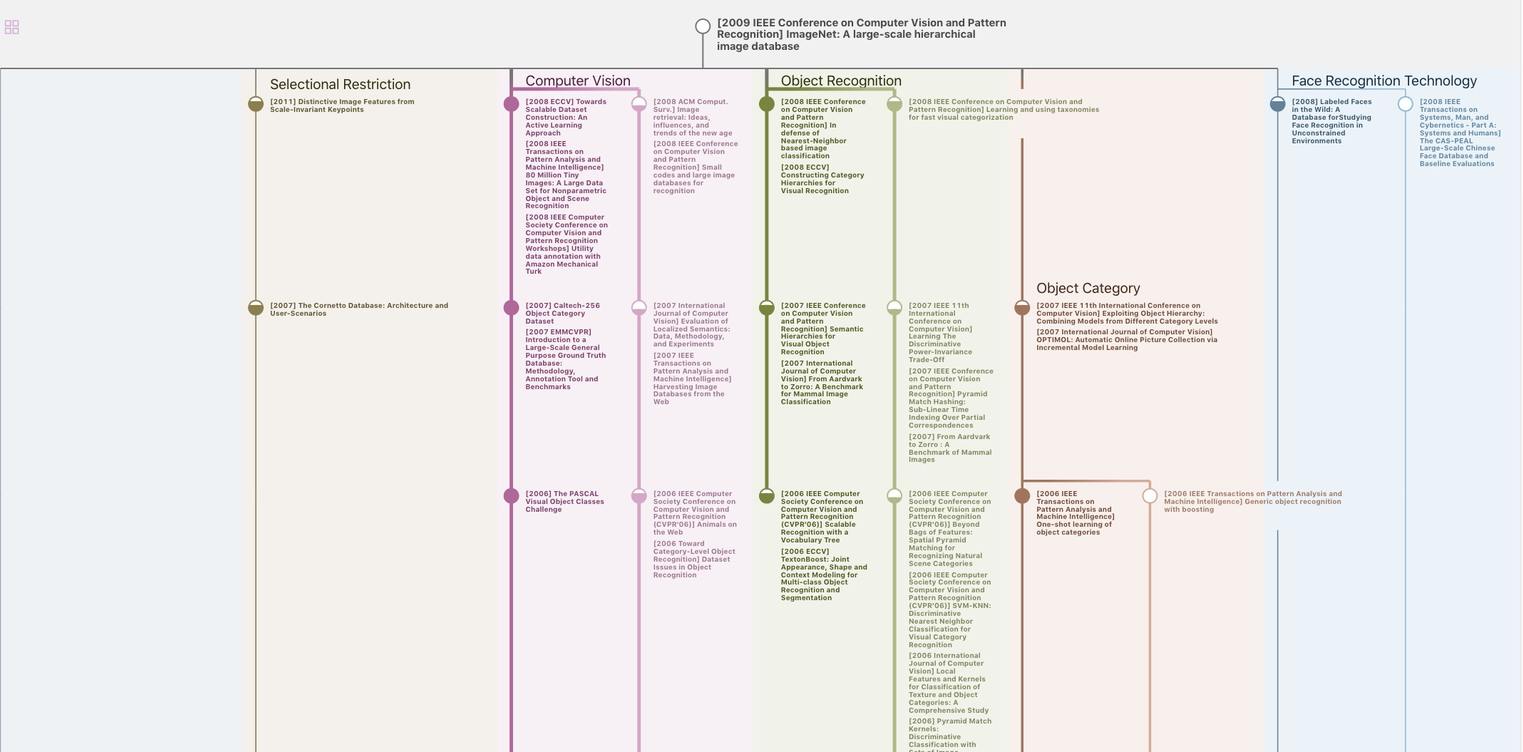
生成溯源树,研究论文发展脉络
Chat Paper
正在生成论文摘要