FedChallenger: Challenge-Response-Based Defence for Federated Learning Against Byzantine Attacks.
Global Communications Conference(2023)
摘要
Federated Learning (FL) is an emerging paradigm that enables multiple clients to train a global model collaboratively without sharing their privacy-sensitive data. However, one of the significant challenges in FL is the aggregation of the model updates from different client devices, as malicious participants acting as Byzantine attackers can craft the model update and poison the global model. The state-of-the-art defence mechanisms mostly rely on aggregation-based security defences to improve the degraded accuracy. However, preventing attacker's participation in the training can have an impact on improving the global model's accuracy. Therefore, in this paper, FedChallenger, a dual-layer defence mechanism, is proposed, which attempts to detect and prevent malicious participation in the FL training process in its first layer. The other layer incorporates a trimmed-mean aggregation strategy, where pairwise cosine similarity identifies malicious updates and removes entire client updates from federated averaging. Extensive experiments using the BloodMNIST dataset validate that the FedChallenger gains nearly 85%, 80%, 15%, and 4% accuracy with more than 1.2 times faster convergence rate over the state-of-the-art Byzantine resilient aggregation strategies called FedAvg, Fang, Krum, and Trimmed-Mean approach, respectively, on 40% compromised devices. Above all, it shows consistently better results than them in both attack and non-attack scenarios.
更多查看译文
关键词
Byzantine Attacks,Challenge-Response,Federated Learning,Data Poisoning,Model Poisoning
AI 理解论文
溯源树
样例
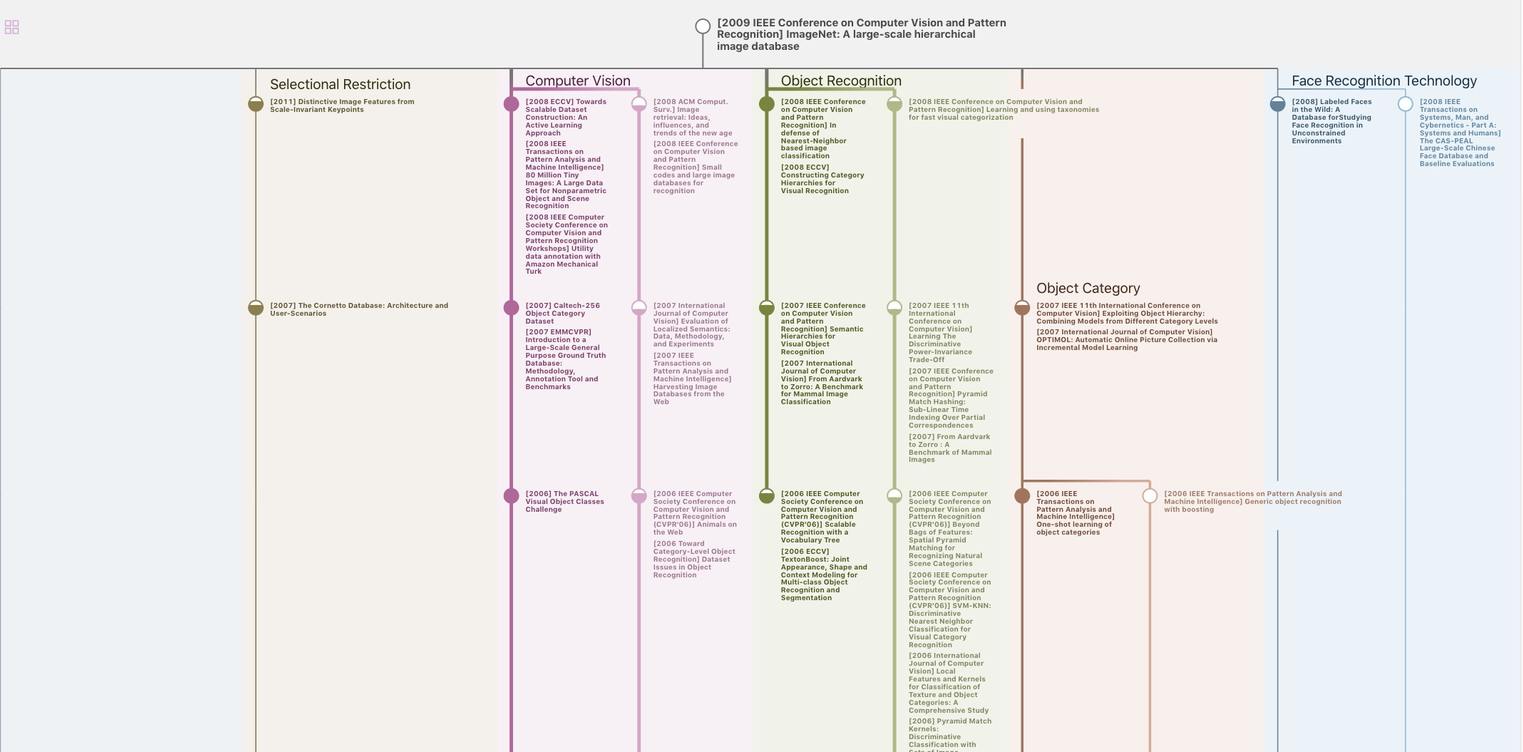
生成溯源树,研究论文发展脉络
Chat Paper
正在生成论文摘要