Federated Deep Reinforcement Learning for Task Participation in Mobile Crowdsensing
IEEE CONFERENCE ON GLOBAL COMMUNICATIONS, GLOBECOM(2023)
摘要
Mobile Crowdsensing (MCS) is a promising distributed sensing architecture that harnesses the power of sensors on mobile units (MUs) to perform sensing tasks. The MCS is a dynamic system in which the requirements of the sensing tasks, the MUs' conditions and the available resources change over time. The performance of an MCS system depends on the selection of the MUs participating in each sensing task. However, this is not a trivial problem. An optimal task participation strategy requires non-causal knowledge about the dynamic MCS system, a requirement that cannot be fulfilled in real implementations. Moreover, centralized optimization-based approaches do not scale with increasing number of participating MUs and often ignore the MUs' preferences. To overcome these challenges, in this paper we propose a novel multi-agent federated deep reinforcement learning algorithm (FDRL-PPO) which does not need this perfect non-causal knowledge, but instead, enables the MUs to learn their own task participation strategies based on their own conditions, available resources, and preferences. Through federated learning, the MUs share their learned strategies without disclosing sensitive information, enabling a robust and scalable task participation scheme. Numerical evaluations validate the effectiveness and efficiency of FDRL-PPO in comparison with reference schemes.
更多查看译文
关键词
Deep Learning,Task Participants,Deep Reinforcement Learning,Mobile Crowdsensing,Scalable,System Dynamics,Learning Algorithms,Learning Strategies,Optimization Task,Federated Learning,Mobile Unit,Perfect Knowledge,Deep Reinforcement Learning Algorithm,Time Step,Artificial Neural Network,Values In The Range,Global Model,Stochastic Gradient Descent,Task Execution,Energy Harvesting,Proximal Policy Optimization,Causal Knowledge,Policy Network,Training Episodes,Future Tasks,Task Allocation,Policy Parameters,Selection Task,Wireless Sensor Networks,Finite Time Horizon
AI 理解论文
溯源树
样例
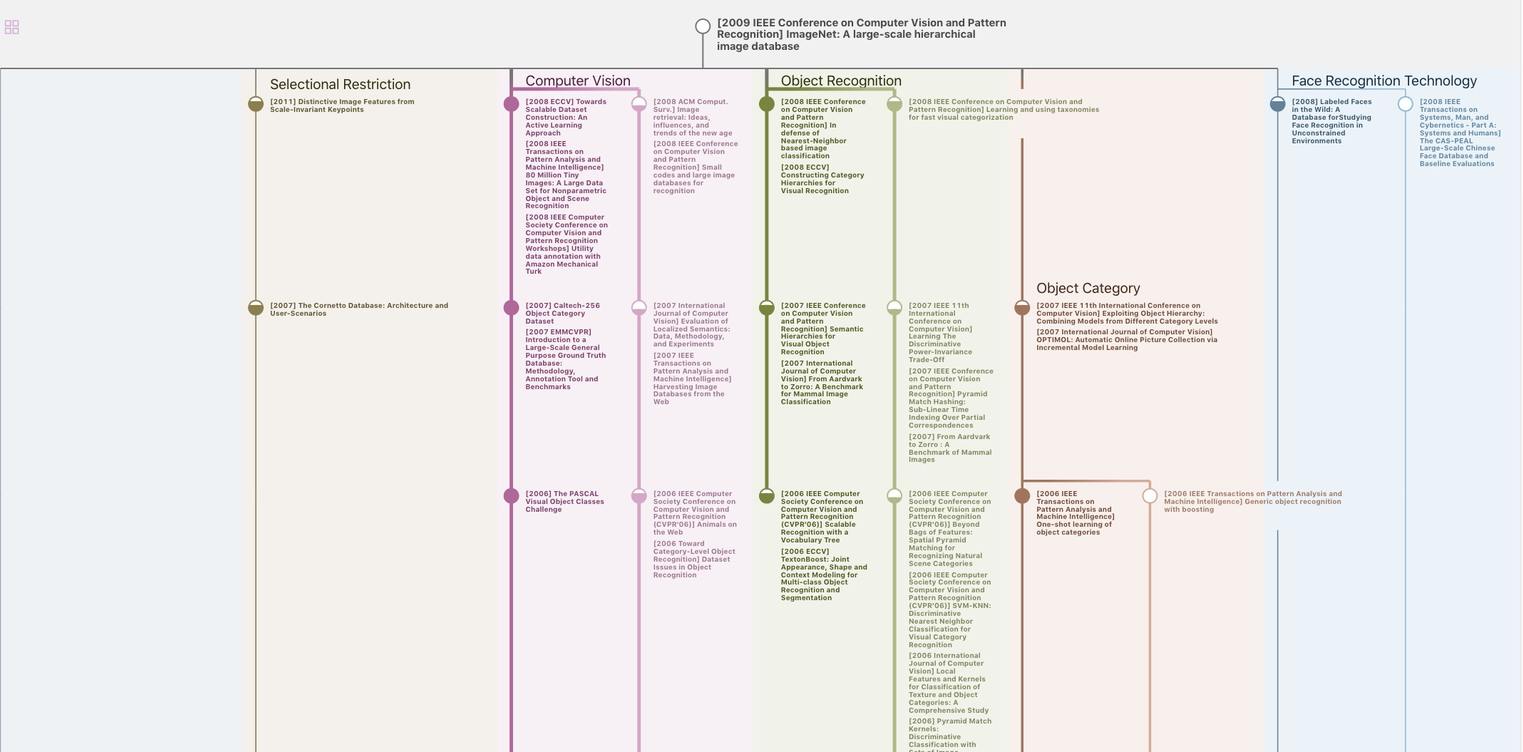
生成溯源树,研究论文发展脉络
Chat Paper
正在生成论文摘要