Performance Modeling and Analysis of Distributed Deep Neural Network Training with Parameter Server
IEEE CONFERENCE ON GLOBAL COMMUNICATIONS, GLOBECOM(2023)
摘要
With the growth of dataset size and the development of hardware accelerators, the application of deep neural networks (DNN) in various fields has made great breakthroughs. In order to improve the training speed of DNN, distributed training has been widely used. However, the imbalance between computation and communication makes distributed training difficult to achieve maximum efficiency. Therefore there is a need to detect the bottleneck state and verify the effect of some optimization schemes. Testing on a physical cluster incurs additional time and cost overhead. This paper builds a DNN-specific performance model that is used for bottleneck detection and tuning at a low cost. We build this model through detailed analysis and reasonable assumptions. We also focus on fine-grained modeling of scalability and network components, which are key factors affecting performance. Then we verify the performance model with an average error of 5% on testbed and emulator. Finally, we provide use cases of the performance model.
更多查看译文
关键词
Distributed Training,Performance Modeling,Communication Optimization
AI 理解论文
溯源树
样例
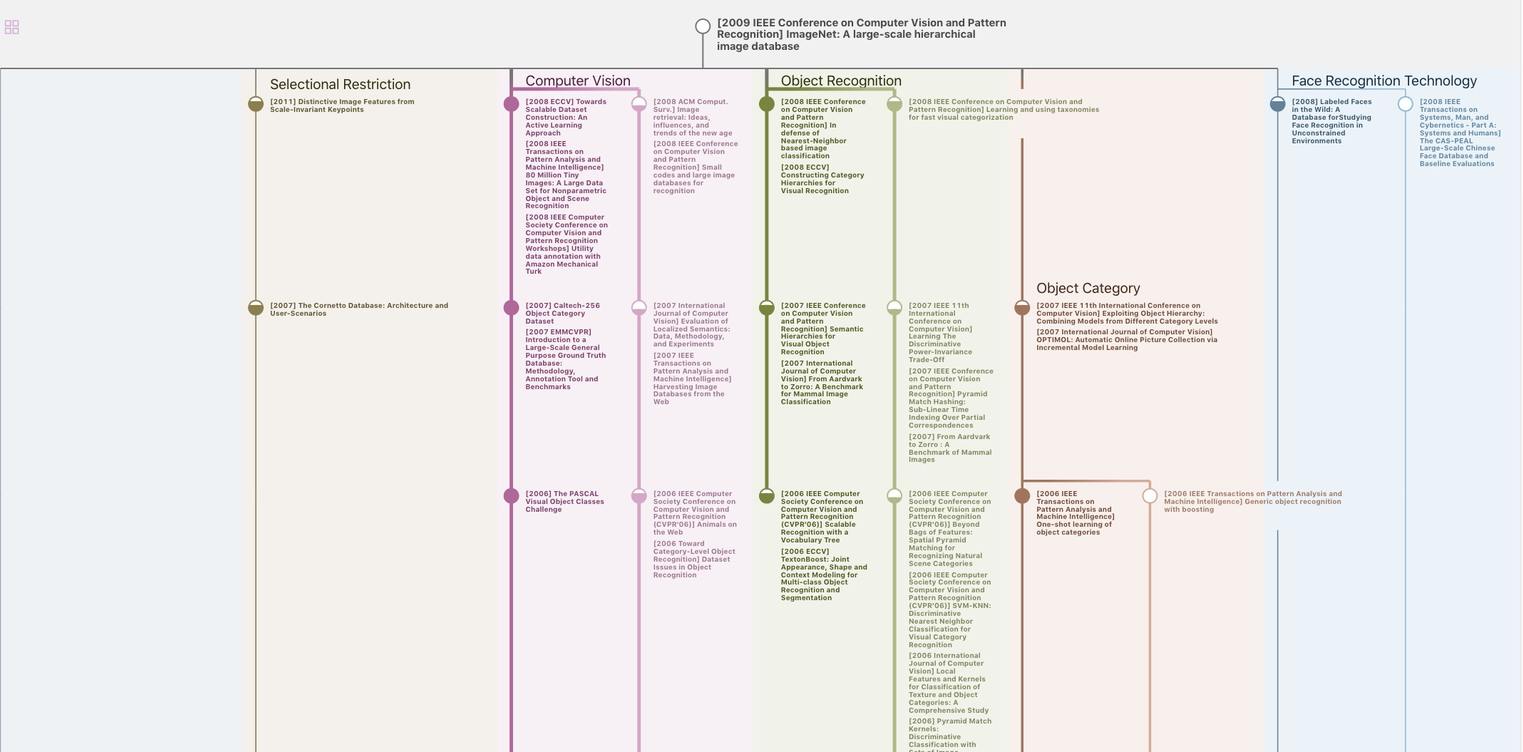
生成溯源树,研究论文发展脉络
Chat Paper
正在生成论文摘要