Temporal Logic Specification-Conditioned Decision Transformer for Offline Safe Reinforcement Learning
CoRR(2024)
摘要
Offline safe reinforcement learning (RL) aims to train a constraint
satisfaction policy from a fixed dataset. Current state-of-the-art approaches
are based on supervised learning with a conditioned policy. However, these
approaches fall short in real-world applications that involve complex tasks
with rich temporal and logical structures. In this paper, we propose temporal
logic Specification-conditioned Decision Transformer (SDT), a novel framework
that harnesses the expressive power of signal temporal logic (STL) to specify
complex temporal rules that an agent should follow and the sequential modeling
capability of Decision Transformer (DT). Empirical evaluations on the DSRL
benchmarks demonstrate the better capacity of SDT in learning safe and
high-reward policies compared with existing approaches. In addition, SDT shows
good alignment with respect to different desired degrees of satisfaction of the
STL specification that it is conditioned on.
更多查看译文
AI 理解论文
溯源树
样例
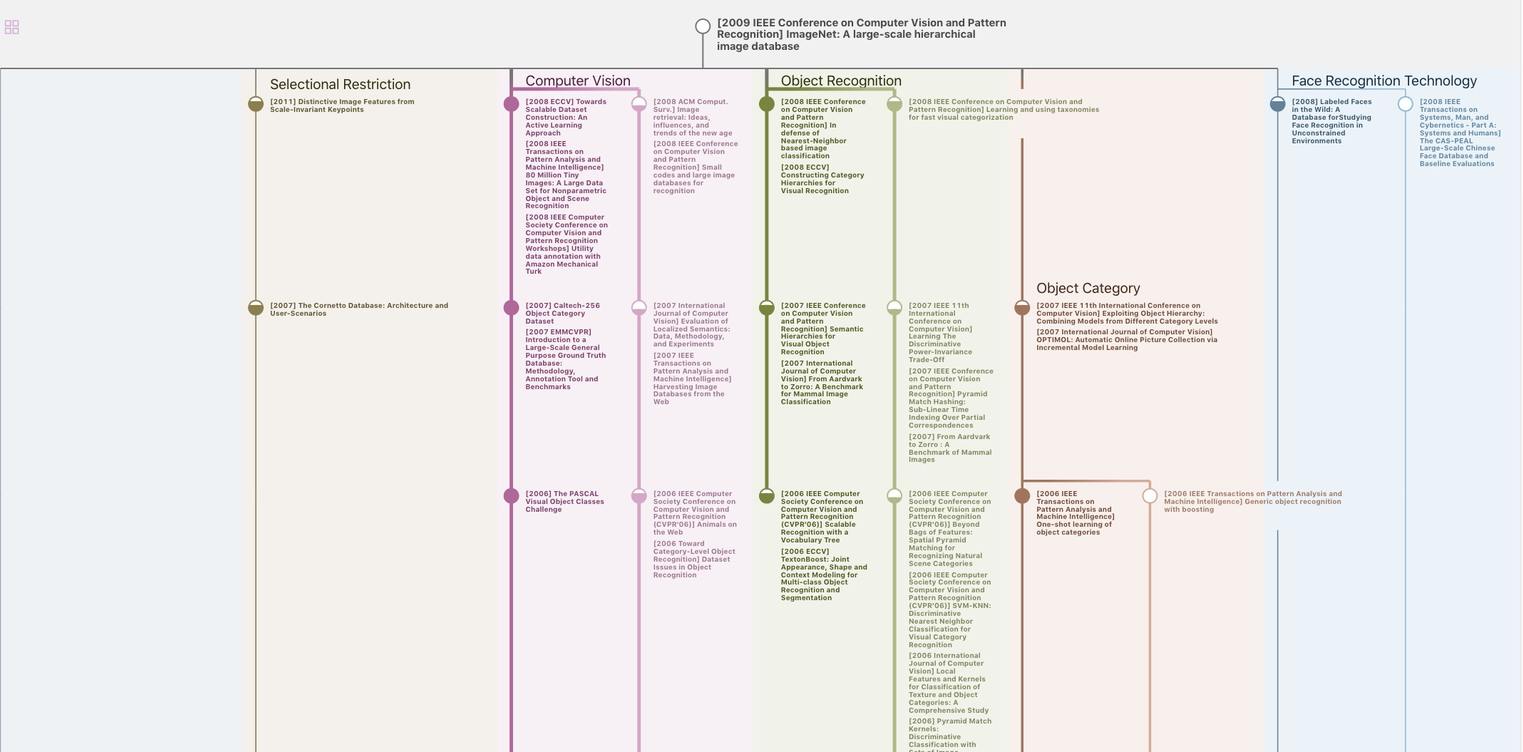
生成溯源树,研究论文发展脉络
Chat Paper
正在生成论文摘要