When Scaling Meets LLM Finetuning: The Effect of Data, Model and Finetuning Method
ICLR 2024(2024)
摘要
While large language models (LLMs) often adopt finetuning to unlock their
capabilities for downstream applications, our understanding on the inductive
biases (especially the scaling properties) of different finetuning methods is
still limited. To fill this gap, we conduct systematic experiments studying
whether and how different scaling factors, including LLM model size,
pretraining data size, new finetuning parameter size and finetuning data size,
affect the finetuning performance. We consider two types of finetuning –
full-model tuning (FMT) and parameter efficient tuning (PET, including prompt
tuning and LoRA), and explore their scaling behaviors in the data-limited
regime where the LLM model size substantially outweighs the finetuning data
size. Based on two sets of pretrained bilingual LLMs from 1B to 16B and
experiments on bilingual machine translation and multilingual summarization
benchmarks, we find that 1) LLM finetuning follows a powerbased multiplicative
joint scaling law between finetuning data size and each other scaling factor;
2) LLM finetuning benefits more from LLM model scaling than pretraining data
scaling, and PET parameter scaling is generally ineffective; and 3) the optimal
finetuning method is highly task- and finetuning data-dependent. We hope our
findings could shed light on understanding, selecting and developing LLM
finetuning methods.
更多查看译文
关键词
LLM finetuning,Scaling Laws,Full-model finetuning,Parameter efficient tuning,Machine Translation,Multilingual Summarization
AI 理解论文
溯源树
样例
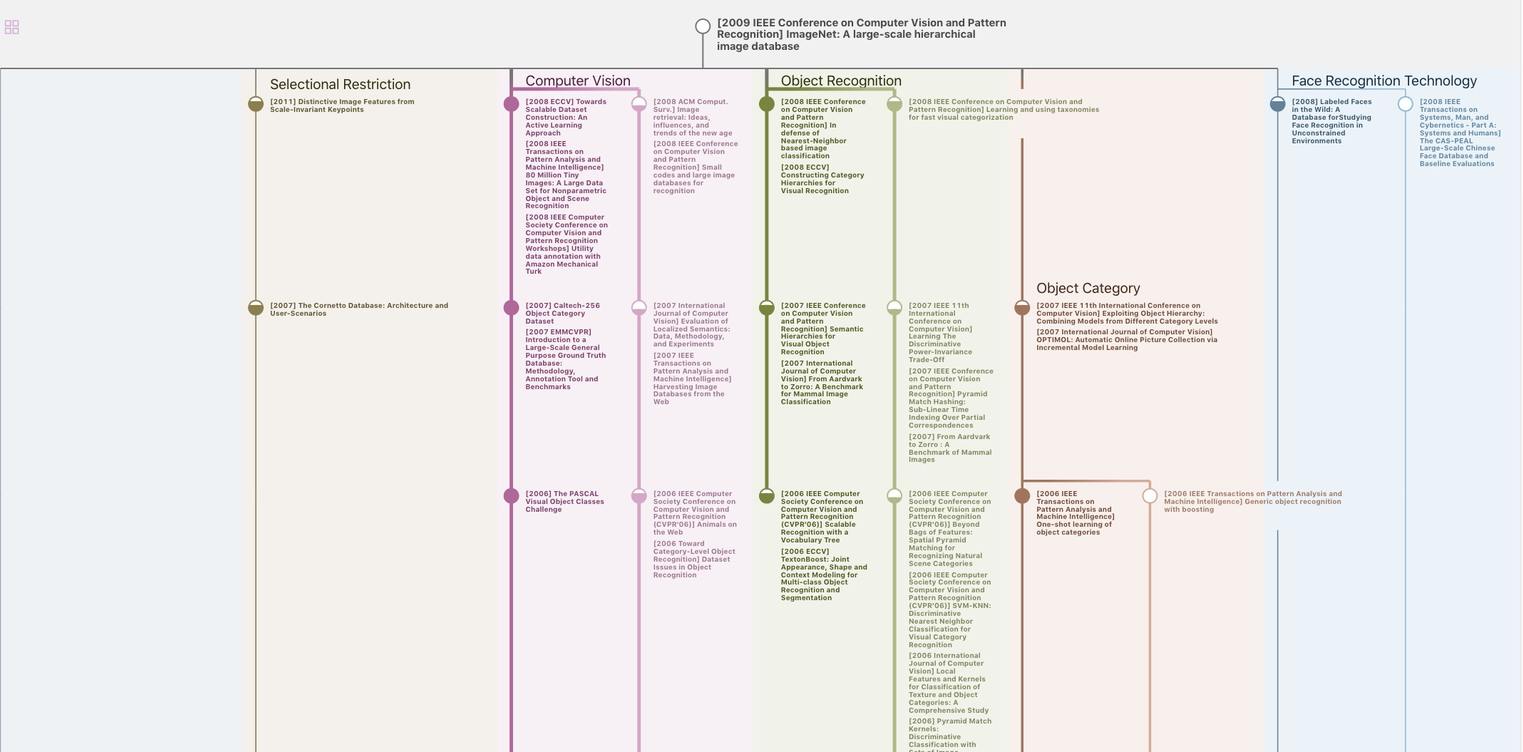
生成溯源树,研究论文发展脉络
Chat Paper
正在生成论文摘要