Self-Tuning Network Control Architectures with Joint Sensor and Actuator Selection
CoRR(2024)
摘要
We formulate a mathematical framework for designing a self-tuning network
control architecture, and propose a computationally-feasible greedy algorithm
for online architecture optimization. In this setting, the locations of active
sensors and actuators in the network, as well as the feedback control policy
are jointly adapted using all available information about the network states
and dynamics to optimize a performance criterion. We show that the case with
full-state feedback can be solved with dynamic programming, and in the
linear-quadratic setting, the optimal cost functions and policies are piecewise
quadratic and piecewise linear, respectively. Our framework is extended for
joint sensor and actuator selection for dynamic output feedback control with
both control performance and architecture costs. For large networks where
exhaustive architecture search is prohibitive, we describe a greedy heuristic
for actuator selection and propose a greedy swapping algorithm for joint sensor
and actuator selection. Via numerical experiments, we demonstrate a dramatic
performance improvement of greedy self-tuning architectures over fixed
architectures. Our general formulation provides an extremely rich and
challenging problem space with opportunities to apply a wide variety of
approximation methods from stochastic control, system identification,
reinforcement learning, and static architecture design for practical
model-based control.
更多查看译文
AI 理解论文
溯源树
样例
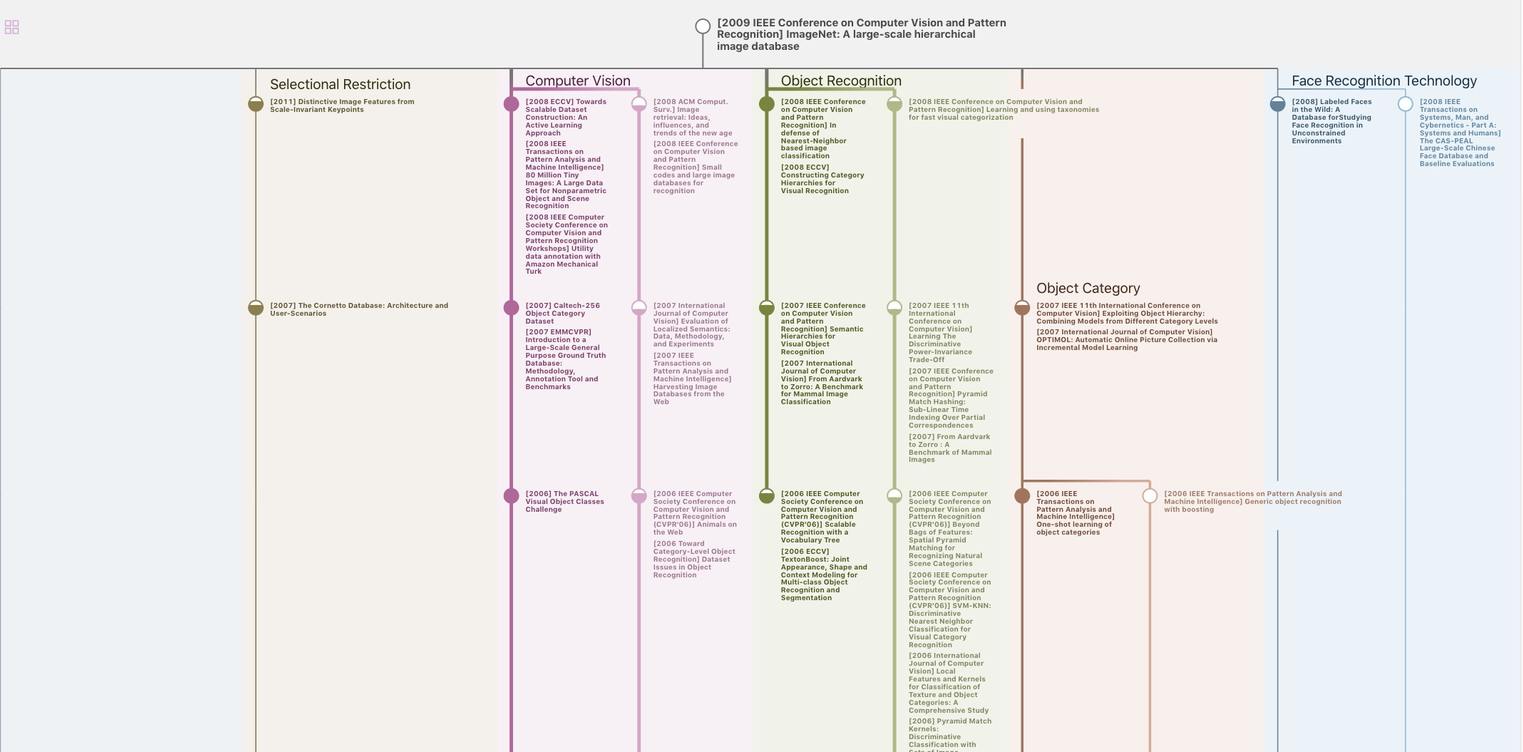
生成溯源树,研究论文发展脉络
Chat Paper
正在生成论文摘要