Data-driven discovery of interpretable Lagrangian of stochastically excited dynamical systems
Computer Methods in Applied Mechanics and Engineering(2024)
摘要
Exploring the intersection of deterministic and stochastic dynamics, this paper delves into Lagrangian discovery for conservative and non-conservative systems under stochastic excitation. Traditional Lagrangian frameworks, adept at capturing deterministic behavior, are extended to incorporate stochastic excitation. The study critically evaluates recent computational methodologies for learning Lagrangians from observed data, highlighting the limitations in interpretability and the exclusion of stochastic excitation. To address these gaps, an automated data-driven framework is proposed for the simultaneous discovery of Lagrange densities and diffusion coefficients of stochastically excited dynamical systems by leveraging sparse regression. This novel framework offers several advantages over existing approaches. Firstly, it provides an interpretable description of the underlying Lagrange density, allowing for a deeper understanding of system dynamics under stochastic excitations. Secondly, it identifies the interpretable form of the diffusion coefficient of generalized stochastic force, addressing the limitations of existing deterministic approaches. Additionally, the framework demonstrates robustness and versatility through numerical case studies encompassing both stochastic differential equations (SDEs) and stochastic partial differential equations (SPDEs), with results showing almost exact approximations to true system behavior and minimal relative error in derived equations of motion.
更多查看译文
关键词
Lagrangian discovery,Stochastic dynamics,Data-driven framework,Sparse regression,Interpretability
AI 理解论文
溯源树
样例
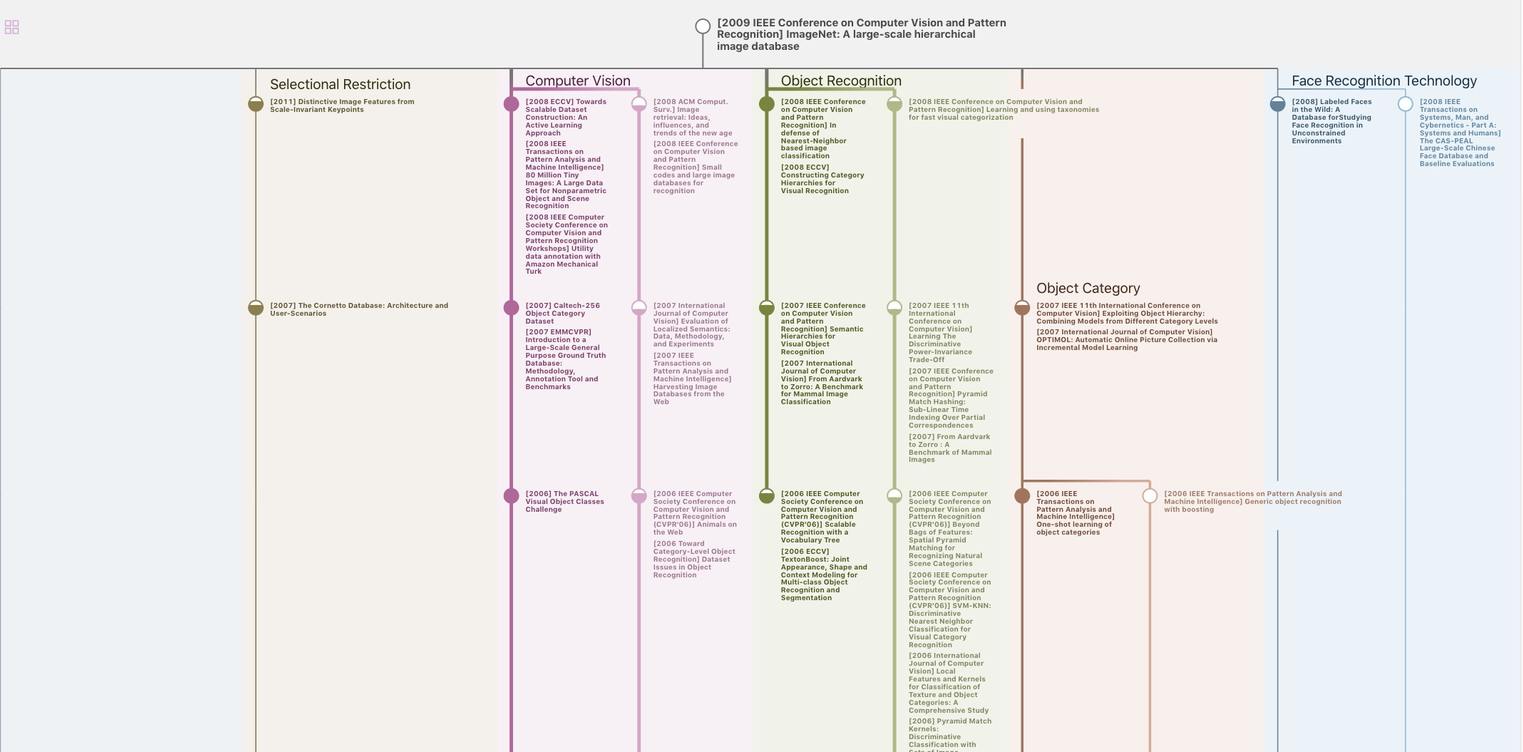
生成溯源树,研究论文发展脉络
Chat Paper
正在生成论文摘要