Unsupervised feature selection using orthogonal encoder-decoder factorization
INFORMATION SCIENCES(2024)
Abstract
Unsupervised feature selection (UFS) is a fundamental task in machine learning and data analysis, aimed at identifying a subset of non -redundant and relevant features from a high -dimensional dataset. Embedded methods seamlessly integrate feature selection into model training, resulting in more efficient and interpretable models. Current embedded UFS methods primarily rely on self -representation or pseudo -supervised feature selection approaches to address redundancy and irrelevant feature issues, respectively. Nevertheless, there is currently a lack of research showcasing the fusion of these two approaches. This paper proposes the Orthogonal EncoderDecoder factorization for unsupervised Feature Selection (OEDFS) model, combining the strengths of self -representation and pseudo -supervised approaches. This method draws inspiration from the self -representation properties of autoencoder architectures and leverages encoder and decoder factorizations to simulate a pseudo -supervised feature selection approach. To further enhance the part -based characteristics of factorization, orthogonality constraints and local structure preservation restrictions are incorporated into the objective function. The optimization process is based on the multiplicative update rule, ensuring efficient convergence. To assess the effectiveness of the proposed method, comprehensive experiments are conducted on 14 datasets and compare the results with eight state-of-the-art methods. The experimental results demonstrate the superior performance of the proposed approach in terms of UFS efficiency.
MoreTranslated text
Key words
Unsupervised feature selection,Encoder-decoder model,Self-representation learning,Pseudo-supervised learning,Nonnegative matrix factorization
AI Read Science
Must-Reading Tree
Example
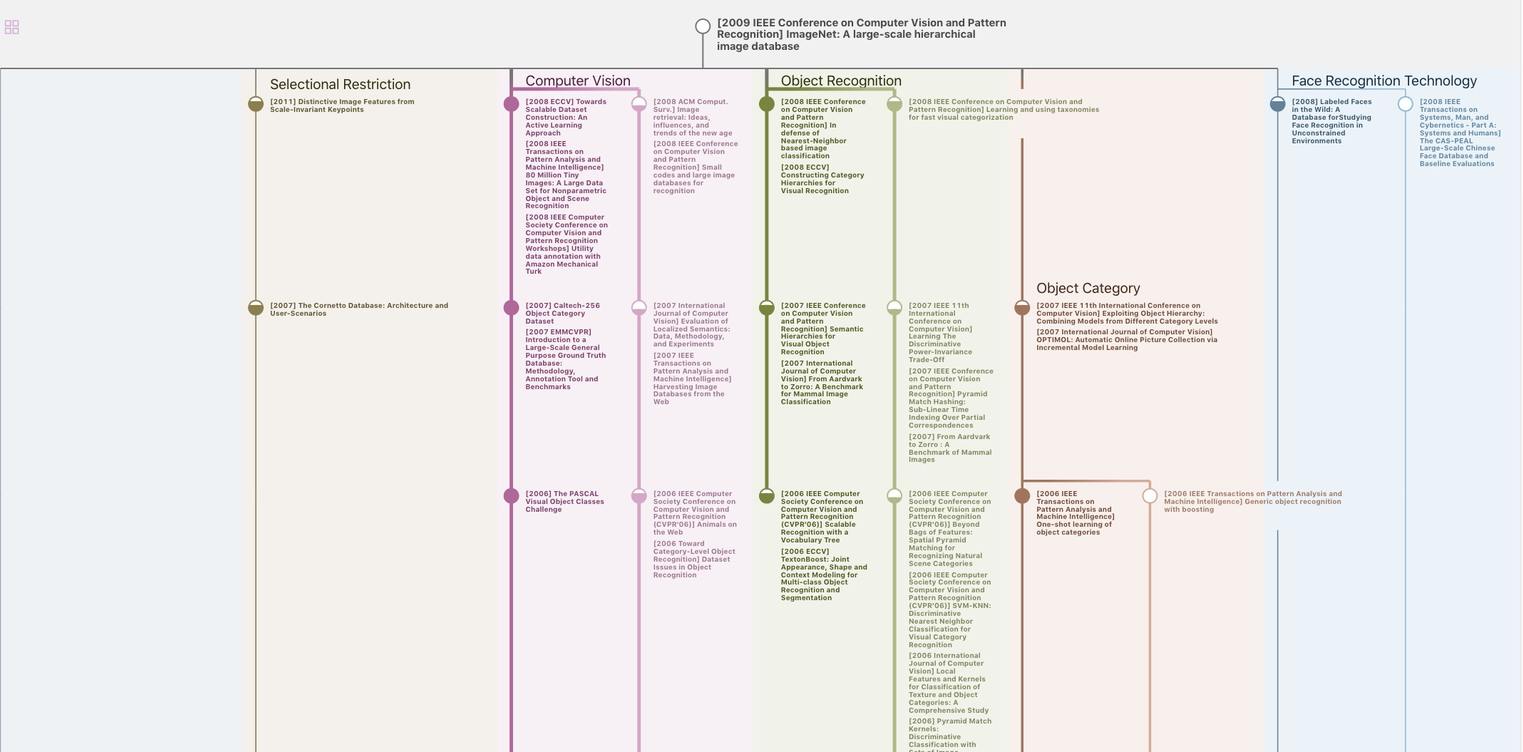
Generate MRT to find the research sequence of this paper
Chat Paper
Summary is being generated by the instructions you defined