Deep Reinforcement Learning-Based Network Slicing Algorithm for 5G Heterogenous Services
IEEE CONFERENCE ON GLOBAL COMMUNICATIONS, GLOBECOM(2023)
摘要
Network slicing is a promising solution to handle the multiple use cases of the 5G system with their diverse requirements. However, applying a static slicing scheme could cause waste of resource and affect the performance. Therefore, dynamic slicing and resource management are necessary to manage the network's resources efficiently among different slices fulfilling their respective requirements. Network slicing approaches proposed in the literature usually consider a small number of slices and do not account for the numerous 5G services simultaneously. In this paper, we study the network slicing problem at the level of the network's computing and storage resources at the edge to support a large number of slices. We model the network and formulate the slicing problem as an integer linear program to maximize the estimated volume of accepted requests of diverse services. We propose a deep reinforcement learning (DRL) based approach to find a near-optimal solution for the NP-hard optimization problem. We assess the performance and the convergence of the proposed algorithm considering multiple configurations. Moreover, we apply the results to a heuristic task offloading that employs network slicing and compare the performance with static slicing. The simulation results show, i) the impact and importance of the parameters during the model's training and building, ii) the proposed algorithm's efficiency in presence of a large number of slices, iii) the importance of dynamic resource management in network slicing.
更多查看译文
关键词
5G Network Slicing,Resource Allocation,5G Services,Deep Reinforcement Learning
AI 理解论文
溯源树
样例
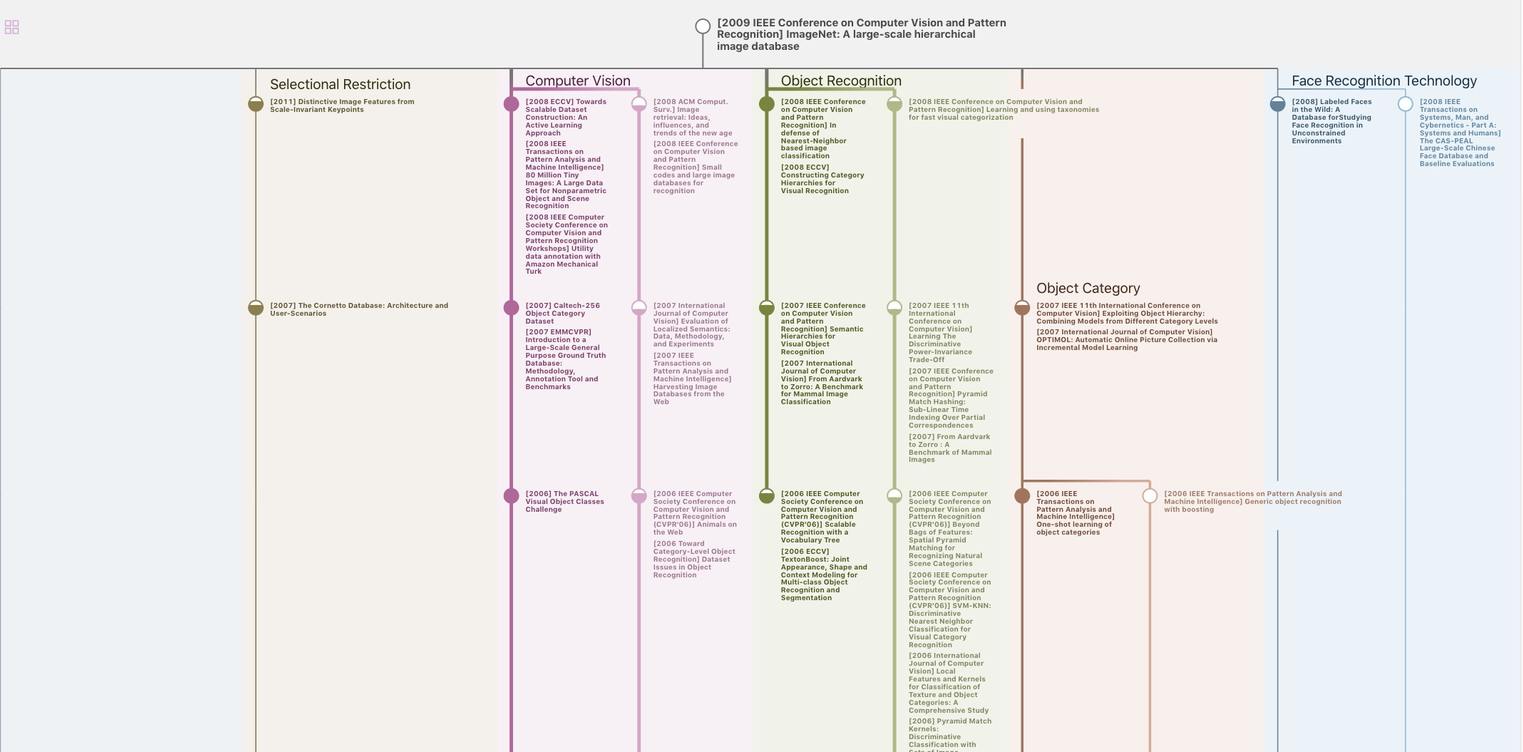
生成溯源树,研究论文发展脉络
Chat Paper
正在生成论文摘要