Knowledge Representation-Actuated Based Spatio-Temporal Graph Neural Network Traffic Flow Prediction
IEEE CONFERENCE ON GLOBAL COMMUNICATIONS, GLOBECOM(2023)
摘要
In the task of traffic flow forecasting, various external factors need to be considered to interfere with the flow, such as weather conditions, traffic accidents, emergency events, and Points of Interest (POIs). While capturing the spatio-temporal dependencies, it is essential to effectively capture the external factors. However, existing studies cannot effectively cascade the information contained in these external factors to traffic features, and lack the co-capture of spatio-temporal features. To address these challenges, we present a Knowledge Representation learning-actuated Spatio-Temporal Graph Neural Network (KR-STGNN) for traffic flow prediction. The Gated Feature Fusion Module (GFFM) is utilized to combine the knowledge embedding with the traffic features, and the traffic features are updated adaptively and dynamically according to the importance of external factors. To conduct the co-capture of spatio-temporal dependencies, we subsequently propose a spatio-temporal feature synchronous capture module combining dilation causal convolution with GRU. Experimental results on a real-world traffic dataset demonstrate that KR-STGNN has superior forecasting performances with different prediction steps, especially for short-term prediction.
更多查看译文
关键词
traffic flow prediction,spatio-temporal graph neural network,knowledge representation learning,external knowledge fusion
AI 理解论文
溯源树
样例
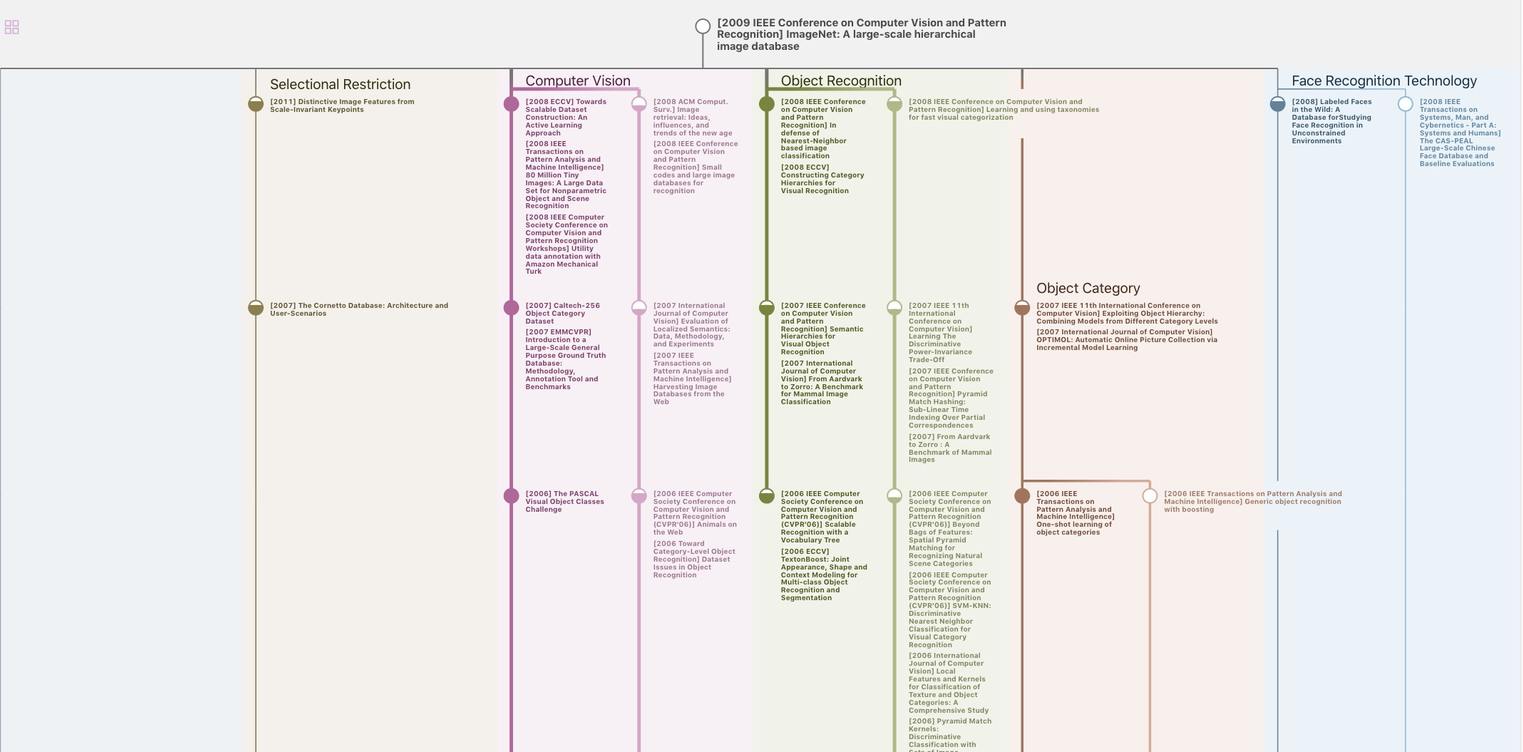
生成溯源树,研究论文发展脉络
Chat Paper
正在生成论文摘要