Task Provisioning in Unreliable Edge Networks: Inferring Utility
IEEE CONFERENCE ON GLOBAL COMMUNICATIONS, GLOBECOM(2023)
摘要
Edge computing can satisfy the requirements of latency-critical and data-intensive applications by exploiting computational resources of end devices. However, such devices inherently suffer from dynamic user behavior, cyclic task-switching, varying link qualities which often impact their reliability. In addition, in incentivized systems, they may often over-estimate their advertised capabilities and consequently fail on delivering. In this paper, we propose the Reputation-based Task Assignment and Replication (RTAR) scheme. RTAR is the first scheme that uses a black box approach to perform cost-efficient task replication that accounts for workers' reliability and preserves workers' privacy by not requiring or soliciting any information about their devices. RTAR incorporates a reputation model using beta distribution to estimate the worker's reputation based on past performance. We formulate the problem as an Integer Linear Program (ILP) that strives to maximize the overall reputation of recruited workers, while abiding by a certain budget limit for each task. We also propose the RTAR-Heuristic (RTAR-H) scheme. RTAR-H uses matching theory to solve the optimization problem in a time-efficient manner. Extensive evaluations show that RTAR yields 63% and 68% reduction in recruitment cost and number of replicas, respectively, compared to a baseline scheme that blindly maximizes the number of replicas. Moreover, RTAR-H closely approaches the optimal solution, rendering a small gap of up to 1% and 1.2% in terms of task drop rate and recruitment cost, respectively.
更多查看译文
关键词
Edge Computing,Resources Provisioning,Task Replication,Matching Theory,Reliability
AI 理解论文
溯源树
样例
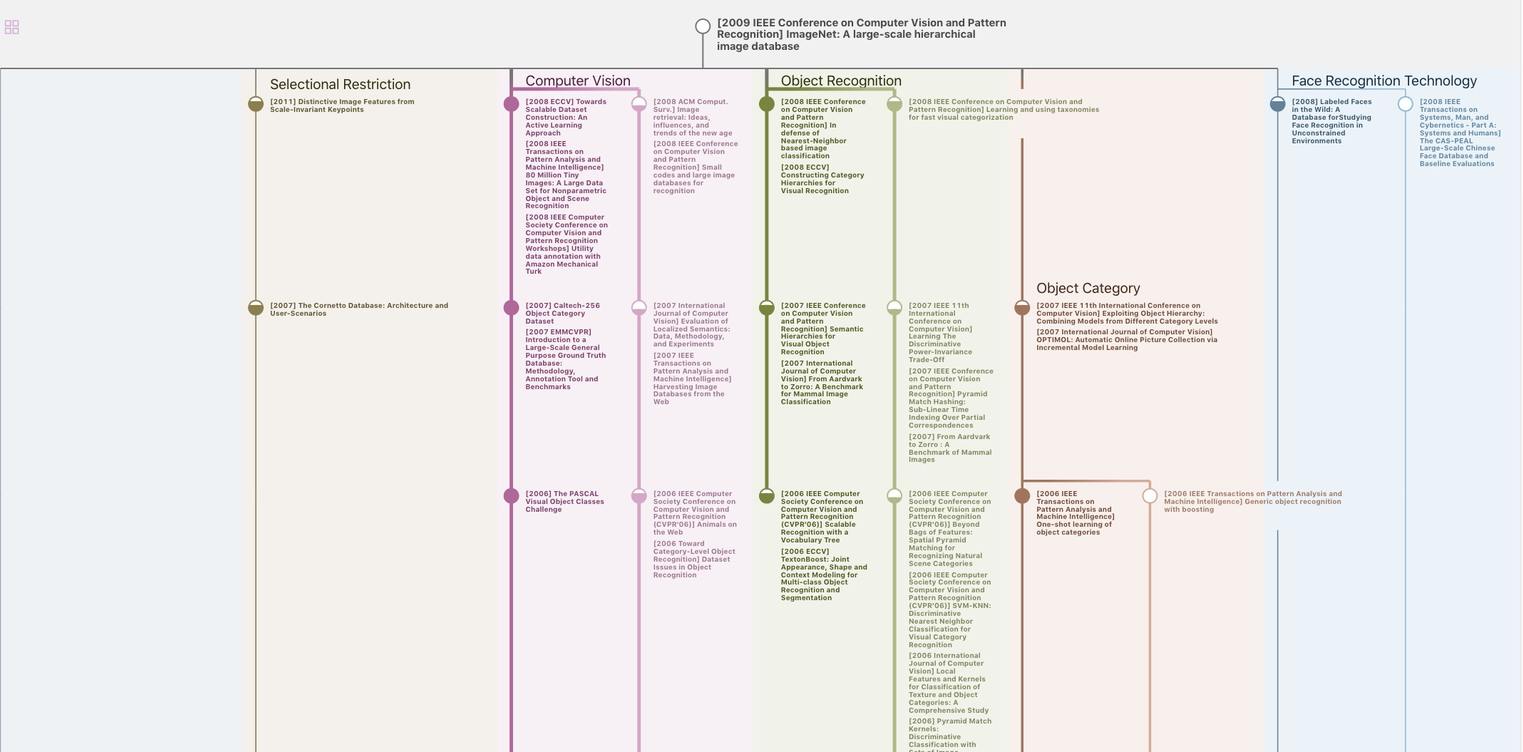
生成溯源树,研究论文发展脉络
Chat Paper
正在生成论文摘要