Machine Learning for Estimating the Impact of Adding New Mobile Network Cells
IEEE CONFERENCE ON GLOBAL COMMUNICATIONS, GLOBECOM(2023)
摘要
In the context of densifying the mobile network in urbanized areas, it is a common practice to increase the capacity by updating existing cell sectors. This paper studies upgrades consisting in adding new cellular frequencies on existing sectors. Using historical data aggregated at sector level spanning over 18 months, we proposed methods to i) following a sector upgrade, evaluate the trending growth rates of resource availability and of the proportion of resource usage per user of already existing cells, ii) use machine learning to predict this trend given the sector existing frequencies, planned frequencies to be added, previous performance indicators and urban fabrics, iii) prioritize cell deployments by computing a predictive score based on the previous predictions. To evaluate the efficiency of machine learning methods, gradient boosting, random forest and SVM models were compared with a baseline, using several metrics. The baseline model was designed to predict the traffic activity evolution as the mean evolution of the same added and existing configurations seen in the training set. This baseline was beaten by all the models trained with our methods. The ranking made by the best ML models had an average precision higher than the baseline by 0.19, a reciprocal rank higher by 0.11 and normalized discounted cumulative gain higher by 0.12.
更多查看译文
关键词
machine learning,mobile network,cell sectors,planning,urban fabrics
AI 理解论文
溯源树
样例
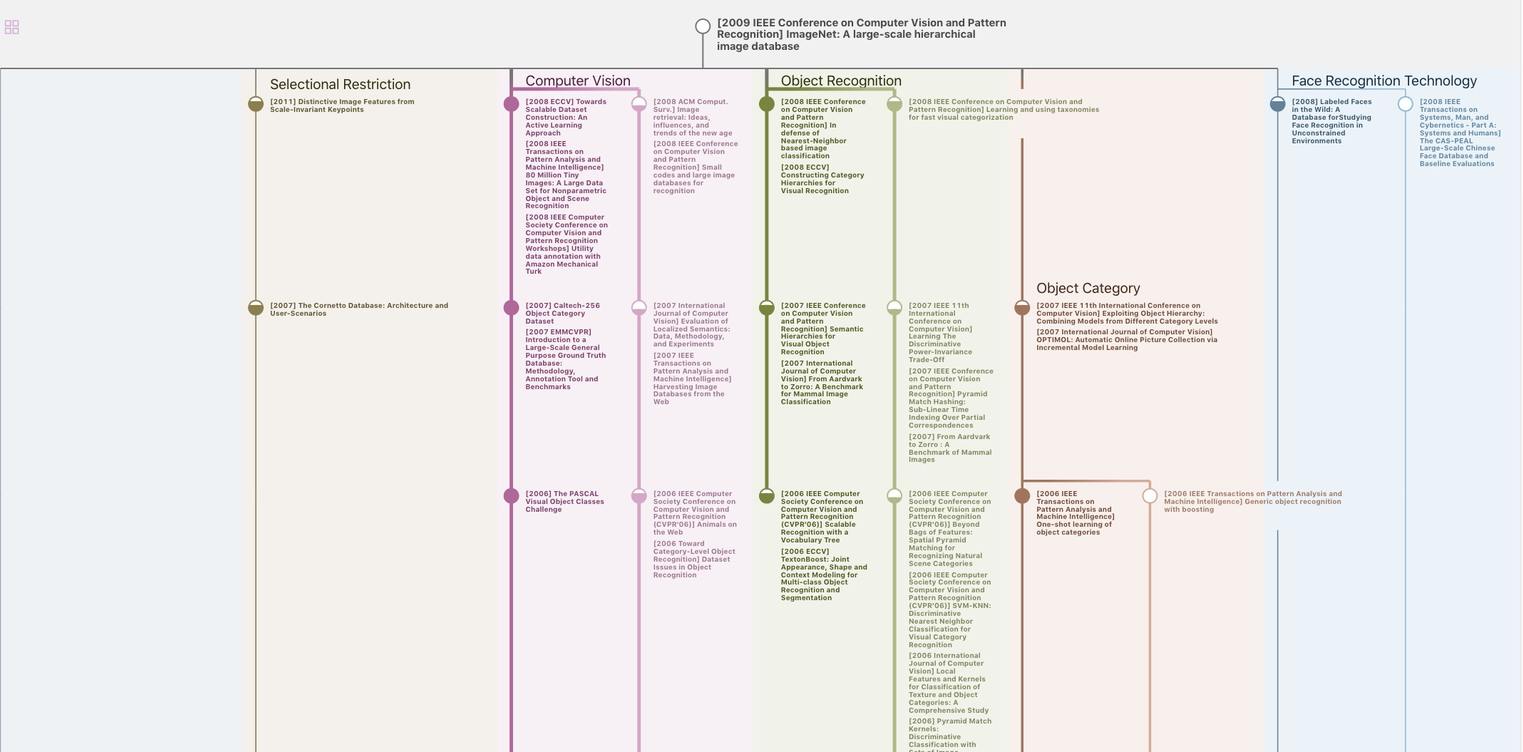
生成溯源树,研究论文发展脉络
Chat Paper
正在生成论文摘要