MIMO Detection Using Gradient-Based Markov Chain Monte Carlo Methods.
Global Communications Conference(2023)
摘要
Optimal detection of symbols transmitted over multiple-input multiple-output (MIMO) wireless channels is known to entail exponentially increasing complexity with MIMO dimensions, making it impractical for large-scale systems. Recently, Markov chain Monte Carlo (MCMC) has been combined with the gradient descent (GD) method to create a promising machine learning solution to this issue. This paper proposes a novel algorithm for approaching optimal detection via MCMC random walk accelerated by Nesterov's gradient method, efficiently exploring the discrete search space for MIMO detection. Our proposed method utilizes multiple GDs per random walk and guarantees high sampling efficiency while mitigating the complexity associated with matrix inversions. Simulation results and complexity analysis reveal that the proposed method achieves near-optimal performance and scales effectively to large MIMO dimensions.
更多查看译文
关键词
Multiple-input Multiple-output,Gradient-based Methods,Multiple-input Multiple-output Detection,Machine Learning,Gradient Descent,Invertible,Markov Chain Monte Carlo,Search Space,Random Walk,Gradient Method,Discrete Space,Gradient Descent Method,Optimal Detection,Near-optimal Performance,Posterior Probability,Newton Method,Bit Error Rate,Minimum Mean Square Error,Maximum A Posteriori,Cholesky Decomposition,Bit Error Rate Performance,Multiple-input Multiple-output Systems,Iterative Gradient Descent,Quadrature Amplitude Modulation,Complex Multiplication,Momentum Factor,Iterative Sampling,Rayleigh Fading Channel,Gaussian Random Vector,Previous Update
AI 理解论文
溯源树
样例
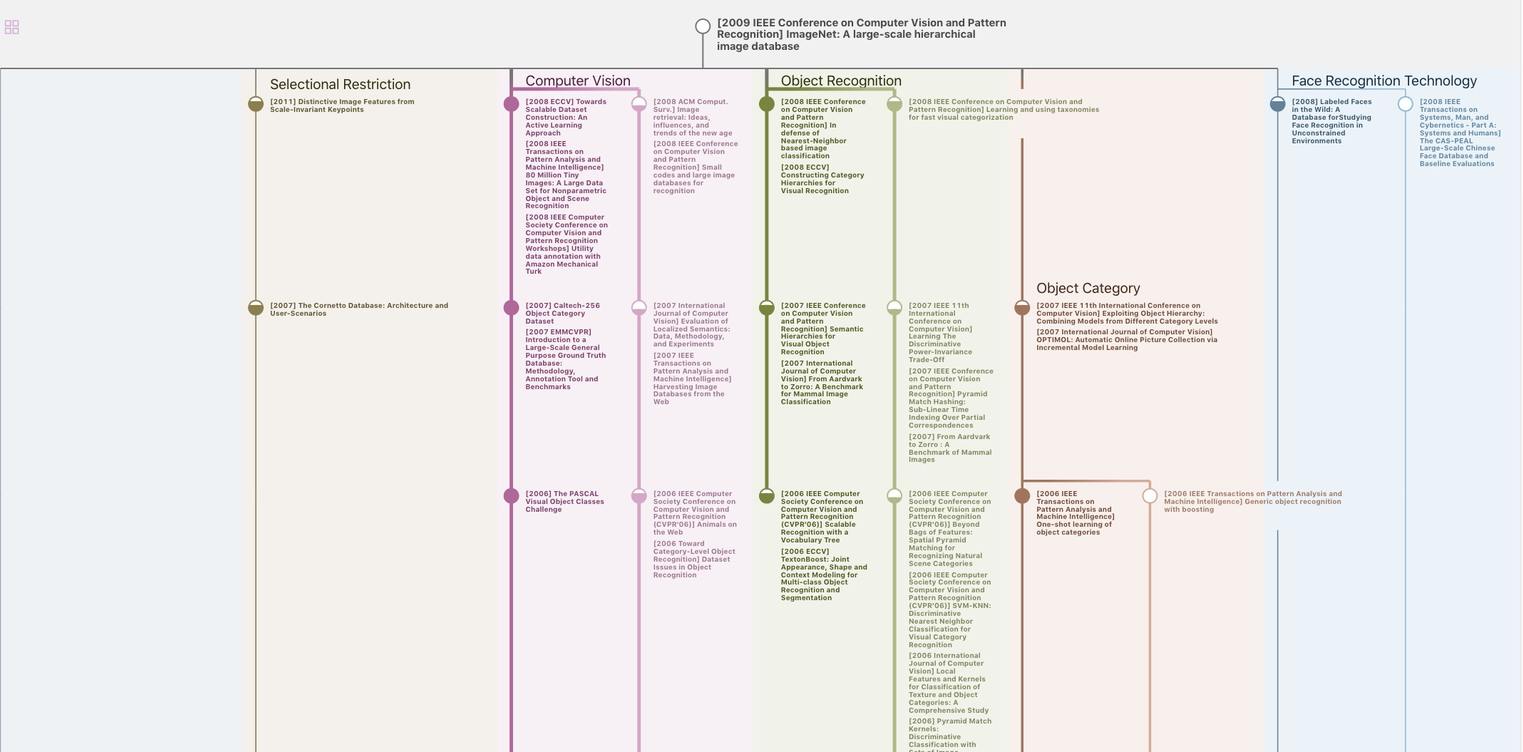
生成溯源树,研究论文发展脉络
Chat Paper
正在生成论文摘要