Dual Layers-Superimposed Training for Joint Channel Estimation and PAPR reduction in OFDM
IEEE CONFERENCE ON GLOBAL COMMUNICATIONS, GLOBECOM(2023)
摘要
Superimposed training (ST) is one of the most appealing channel estimation techniques for orthogonal frequency division multiplexing (OFDM), to be possibly exploited in the 6G. The data and pilot symbols are sharing the same time and frequency resources, and the overhead is significantly reduced. Besides, the superimposed pilots can be also used for the reduction of the peak-to-average power ratio (PAPR). However, joint channel estimation and PAPR reduction procedures have never been addressed. In this work, a novel scheme denoted as dual layers-superimposed training (DL-ST) is proposed for this joint purpose. The training sequence (TS) of the first layer is targeted to perform channel estimation, while the TS of the second layer is designed for PAPR reduction and is made transparent to the first one. Both layers can be independently processed, which implies a reduced complexity. To verify the performance of the proposed technique, the analytical expression of the channel estimation mean squared error (MSE) is derived. Finally, several numerical results further illustrate the performance of the proposal, showing how the MSE is improved while significant PAPR reductions are attained with negligible complexity.
更多查看译文
关键词
channel estimation,layers,OFDM,PAPR,ST
AI 理解论文
溯源树
样例
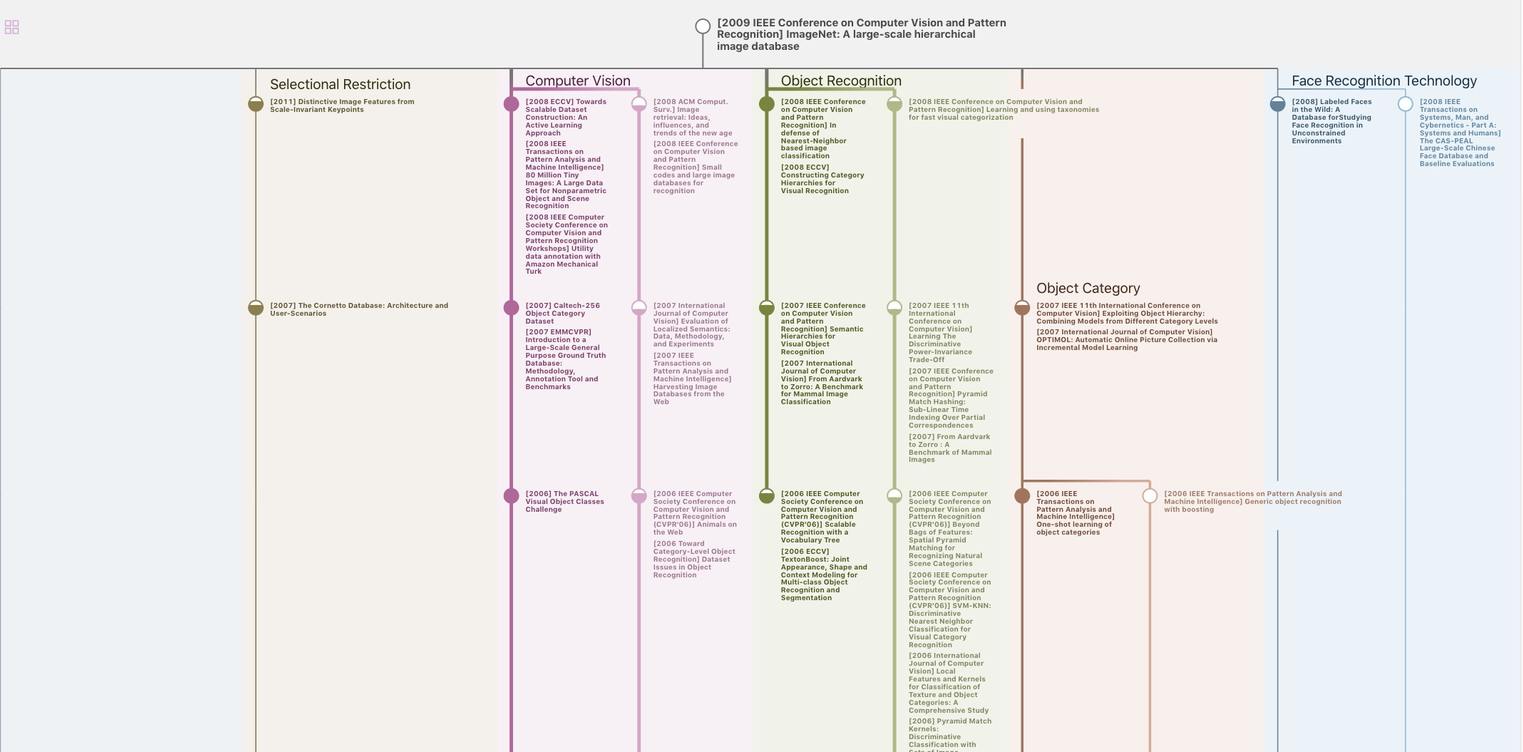
生成溯源树,研究论文发展脉络
Chat Paper
正在生成论文摘要