Machine learning identifies fatigue as a key symptom of fibromyalgia reflected in tyrosine, purine, pyrimidine, and glutaminergic metabolism
CTS-CLINICAL AND TRANSLATIONAL SCIENCE(2024)
摘要
Fibromyalgia patients vary in clinical phenotype and treatment can be challenging. The pathophysiology of fibromyalgia is incompletely understood but appears to involve metabolic changes at rest or in response to stress. We enrolled 54 fibromyalgia patients and 31 healthy controls to this prospective study. Symptoms were assessed using the Fibromyalgia Impact Questionnaire (FIQ) and blood samples were collected for metabolomics analysis at baseline and after an oral glucose tolerance test and a cardiopulmonary exercise test. We identified key symptoms of fibromyalgia and related them to changes in metabolic pathways with supervised and unsupervised machine learning methods. Algorithms trained with the FIQ information assigned the fibromyalgia diagnosis in new data with balanced accuracy of 88% while fatigue alone already provided the diagnosis with 86% accuracy. Supervised analyses reduced the metabolomic information from 77 to 13 key markers. With these metabolites, fibromyalgia could be identified in new cases with 79% accuracy. In addition, 5-hydroxyindole-3-acetic acid and glutamine levels correlated with the severity of fatigue. Patients differed from controls at baseline in tyrosine and purine pathways, and in the pyrimidine pathway after the stress challenges. Several key markers are involved in glutaminergic neurotransmission. This data-driven analysis highlights fatigue as a key symptom of fibromyalgia. Fibromyalgia is associated with metabolic changes which also reflect the degree of fatigue. Responses to metabolic and physical stresses result in a metabolic pattern that allows discrimination of fibromyalgia patients from controls and narrows the focus on key pathophysiological processes in fibromyalgia as treatment targets.
更多查看译文
AI 理解论文
溯源树
样例
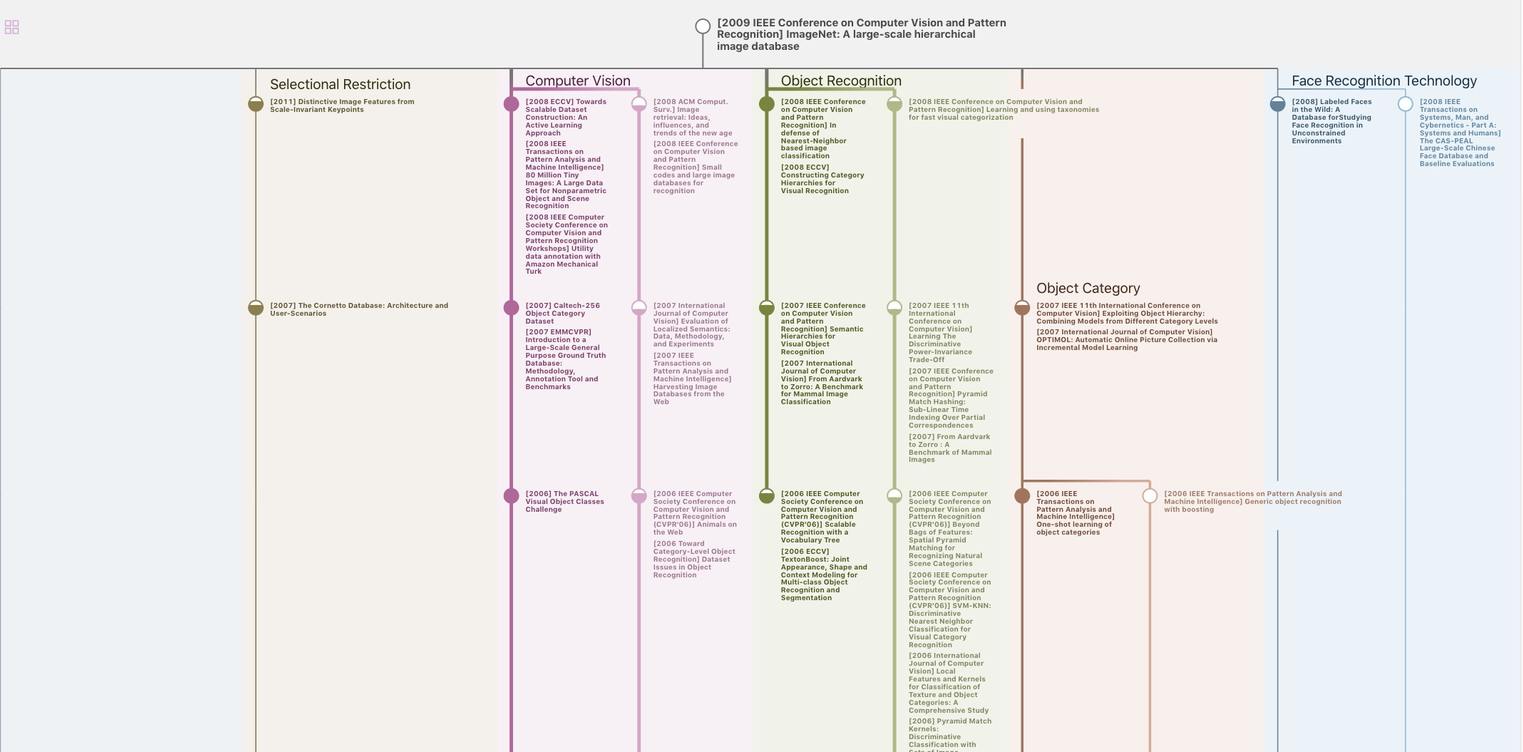
生成溯源树,研究论文发展脉络
Chat Paper
正在生成论文摘要