Syntax-guided question generation using prompt learning
Neural Computing and Applications(2024)
摘要
Question generation (QG) aims to generate natural questions from relevant input. Existing state-of-the-art QG approaches primarily leverage pre-trained language models (PLMs) to encode the deep semantics within the input. Meanwhile, studies show that the input’s dependency parse tree (referred to as syntactic information) is promising in improving NLP-oriented tasks. However, how to incorporate syntactic information in PLMs to guide a QG process effectively still needs to be settled. This paper introduces a syntax-guided sentence-level QG model based on prompt learning. Specifically, we model the syntactic information by utilizing soft prompt learning, jointly considering the syntactic information from a constructed dependency parse graph and PLM to guide question generation. We conduct experiments on two benchmark datasets, SQuAD1.1 and MS MARCO. Experiment results show that our model exceeded both automatic and human evaluation metrics compared with mainstream approaches. Moreover, our case study shows that the model can generate more fluent questions with richer information.
更多查看译文
关键词
Question generation,Pre-trained language models,Syntactic information,Prompt learning
AI 理解论文
溯源树
样例
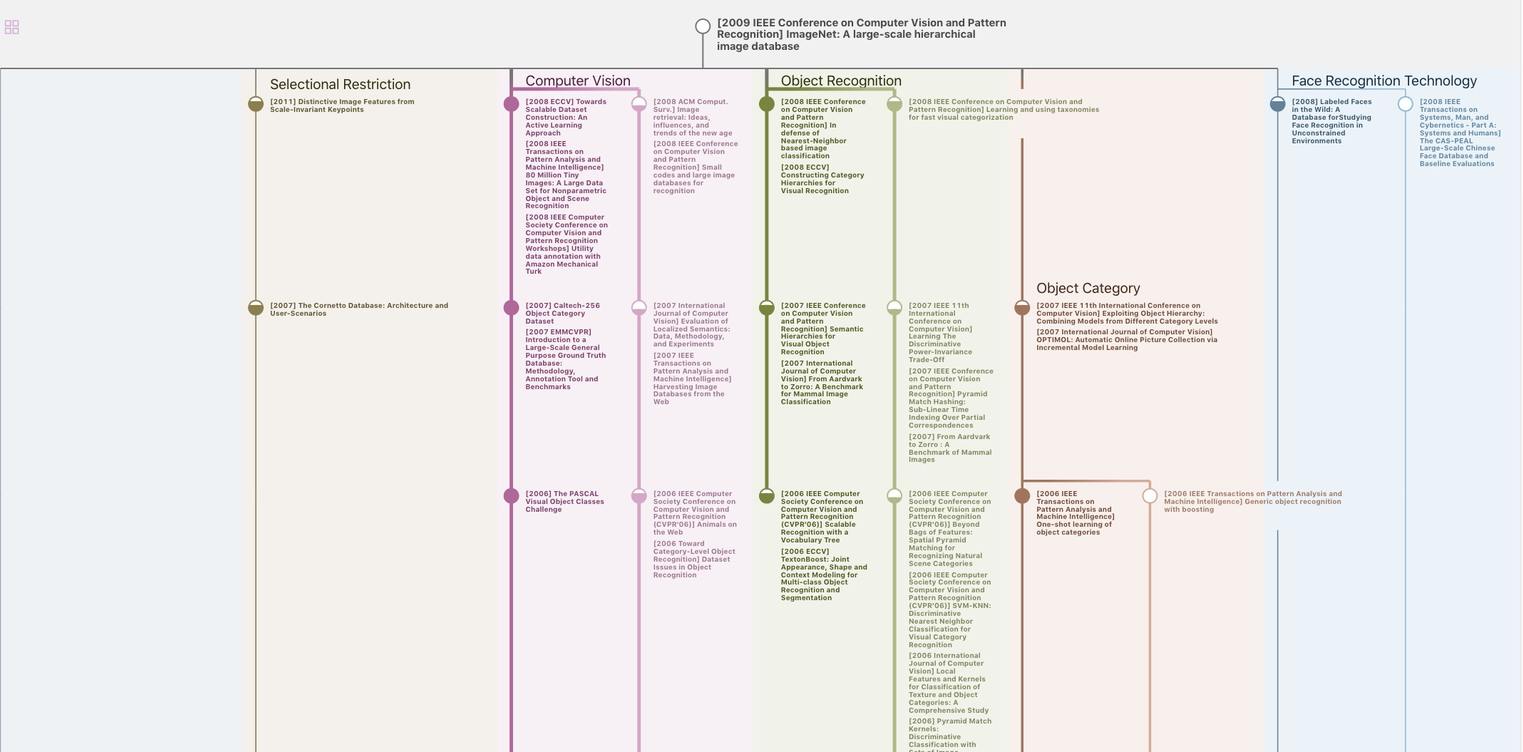
生成溯源树,研究论文发展脉络
Chat Paper
正在生成论文摘要