Cross-Modal Contextualized Diffusion Models for Text-Guided Visual Generation and Editing
ICLR 2024(2024)
摘要
Conditional diffusion models have exhibited superior performance in
high-fidelity text-guided visual generation and editing. Nevertheless,
prevailing text-guided visual diffusion models primarily focus on incorporating
text-visual relationships exclusively into the reverse process, often
disregarding their relevance in the forward process. This inconsistency between
forward and reverse processes may limit the precise conveyance of textual
semantics in visual synthesis results. To address this issue, we propose a
novel and general contextualized diffusion model (ContextDiff) by incorporating
the cross-modal context encompassing interactions and alignments between text
condition and visual sample into forward and reverse processes. We propagate
this context to all timesteps in the two processes to adapt their trajectories,
thereby facilitating cross-modal conditional modeling. We generalize our
contextualized diffusion to both DDPMs and DDIMs with theoretical derivations,
and demonstrate the effectiveness of our model in evaluations with two
challenging tasks: text-to-image generation, and text-to-video editing. In each
task, our ContextDiff achieves new state-of-the-art performance, significantly
enhancing the semantic alignment between text condition and generated samples,
as evidenced by quantitative and qualitative evaluations. Our code is available
at https://github.com/YangLing0818/ContextDiff
更多查看译文
关键词
Diffusion Model,Text-to-Image Generation,Text-to-Video Editing
AI 理解论文
溯源树
样例
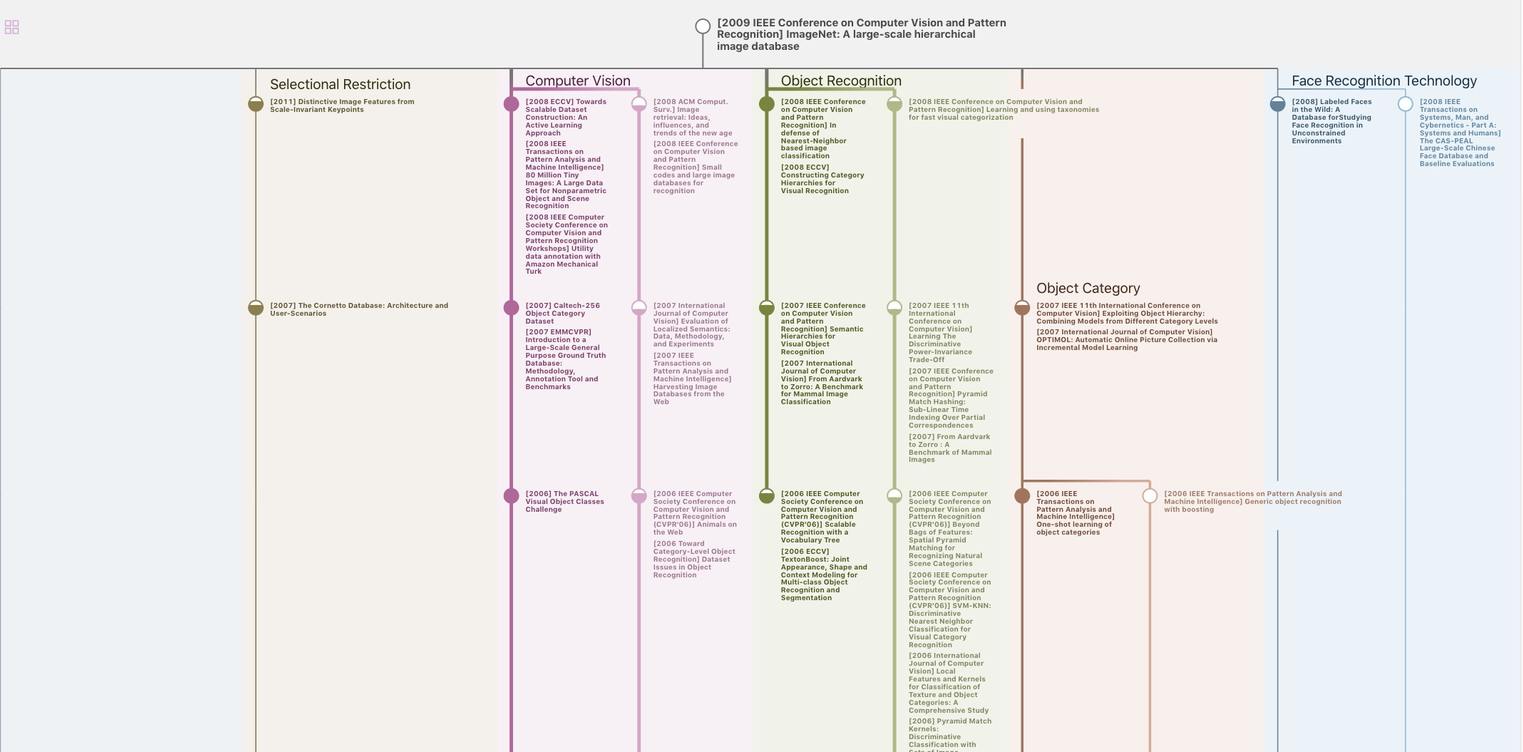
生成溯源树,研究论文发展脉络
Chat Paper
正在生成论文摘要