Matrix denoising: Bayes-optimal estimators via low-degree polynomials
CoRR(2024)
摘要
We consider the additive version of the matrix denoising problem, where a
random symmetric matrix S of size n has to be inferred from the observation
of Y=S+Z, with Z an independent random matrix modeling a noise. For prior
distributions of S and Z that are invariant under conjugation by orthogonal
matrices we determine, using results from first and second order free
probability theory, the Bayes-optimal (in terms of the mean square error)
polynomial estimators of degree at most D, asymptotically in n, and show
that as D increases they converge towards the estimator introduced by Bun,
Allez, Bouchaud and Potters in [IEEE Transactions on Information Theory 62, 7475 (2016)]. We conjecture that this optimality holds beyond strictly
orthogonally invariant priors, and provide partial evidences of this
universality phenomenon when S is an arbitrary Wishart matrix and Z is
drawn from the Gaussian Orthogonal Ensemble, a case motivated by the related
extensive rank matrix factorization problem.
更多查看译文
AI 理解论文
溯源树
样例
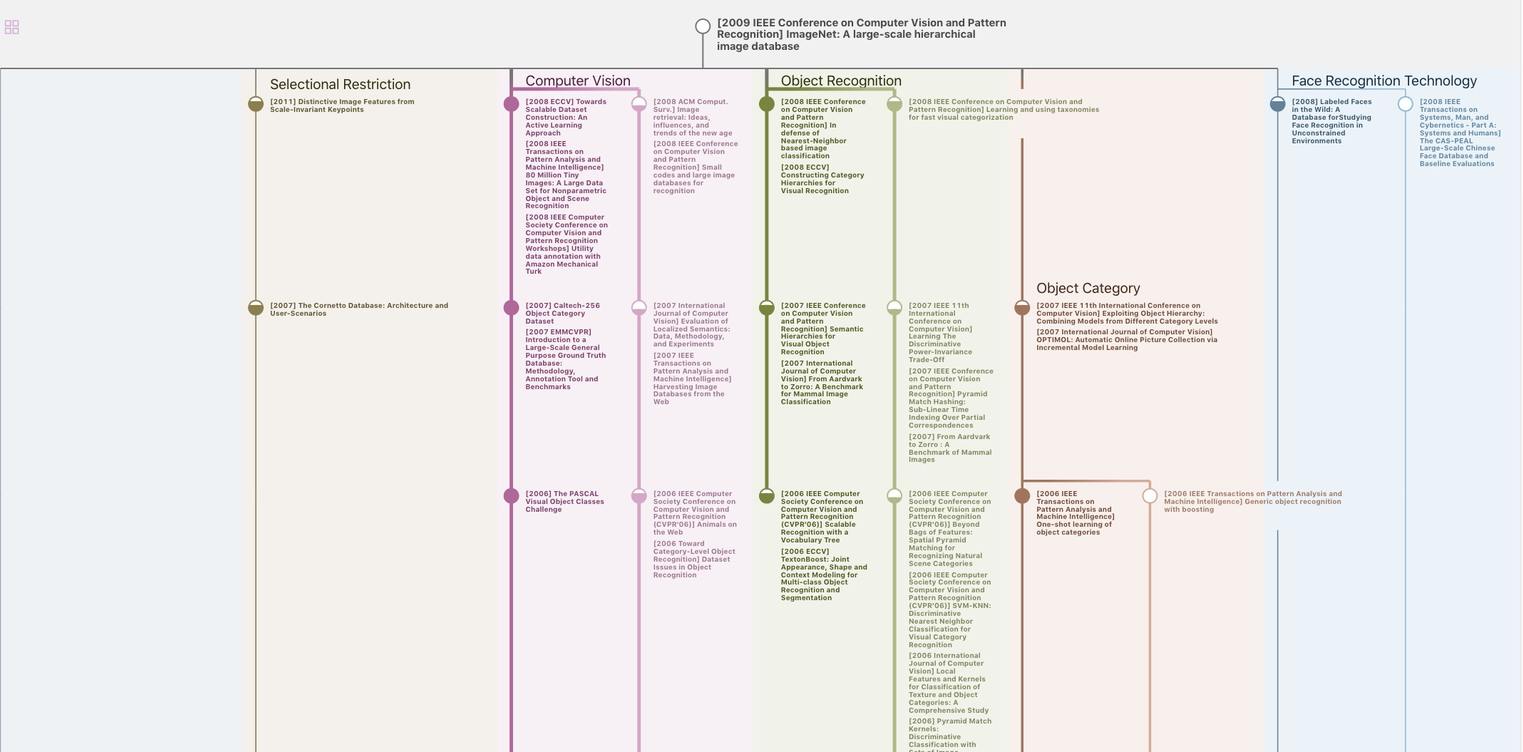
生成溯源树,研究论文发展脉络
Chat Paper
正在生成论文摘要