Model-based deep reinforcement learning for accelerated learning from flow simulations
Meccanica(2024)
摘要
In recent years, deep reinforcement learning has emerged as a technique to solve closed-loop flow control problems. Employing simulation-based environments in reinforcement learning enables a priori end-to-end optimization of the control system, provides a virtual testbed for safety-critical control applications, and allows to gain a deep understanding of the control mechanisms. While reinforcement learning has been applied successfully in a number of rather simple flow control benchmarks, a major bottleneck toward real-world applications is the high computational cost and turnaround time of flow simulations. In this contribution, we demonstrate the benefits of model-based reinforcement learning for flow control applications. Specifically, we optimize the policy by alternating between trajectories sampled from flow simulations and trajectories sampled from an ensemble of environment models. The model-based learning reduces the overall training time by up to 85% for the fluidic pinball test case. Even larger savings are expected for more demanding flow simulations.
更多查看译文
关键词
Closed-loop flow control,Model ensemble proximal policy optimization,Fluidic pinball
AI 理解论文
溯源树
样例
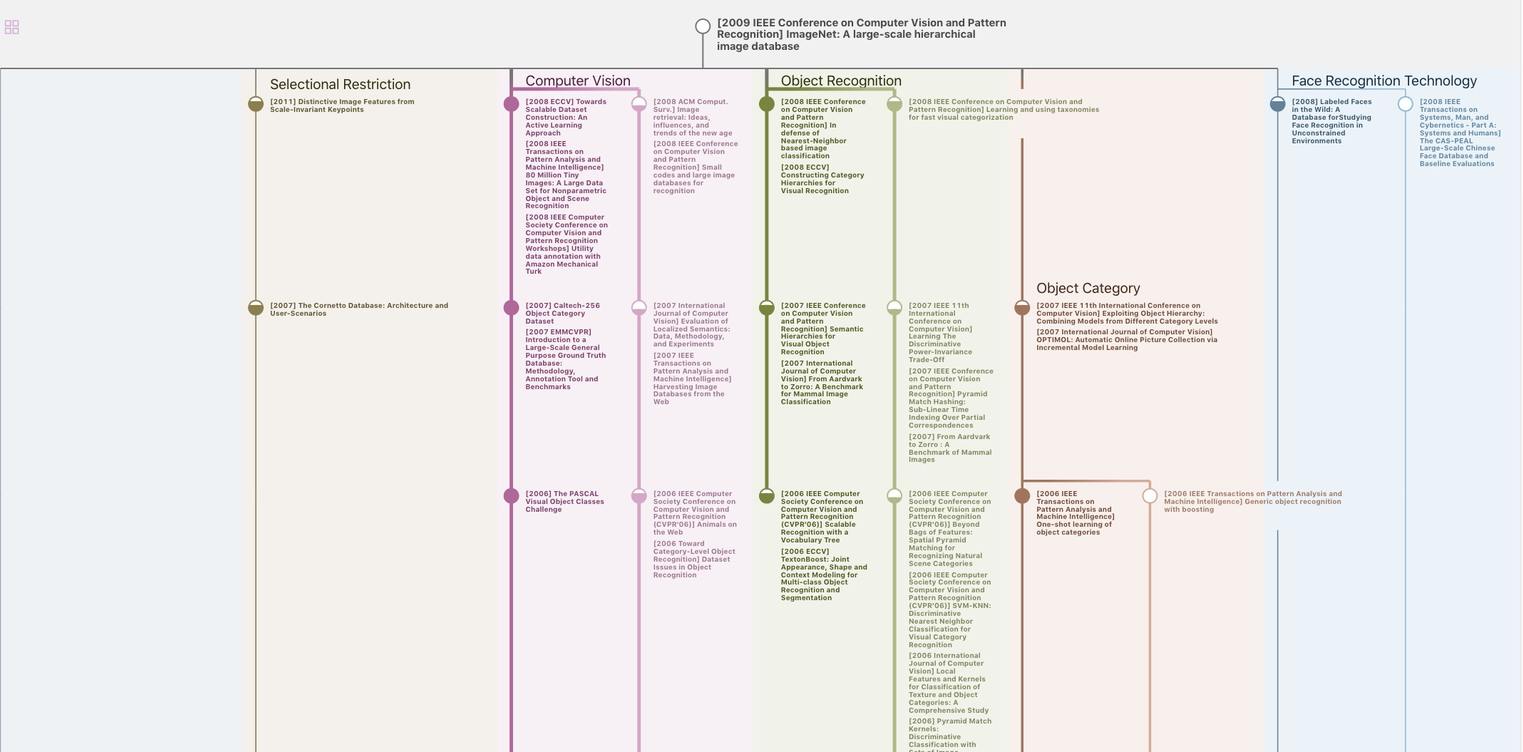
生成溯源树,研究论文发展脉络
Chat Paper
正在生成论文摘要