Fully automated deep learning based auto-contouring of liver segments and spleen on contrast-enhanced CT images
Scientific Reports(2024)
摘要
Manual delineation of liver segments on computed tomography (CT) images for primary/secondary liver cancer (LC) patients is time-intensive and prone to inter/intra-observer variability. Therefore, we developed a deep-learning-based model to auto-contour liver segments and spleen on contrast-enhanced CT (CECT) images. We trained two models using 3d patch-based attention U-Net ( M_paU-Net) and 3d full resolution of nnU-Net ( M_nnU-Net) to determine the best architecture ( BA) . BA was used with vessels ( M_Vess) and spleen ( M_seg+spleen) to assess the impact on segment contouring. Models were trained, validated, and tested on 160 ( C_RTTrain ), 40 ( C_RTVal ), 33 ( C_LS ), 25 (C CH ) and 20 (C PVE ) CECT of LC patients. M_nnU-Net outperformed M_paU-Net across all segments with median differences in Dice similarity coefficients (DSC) ranging 0.03–0.05 (p < 0.05). M_seg+spleen , and M_nnU-Net were not statistically different (p > 0.05), however, both were slightly better than M_Vess by DSC up to 0.02. The final model, M_seg+spleen , showed a mean DSC of 0.89, 0.82, 0.88, 0.87, 0.96, and 0.95 for segments 1, 2, 3, 4, 5–8, and spleen, respectively on entire test sets. Qualitatively, more than 85
更多查看译文
AI 理解论文
溯源树
样例
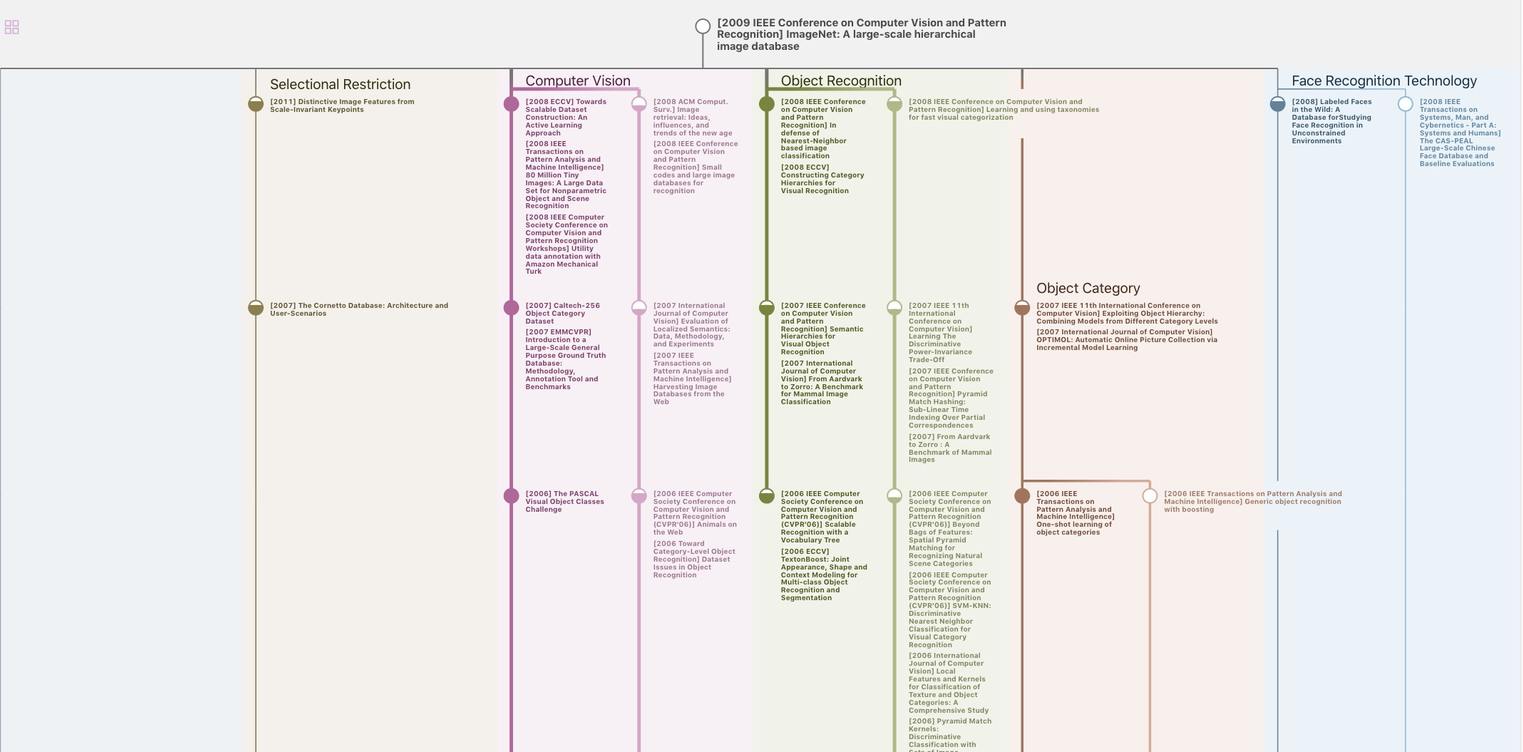
生成溯源树,研究论文发展脉络
Chat Paper
正在生成论文摘要