Top-Personalized-K Recommendation
CoRR(2024)
摘要
The conventional top-K recommendation, which presents the top-K items with
the highest ranking scores, is a common practice for generating personalized
ranking lists. However, is this fixed-size top-K recommendation the optimal
approach for every user's satisfaction? Not necessarily. We point out that
providing fixed-size recommendations without taking into account user utility
can be suboptimal, as it may unavoidably include irrelevant items or limit the
exposure to relevant ones. To address this issue, we introduce
Top-Personalized-K Recommendation, a new recommendation task aimed at
generating a personalized-sized ranking list to maximize individual user
satisfaction. As a solution to the proposed task, we develop a model-agnostic
framework named PerK. PerK estimates the expected user utility by leveraging
calibrated interaction probabilities, subsequently selecting the recommendation
size that maximizes this expected utility. Through extensive experiments on
real-world datasets, we demonstrate the superiority of PerK in
Top-Personalized-K recommendation task. We expect that Top-Personalized-K
recommendation has the potential to offer enhanced solutions for various
real-world recommendation scenarios, based on its great compatibility with
existing models.
更多查看译文
AI 理解论文
溯源树
样例
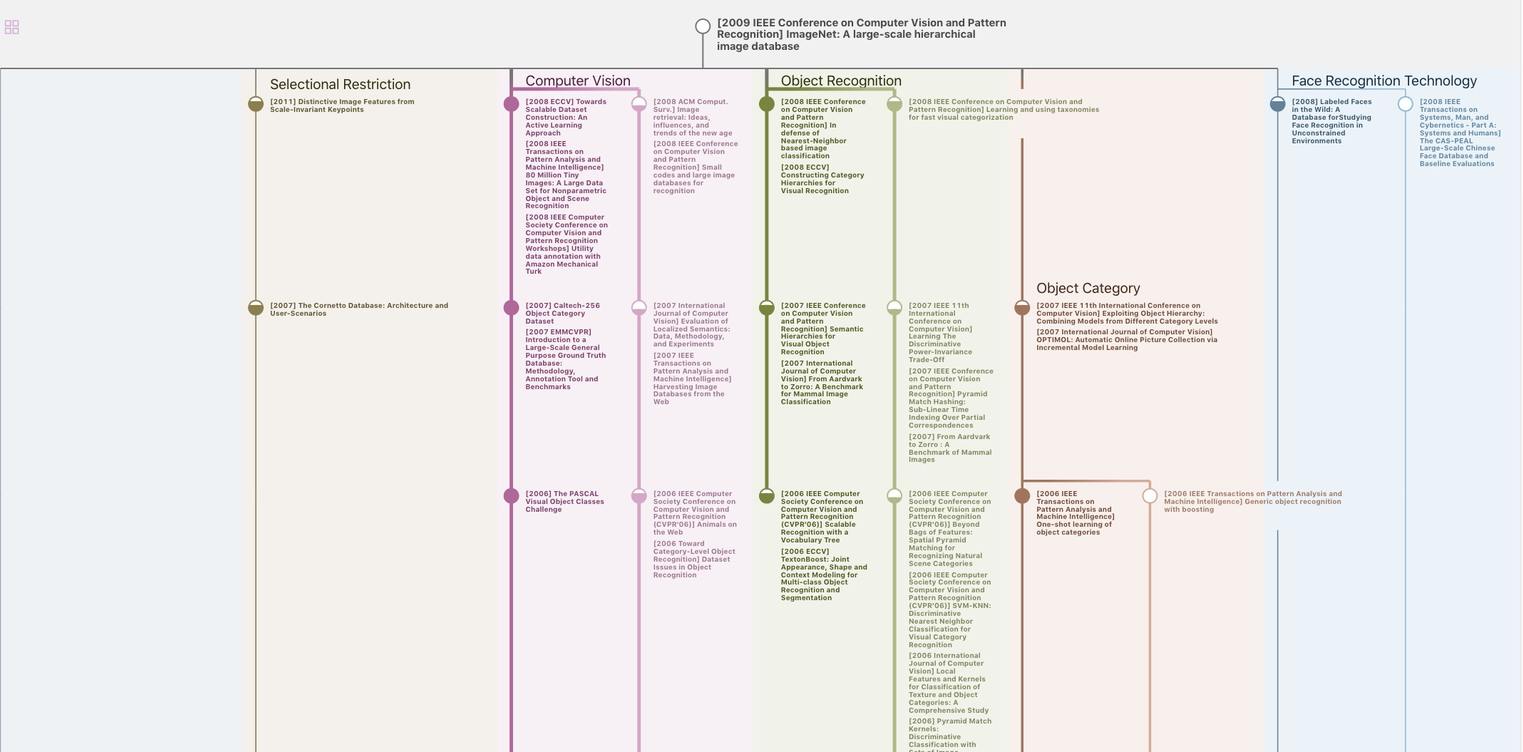
生成溯源树,研究论文发展脉络
Chat Paper
正在生成论文摘要