DeepForge: Leveraging AI for microstructural control in metal forming via model predictive control
Journal of Manufacturing Processes(2024)
摘要
This study presents a novel method for microstructure control in closed die hot forging that combines Model Predictive Control (MPC) with a developed machine learning model called DeepForge. DeepForge uses an architecture that incorporates 1D convolutional neural networks and gated recurrent units to make predictions about microstructure changes during forging based on the surface temperature of a workpiece. The paper also details DeepForge’s architecture and the finite element simulation of a three-stroke forging process used to generate the dataset for AI model development. The results demonstrate DeepForge’s ability to replace the underlying FE simulation and predict microstructure with a mean absolute error of 0.4 ± 0.3%. In addition, the study explores the use of MPC to adjust inter-stroke wait times, effectively counteracting temperature disturbances to achieve a target grain size of less than 35μm within a specific 2D region of the workpiece. These results are then verified experimentally, demonstrating a significant step towards improved control and quality in forging processes where temperature can be used as an additional degree of freedom in the process.
更多查看译文
关键词
Hot closed-die forging,Model predictive control,Machine learning,Microstructure prediction,Finite element method
AI 理解论文
溯源树
样例
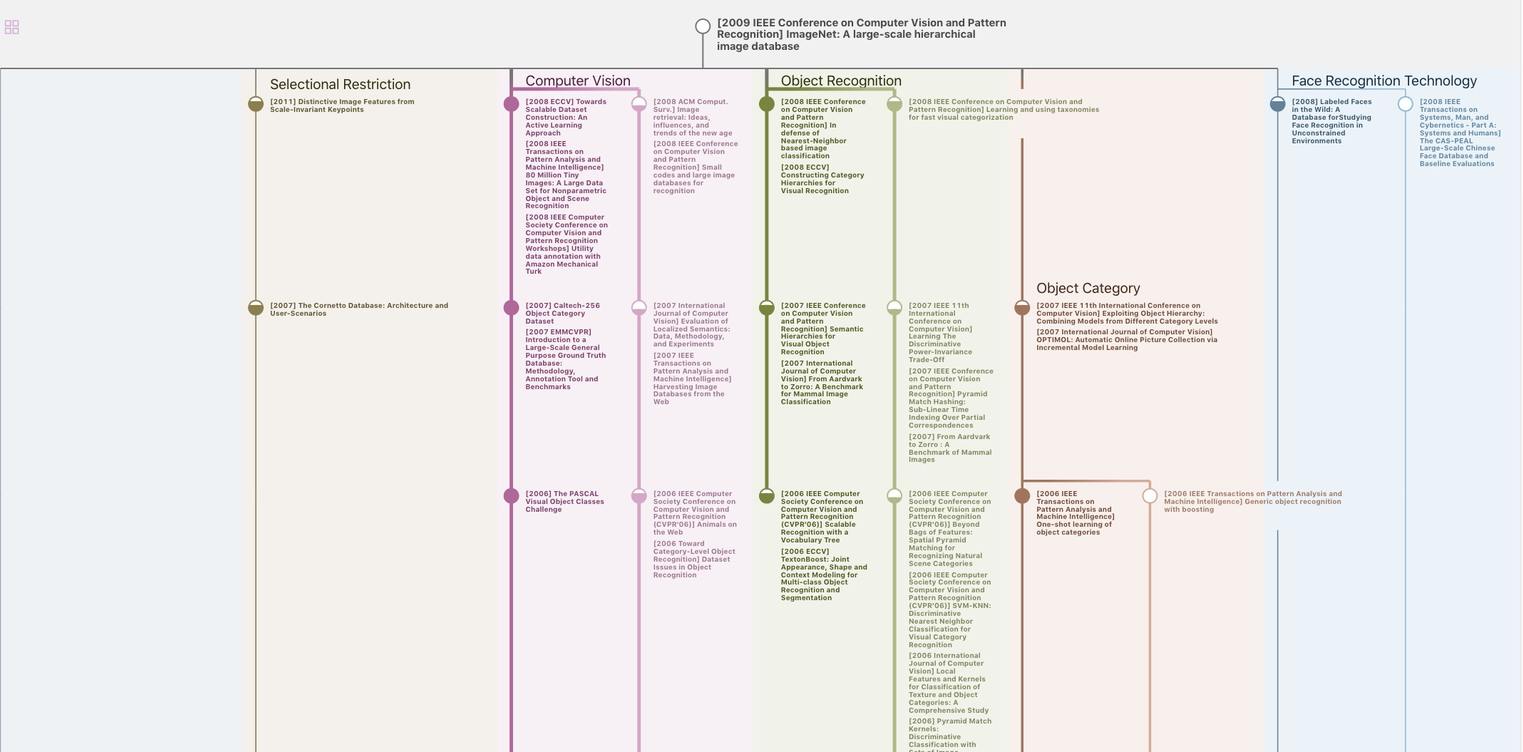
生成溯源树,研究论文发展脉络
Chat Paper
正在生成论文摘要