A unified Fourier slice method to derive ridgelet transform for a variety of depth-2 neural networks
Journal of Statistical Planning and Inference(2024)
摘要
To investigate neural network parameters, it is easier to study the distribution of parameters than to study the parameters in each neuron. The ridgelet transform is a pseudo-inverse operator that maps a given function f to the parameter distribution γ so that a network NN[γ] reproduces f, i.e. NN[γ]=f. For depth-2 fully-connected networks on a Euclidean space, the ridgelet transform has been discovered up to the closed-form expression, thus we could describe how the parameters are distributed. However, for a variety of modern neural network architectures, the closed-form expression has not been known. In this paper, we explain a systematic method using Fourier expressions to derive ridgelet transforms for a variety of modern networks such as networks on finite fields Fp, group convolutional networks on abstract Hilbert space H, fully-connected networks on noncompact symmetric spaces G/K, and pooling layers, or the d-plane ridgelet transform.
更多查看译文
关键词
Neural network,d-plane ridgelet transform,Fourier slice theorem,Group convolution,Noncompact symmetric space
AI 理解论文
溯源树
样例
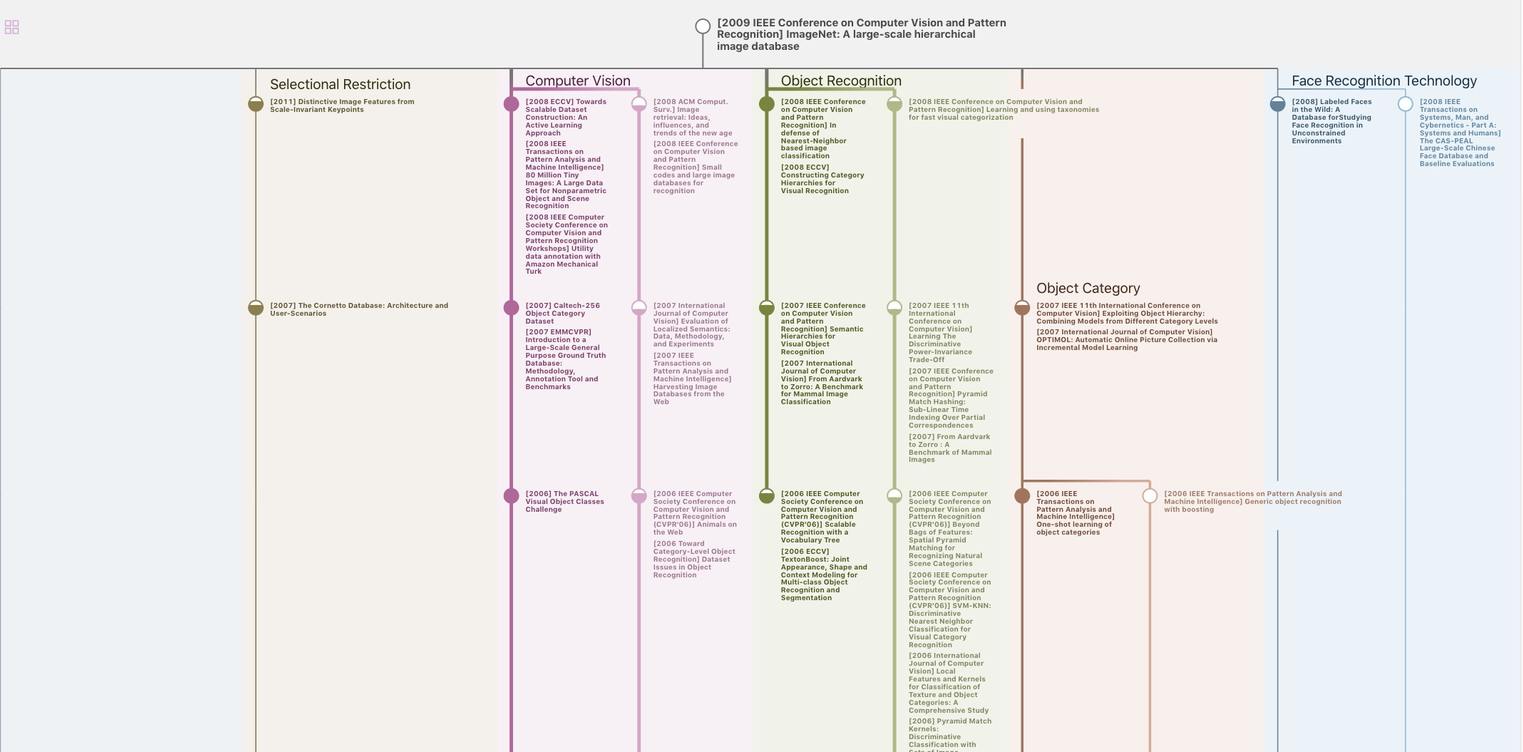
生成溯源树,研究论文发展脉络
Chat Paper
正在生成论文摘要