Importance Guided Data Augmentation for Neural-Based Code Understanding
CoRR(2024)
摘要
Pre-trained code models lead the era of code intelligence. Many models have
been designed with impressive performance recently. However, one important
problem, data augmentation for code data that automatically helps developers
prepare training data lacks study in the field of code learning. In this paper,
we introduce a general data augmentation framework, GenCode, to enhance the
training of code understanding models. GenCode follows a
generation-and-selection paradigm to prepare useful training codes.
Specifically, it uses code transformation techniques to generate new code
candidates first and then selects important ones as the training data by
importance metrics. To evaluate the effectiveness of GenCode with a general
importance metric – loss value, we conduct experiments on four code
understanding tasks (e.g., code clone detection) and three pre-trained code
models (e.g., CodeT5). Compared to the state-of-the-art (SOTA) code
augmentation method, MixCode, GenCode produces code models with 2.92
accuracy and 4.90
更多查看译文
AI 理解论文
溯源树
样例
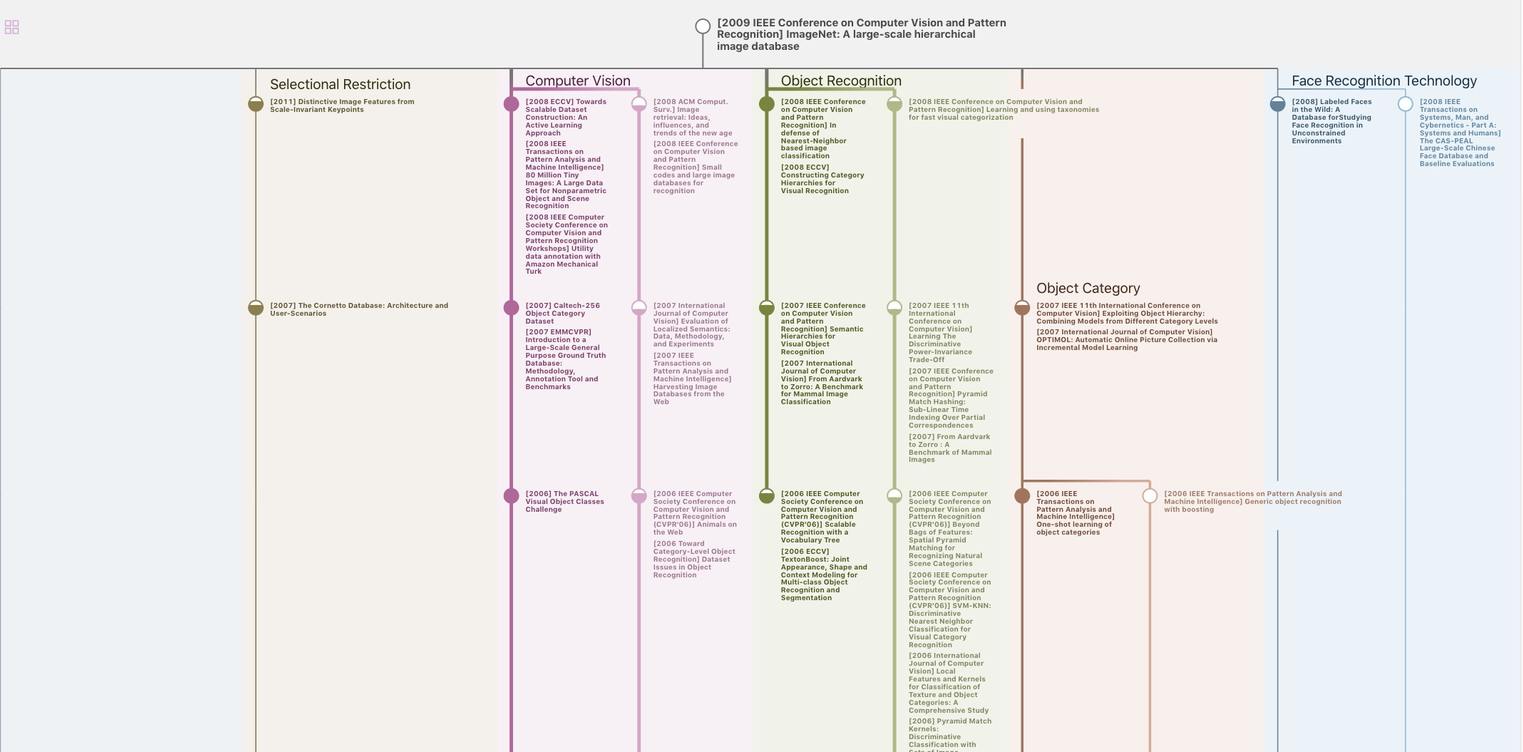
生成溯源树,研究论文发展脉络
Chat Paper
正在生成论文摘要