Stance prediction with a relevance attribute to political issues in comparing the opinions of citizens and city councilors
International Journal on Digital Libraries(2024)
摘要
This study focuses on a method for differentiating between the stance of citizens and city councilors on political issues (i.e., in favor or against) and attempts to compare the arguments of both sides. We created a dataset by annotating citizen tweets and city council minutes with labels for four attributes: stance, usefulness, regional dependence, and relevance. We then fine-tuned pretrained large language model using this dataset to assign the attribute labels to a large quantity of unlabeled data automatically. We introduced multitask learning to train each attribute jointly with relevance to identify the clues by focusing on those sentences that were relevant to the political issues. Our prediction models are based on T5, a large language model suitable for multitask learning. We compared the results from our system with those that used BERT or RoBERTa. Our experimental results showed that the macro-F1-scores for stance were improved by 1.8% for citizen tweets and 1.7% for city council minutes with multitask learning. Using the fine-tuned model to analyze real opinion gaps, we found that although the vaccination regime was positively evaluated by city councilors in Fukuoka city, it was not rated very highly by citizens.
更多查看译文
关键词
Stance prediction,Citizen opinion,City council minutes,Twitter (X),T5,Multitask learning
AI 理解论文
溯源树
样例
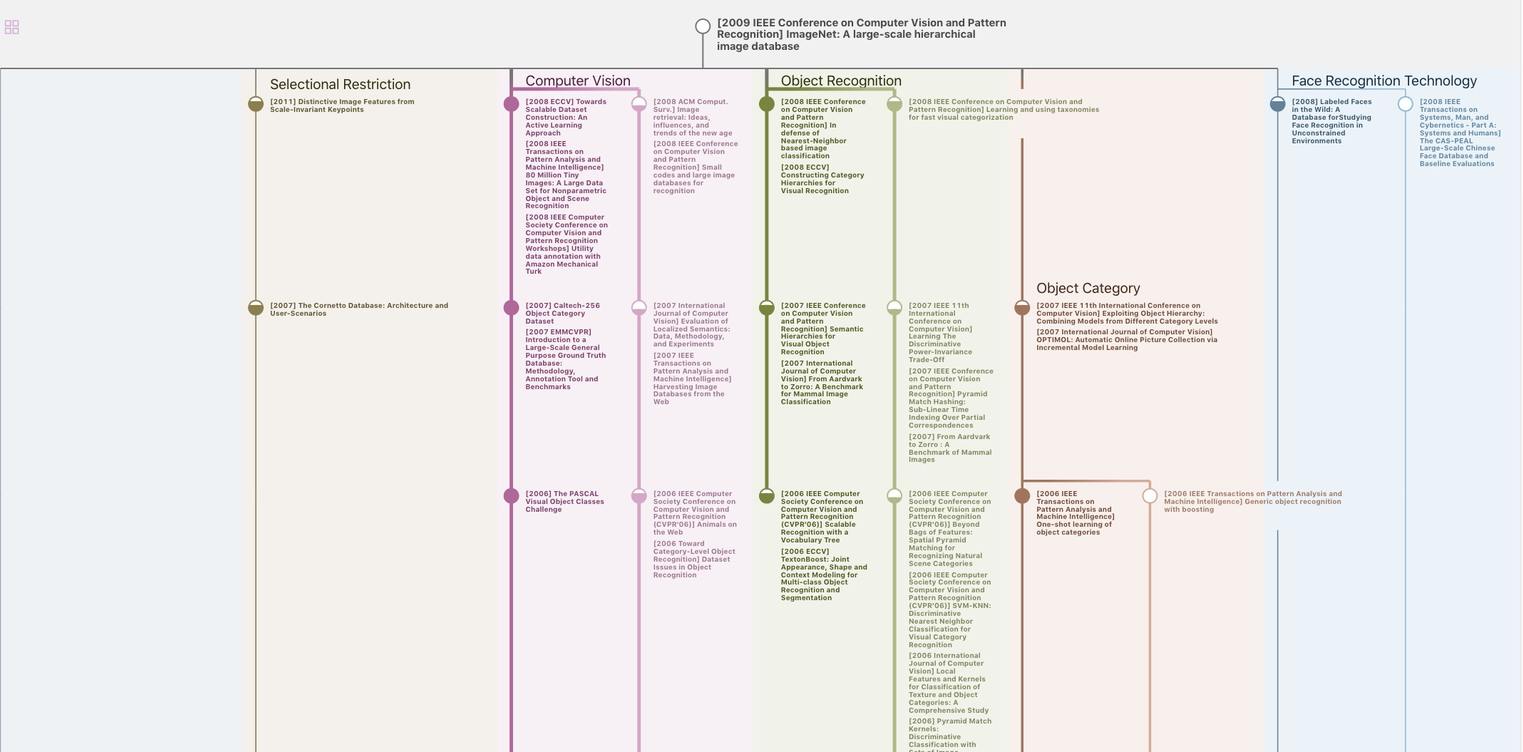
生成溯源树,研究论文发展脉络
Chat Paper
正在生成论文摘要